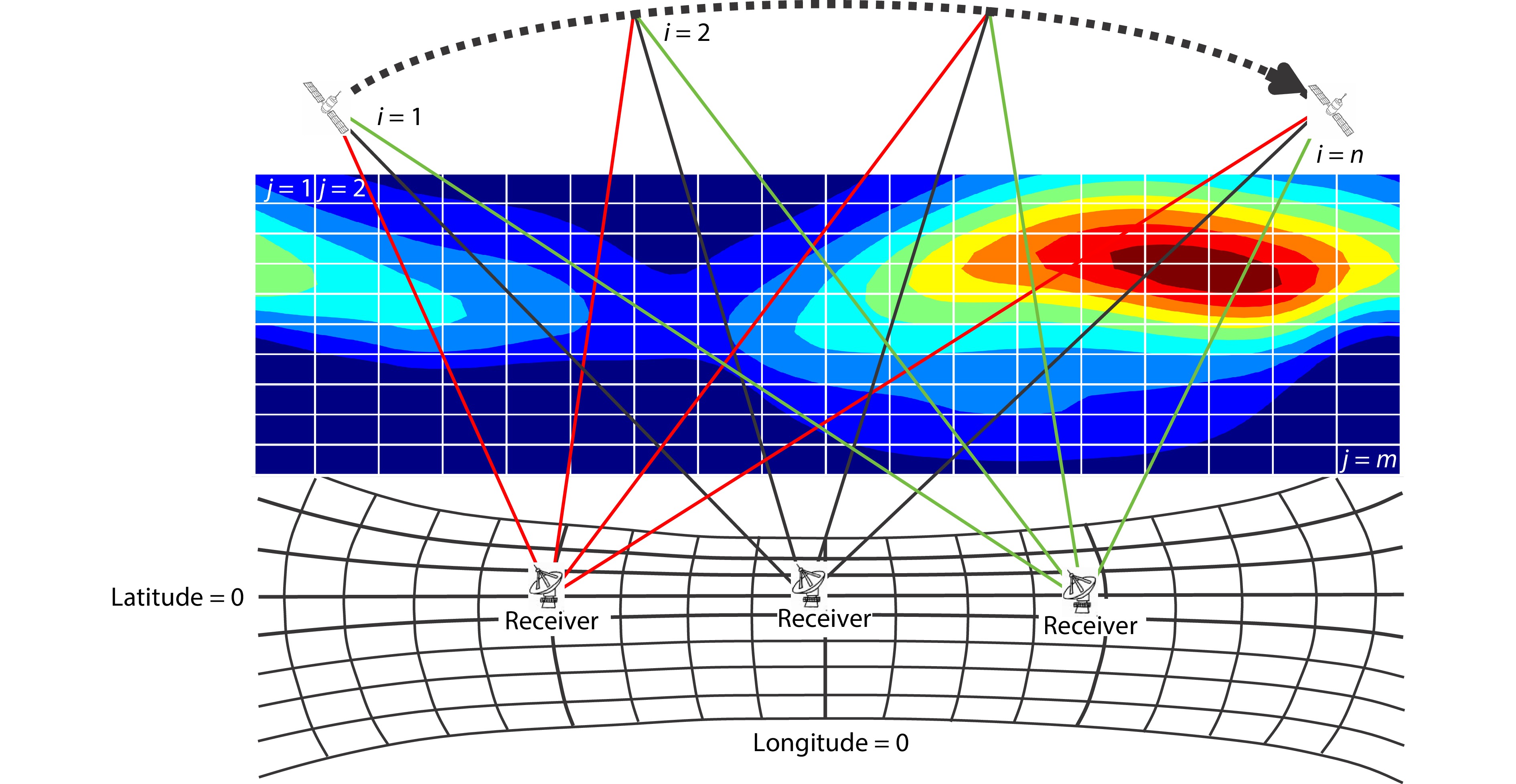
Citation: | Qi, A., and Ruan, H. B. (2025). Accurate quantification of the algebraic, multiplicative algebraic, and simultaneous iterative reconstruction techniques in ionosphere rebuilding based on the TIEGCM assessment. Earth Planet. Phys., 9(1), 117–126. DOI: 10.26464/epp2024060 |
The algebraic reconstruction technique (ART), multiplicative algebraic reconstruction technique (MART), and simultaneous iterative reconstruction technique (SIRT) are computational methodologies extensively utilized within the field of computerized ionospheric tomography (CIT) to facilitate three-dimensional reconstruction of the ionospheric morphology. However, reconstruction accuracy elicits recurrent disputes over its practical application, and people usually attribute this issue to incomplete and uneven coverage of the measurements. The Thermosphere Ionosphere Electrodynamics General Circulation Model (TIEGCM) offers a reasonable physics-based ionospheric background and is widely utilized in ionospheric research. We use the TIEGCM simulations as the targeted ionosphere because the current measurements are far from able to realistically reproduce the ionosphere in detail. Optimized designations of satellite measurements are conducted to investigate the limiting performance of CIT methods in ionospheric reconstruction. Similar to common practice, electron density distributions from outputs of the International Reference Ionosphere (IRI) model are used as the iterative initial value in CIT applications. The outcomes suggest that despite data coverage, iterative initial conditions also play an essential role in ionospheric reconstruction. In particular, in the longitudinal sectors where the iterative initial height of the F2-layer peak electron density (hmF2) differs substantially from the background densities, none of the three CIT methods can reproduce the exact background profile. When hmF2 is close but the ionospheric F2-layer peak density (NmF2) is different between the targeted background and initial conditions, the MART performs better than the ART and SIRT, as evidenced by the correlation coefficients of MART being above 0.97 and those of ART and SIRT being below 0.85. In summary, this investigation reveals the potential uncertainties in traditional CIT reconstruction, particularly when realistic hmF2 or NmF2 values differ substantially from the initial CIT conditions.
Computerized ionospheric tomography (CIT) is widely used for reproducing ionosphere electron density distributions, which are primarily facilitated by advances in the global navigation satellite system (GNSS). Because of insufficient GNSS measurements, the ionospheric reconstructions obtained through tomographic techniques invariably harbor uncertainties, constraining their practical applications, particularly scientific ones. The question is then whether tomography utilizing comprehensive observations would possess the capability to precisely reconstruct the ionosphere. To address this problem, the present study uses the Thermosphere Ionosphere Electrodynamics General Circulation Model (TIEGCM) simulation to construct a physics-based ionospheric background and devises a GNSS–ground network to ensure exhaustive observational coverage. The study uses this methodological framework to evaluate the efficacy of various tomographic techniques for ionospheric reconstruction. The findings reveal that, alongside the uneven spatial distribution of GNSS measurements, the selection of initial iteration values exerts a notable influence on the accuracy of the resultant tomographic reconstructions. These insights carry important implications for advancing the efficacy of ionospheric tomographic methodologies in the future.
The ionosphere ranges from 60 to 1000 km and is filled with charged particles, which affect the radio signals passing through it. Driven nonlinearly by the solar–terrestrial space environment, the ionosphere exhibits complex variabilities (Wan X et al., 2021). Ionospheric monitoring benefits comprehensive fields of communication, navigation, and space weather modeling and forecasting (Xiong C et al., 2016, 2020; Luo XM et al., 2018). Among multiple methodologies, computerized ionospheric tomography (CIT) is used to reconstruct the ionosphere based on satellite–ground radio signature measurements, as first reported by Austen et al. (1988) and widely used ever since.
With the completion and success of the global navigation satellite system (GNSS), CIT has entered a new stage of all-weather and all-time availability, providing comprehensive data for reconstructing the ionospheric electron density (Ssessanga et al., 2021; Hu TY et al., 2024). Prol et al. (2021), for instance, performed global-scale tomography with the GNSS data at more than 2700 stations to explore the ionospheric variability during the geomagnetic disturbance period, and Song R et al. (2021) used datasets collected by 800 GNSS ground-based receivers located at 25°N to 50°N and 125°E to 150°E to detect ionospheric disturbances in Japan. However, an ill-posed problem is engendered by the incomplete coverage of the measurements (Lu WJ et al., 2021a). To solve the ill-posed problem, Yao YB et al. (2020) restricted the initial ionospheric electron density by using the inverse distance weighted interpolation, thereby ensuring the smoothness of the reconstructed region. Wen DB et al. (2020) proposed a new approach by imposing a horizontal and vertical smoothing constraint on the tomography system to improve the accuracy of the CIT. Many efforts were also made to increase the observations for full coverage to address the ill-posed problem. Chen BY et al. (2019) and Lu WJ et al. (2021b) created virtual reference stations to deduce the GNSS rays, and their findings suggested a significant decrease in the slant total electron content (sTEC) errors. Ren XD et al. (2021) incorporated data from low-earth-orbit satellites to improve GNSS-based CIT, which reduced the root mean square errors of the sTEC by 15% and 20.3% at most during low and high solar activity years, respectively.
The question is now whether CIT can rebuild the ionosphere with complete coverage of the measurements. Previous studies have typically assessed CIT reconstructions by using the total electron content (TEC). However, assessments of CIT rebuilding in the vertical profiles are lacking, which results in questionable effectiveness of the increasing observations. The function of CIT is to obtain a designed ionosphere by modulating the initial background to be consistent with the radio observations (Bruno et al., 2020). The initial ionospheric state would be expected to play a vital role in the CIT operation (Wang C et al., 2021). Ou M et al. (2012) integrated the International Reference Ionosphere (IRI) model and ionosonde data and used it as the initial CIT input. They demonstrated superior performance compared with that using the original IRI model. Wang C et al. (2021) assimilated the ionosonde data with ground-based radar measurements to obtain the initial condition for CIT, which led to a substantial enhancement in ionosphere reconstruction. Jin SG and Li D (2018) decreased the standard deviation (STD) in the TEC by more than 20% by updating the initial ionospheric states, and Wen DB et al. (2012) confirmed this expectation. Thus, the limitations of CIT reconstruction solely through increased measurements require further comprehensive assessments.
To achieve the purpose of quantifying limitations, in this work we first construct a physics-based ionospheric environment from the Thermosphere Ionosphere Electrodynamics General Circulation Model (TIEGCM) simulation, and then design a virtual GNSS–ground network to obtain measurements of full coverage to circumvent the ill-posed problem mentioned. The constructed measurements are derived by accumulating free electron densities along the radio paths, and consistent with conventional practices, the IRI model computations are utilized to provide the initial iterative conditions for CIT reconstruction. Widely used CIT methods, namely the algebraic reconstruction technique (ART), the multiplicative algebraic reconstruction technique (MART), and the simultaneous iterative reconstruction technique (SIRT; Das and Shukla, 2011; Nandi and Bandyopadhyay, 2015; Prol et al., 2017), are used to rebuild the targeted ionosphere, and the performance of each is further assessed. Our results indicate that the initial conditions during the CIT procedure play an essential role, and they highlight the potential risk of the monitoring application.
In this study, the TIEGCM simulation serves as the background ionosphere, and IRI outputs provide the initial states for CIT. The TIEGCM is a physics-based model simulating reasonable variability in the ionosphere–thermosphere system (Qian LY et al., 2014). The virtual measurements of the sTEC, the critical parameter in tomography, are then expressed as follows:
sTEC=S∫RNe⋅ds, | (1) |
where the electron density Ne is along the ray path between the satellite and the ground receiver. However, because the ionosphere is divided into grids during the CIT procedure, the expression of the sTEC derivation is revised as follows:
sTECi=m∑j=1ai,jNej, | (2) |
where sTECi is the
Figure 1 is a schematic diagram of the optimized design of data coverage. In actual GNSS observations, the regions without GNSS rays passing through them create data gaps that lead to ill-posed problems in CIT, and the nonuniform distribution affects the weight allocation in CIT. Thus, the GNSS network in this study is designed to be completely covered and uniformly distributed to avoid these issues. To reduce the computational complexity, this study concentrates on the region of the equator. The satellites and ground receivers are positioned at the equator and several longitudinal degrees apart. Consequently, the ill-posed problem could be settled. Notably, this study concentrates on the performance of CIT during magnetic quiet periods, and the situation under disturbance conditions will be investigated in the future.
Austen et al. (1988) proposed the ART, which performs the tomography by linearly assimilating the initial ionosphere with the observations. The iteration procedure can be expressed as follows:
{{{\boldsymbol{x}}^{k + 1}}} = {{{\boldsymbol{x}}^k}} + \lambda \frac{{{y_i} - \displaystyle\sum\limits_{j = 1}^m {{a_{i,j}}x_{i,j}^k} }}{{\displaystyle\sum\limits_{j = 1}^m {a_{i,j}^2} }} {{{\boldsymbol{a}}_i}}, | (3) |
The subscripts
Compared with the ART, the MART is an entropy-optimization algorithm based on the following nonlinear iterations:
{{{\boldsymbol{x}}}^{k+1}}={{{\boldsymbol{x}}}^{k}}\cdot {\left(\frac{{y}_{i}}{{\displaystyle \sum _{j=1}^{m}{a}_{i,j}{x}_{i,j}^{k}}}\right)}^{\tfrac{\lambda {{{\boldsymbol{a}}}_{i}}}{{\sum\limits _{j=1}^{m}{a}_{i,j}^{2}}}}, | (4) |
{\left( {\frac{{{y_i}}}{{\displaystyle\sum\limits_{j = 1}^m {{a_{i,j}}x_j^k} }}} \right)^{\frac{{\lambda {{{\boldsymbol{a}}_i}} }}{{\sum\limits_{j = 1}^m {a_{i,j}^2} }}}} = \left( {\begin{array}{*{20}{c}} {{{\left( {\frac{{{y_i}}}{{\displaystyle\sum\limits_{j = 1}^m {{a_{i,j}}x_j^k} }}} \right)}^{\frac{{\lambda {a_{i,1}}}}{{\sum\limits_{j = 1}^m {a_{i,j}^2} }}}}} \\ {{{\left( {\frac{{{y_i}}}{{\displaystyle\sum\limits_{j = 1}^m {{a_{i,j}}x_j^k} }}} \right)}^{\frac{{\lambda {a_{i,2}}}}{{\sum\limits_{j = 1}^m {a_{i,j}^2} }}}}} \\ {\cdots} \\ {{{\left( {\frac{{{y_i}}}{{\displaystyle\sum\limits_{j = 1}^m {{a_{i,j}}x_j^k} }}} \right)}^{\frac{{\lambda {a_{i,m}}}}{{\sum\limits_{j = 1}^m {a_{i,j}^2} }}}}} \end{array}} \right) . | (5) |
Equation (4) is similar to Equation (2), except that the term
The SIRT was developed from the ART and is well suited for parallel implementation because it displays a comparably faster convergence (van Dijke et al., 1992). The iteration is expressed as follows:
{{{\boldsymbol{x}}^{k + 1}}} = {{{\boldsymbol{x}}^k}} + \lambda \sum\limits_{i = 1}^n {\frac{{{y_i} - \displaystyle\sum\limits_{j = 1}^m {{a_{i,j}}x_j^k} }}{{\displaystyle\sum\limits_{j = 1}^m {a_{i,j}^2} }} {{{\boldsymbol{a}}_i}} }. | (6) |
Again, the parameters here are the same as the ones above, but
Algorithm | Processing | Reference |
ART | Assimilates GNSS rays one by one through linear iteration | Austen et al. (1988) |
MART | Assimilates GNSS rays one by one through nonlinear iteration | Raymund et al. (1990) |
SIRT | Assimilates GNSS rays simultaneously through linear iteration | van Dijke et al. (1992) |
This section describes the performance of the three CIT algorithms. The TIEGCM simulates the target equatorial ionosphere at 10:00 universal time (UT) on January 1, 2007, when the solar and geomagnetic conditions are quiet, with F10.7 (solar radio flux at 10.7 cm) being 87 solar flux units and the Ap index being 7, respectively. The altitude covers a range between 100 and 500 km, and the ionosphere is gridded with resolutions of 1° in longitude and 10 km in height. Thus, the total number of grids is 14,400. The GNSS satellites are designed to be located at the equator 20,000 km away from the Earth’s center. The longitudinal interval is 2° for the satellite network and 0.5° for the receivers. In this way, we can obtain 40,860 measurements simultaneously, and the ill-posed problem will not affect the final reproduction.
Figure 2 compares the target ionosphere and CIT outcomes. As shown in Figure 2a, the target ionosphere is enhanced at 250–450 km, displaying a substantial altitudinal dependence. Meanwhile, the electron density decreases to a minimum until approximately −60°, then increases to a peak at approximately 120°. These characteristics are related to day–night ionospheric evolution and are consistent with the present shared understanding. The CIT outcomes in Figures 2b–2d reproduce the climatological behavior of the ionosphere but do not capture the small-scale variability. For instance, the maximum near 120° in the ART reproduction (Figure 2b) is lower than that of the target ionosphere at the exact longitude, and the peak altitudes in the ART are comparably lower than those in the TIEGCM. The MART and SIRT outcomes in Figures 2c and 2d show performance similar to that of the ART. However, the difference is that the peak altitudes in the ART reproduction in the nighttime, within longitudes from −120° to −90°, disappear, which does not happen in the performance of MART or SIRT.
Figure 3 compares the average profiles and STDs of the residuals from the TIEGCM. As shown, the average profile of the TIEGCM (solid black line) peaks at 360 km. However, the vertical profiles of the CIT reconstructions and the IRI model are similar, and they peak at 330 km, which is significantly different from the TIEGCM. The error bars in Figure 3 indicate differences of the CIT outcomes and the IRI model from the TIEGCM simulation. The IRI model obviously has more excellent STDs than the CIT outcomes, which suggests an improvement when using the CIT methodology to rebuild the ionosphere. Furthermore, the minimal STDs indicate that the MART performs better than the ART and SIRT. However, all the CIT outcomes are more or less similar to the IRI model, demonstrating a significant dependence of the CIT iteration on the initial condition.
The indication that the initial value plays an essential role is also demonstrated in Figure 4, which presents in detail the differences of the IRI model and the CIT results from the targeted ionosphere as functions of longitude and altitude. As shown in Figure 4a, the result of the IRI model is generally lower than that of the TIEGMC simulation in the nighttime, especially at the altitudinal ranges of 250–450 km. In contrast, the IRI computation near a 300-km altitude is higher than that of the TIEGCM in the daytime. Thus, the gap between the initial condition of the iteration and the final target is noticeable. However, characteristics of IRI residuals also occur in the CIT situations (Figures 2b–2d), except that the magnitude is substantially reduced. These CIT methodologies can decrease the deviations but fail to reshape the vertical structure to meet the target, again indicating that the initial condition is essential during the iteration.
Figure 5 depicts the vertical profiles from both models and the CIT results at typical longitudes. The selection of the three longitudes is primarily based on the differences in critical parameters of hmF2 and NmF2 between the initial tomography values and the target ionosphere. For instance, at −120° longitude, the discrepancy in NmF2 between the IRI model and the TIEGCM is substantial, whereas it is small in hmF2. A considerable discrepancy occurs at −20° longitude in both hmF2 and NmF2. However, at 80° longitude, the discrepancy exists mainly in hmF2. At a longitude of −120° (Figure 5a), the profile from TIEGCM peaks at 310 km with the NmF2 of 2.75 × 1011 el/m3, whereas the IRI profile also peaks at 310 km but with a different magnitude of 0.56 × 1011 el/m3. In this case, the rebuilt hmF2 equals the target ionosphere (black line), whereas the NmF2 does not, except for the reconstruction by the MART. Moreover, it is abnormal for the electron density at the top of the profile section to become unusually large in ART rebuilding. At the longitude of −20° (Figure 5b), the profile from TIEGCM peaks at 350 km with the NmF2 of 4.61 × 1011 el/m3. Meanwhile, the IRI profile peaks at an altitude of 300 km, but the peak magnitude is comparably higher, at 6.18 × 1011 el/m3. In this situation, the profile peaks of the ART, MART, and SIRT are consistent with that of the IRI model, whereas the NmF2 values of the CIT profiles are generally lower than that of the IRI model. As in the case of 80° longitude given in Figure 5c, the TIEGCM profile peaks at 370 km with the NmF2 of 8.23 × 1011 el/m3, whereas the IRI peak occurs at 330 km with the peak density of 8.20 × 1011 el/m3. Thus, the IRI hmF2 differs from the TIEGCM value, but NmF2 is similar in the two models. In this instance, the NmF2 and hmF2 of all CIT reconstructions are consistent with IRI model values.
Previous investigations have indicated that the initial values from the IRI model substantially influence the outcomes of CIT. In the ensuing discussion, we investigate how NmF2 and hmF2 evolve throughout iterations initiated from the IRI model, and Figure 6 displays this in the ART. At the longitude of −120° (Figure 6a), the first iteration result is similar to the IRI, except for the linearly enlarged magnitude in the electron density along the entire profile. However, this vertical profile becomes unreasonable during the iteration in which the profile peak disappears, so the variations in the difference between NmF2 and hmF2 are not illustrated in Figures 6d and 6g. At the longitude of −20° (Figure 6b), the first iteration profile of ART is again similar to that of the IRI model, and the profile of the higher order iteration shows little change. Figure 6e gives the NmF2 deviations from the target of the TIEGCM as a function of the iteration order, and the relative deviation decreases from 34.14% to near zero. Figure 6h exhibits the evolution of hmF2, and despite the 10-km change in the first iteration (because of the vertical resolution in this work, and likewise hereafter), the hmF2 gap remains the same for all iterations. At the longitude of 80° (Figure 6c), the iteration profiles are much the same as the IRI result, even though the peak altitude is different between the IRI model and the target. Figures 6f and 6i support this examination.
Figures 7 and 8 depict the performance of MART and SIRT at the specified longitudes. A notable distinction at the longitude of −120° is that MART performs better than ART and SIRT, as evidenced by the comparably low deviation in NmF2 and hmF2 from the TIEGCM target in Figures 7d and 7g. The relative deviation of NmF2 during the iteration moves from −79.59% to approximately −5% (Figure 7d), but hmF2 remains the same throughout the entire iteration procedure (Figure 7g). In Figures 8a–8c, the SIRT profiles of the first iteration have peak altitudes similar to those in the initial state, with the modulations existing only in the magnitudes, and no significant changes occur in the following iterations. The patterns in the rest denote the variations in NmF2 and hmF2 during the iterations, which suggest an excellent but biased convergence.
The examinations above suggest that the initial condition plays a vital role within the CIT iterations at the typical longitudes; hence, Figure 9 further provides details over all longitudes. Figures 9a and 9b display differences in NmF2 and hmF2, respectively, between the target background (TIEGCM) and the initial condition (IRI). At longitudes ranging from −160° to −60°, the NmF2 values of the IRI model are smaller than those of the TIEGCM, and the hmF2 values for the IRI and the TIEGCM are comparable. Within the longitudes from −40° to 0°, the NmF2 values of the IRI are greater, but the hmF2 values are more minor, respectively, compared with those of the TIEGCM. At the longitude interval between 50° and 180°, the NmF2 values of the IRI and TIEGCM are comparable. In contrast, the hmF2 values are smaller in the IRI than the TIEGCM, indicating a substantial gap between the target ionosphere and the initial conditions. Figure 9d shows that the difference in NmF2 is significantly reduced after CIT reconstruction. Notably, at longitudes between −121° and −80°, the vertical profiles of ART are unreasonable, as previously seen in Figure 6, so the differences in this interval are not displayed. Figure 9e is similar to Figure 9d, but for the differences in hmF2. As shown, the hmF2 variations are similar to those in Figure 9b, suggesting that CIT is ineffective in reducing the hmF2 gap. Figure 9c presents the correlation coefficients of the vertical profiles of CIT results from the TIEGCM across all longitudes. As shown, the correlation coefficients for ART and SIRT underwent a significant drop in the nighttime of the longitude interval from −180° to −120°, reaching their lowest values of 0.67 and 0.84, respectively. In contrast, MART consistently maintained correlation coefficients above 0.97. The correlation coefficients for ART, MART, and SIRT are comparable for the other longitudes.
The findings above indicate that the initial values had a substantial impact on the CIT outcomes. Some might dispute whether this study is suitable for actual applications. It should be pointed out that the initial conditions derived from the IRI are widely used in CIT, and indeed, discrepancies between the actual ionosphere and empirical model outputs are expected, especially during geomagnetic storms. Thus, the initial condition with high precision for tomography benefits the ionospheric reconstruction and its practical applications. In addition to the accuracy of model development, previous investigations have suggested that the hmF2 values can be obtained well from occultation measurements (Tsai et al., 2002; Chen P et al., 2017). Thus, blending the occultation results is expected to shed light on the CIT reconstruction, and this will be investigated in the future.
In this study, we used a virtual environment derived from the TIEGCM as the backdrop for our ionospheric investigations. An equator observational network of GNSS with longitudinal intervals of 0.5° and 2° for receivers and satellites was designed to ensure comprehensive coverage of measurements, thereby resolving the ill-posed problem inherent in the CIT procedure. Three tomography methods, the ART, MART, and SIRT, were used to reconstruct the target ionospheric electron density distribution. The results indicated that conventional tomography captures the general character of the target ionosphere but not the small-scale variations. For instance, all CIT reconstructions failed to reduce the hmF2 difference between the initial value and the target ionosphere. Furthermore, in the case of hmF2 being comparable, the difference in NmF2 also affects the ability of tomography to reconstruct the vertical ionospheric structures, except for the MART methodology. This work highlights the importance of the reference during iterations, although more effects are required to improve the accuracy of conventional tomography for the ionosphere.
This work was supported by the National Key R&D Program of China (Grant No. 2022YFF0503702), the National Natural Science Foundation of China (Grant No.
The authors declare that they have no competing interests.
H. Ruan and A. Qi provided the initial idea and designed the research, and A. Qi developed the program, processed the data, and wrote the manuscript. All authors provided critical feedback and helped shape the analysis and manuscript.
Austen, J. R., Franke, S. J., and Liu, C. H. (1988). Ionospheric imaging using computerized tomography. Radio Sci., 23(3), 299–307. https://doi.org/10.1029/RS023i003p00299
|
Bruno, J., Mitchell, C. N., Bolmgren, K. H. A., and Witvliet, B. A. (2020). A realistic simulation framework to evaluate ionospheric tomography. Adv. Space Res., 65(3), 891–901. https://doi.org/10.1016/j.asr.2019.11.015
|
Bust, G. S., and Mitchell, C. N. (2008). History, current state, and future directions of ionospheric imaging. Rev. Geophys., 46(1), RG1003. https://doi.org/10.1029/2006rg000212
|
Chen, B. Y., Wu, L. X., Dai, W. J., Luo, X. M., and Xu, Y. (2019). A new parameterized approach for ionospheric tomography. GPS Solut., 23(4), 96. https://doi.org/10.1007/s10291-019-0893-4
|
Chen, P., Yao, Y. B., and Yao, W. Q. (2017). Global ionosphere maps based on GNSS, satellite altimetry, radio occultation and DORIS. GPS Solut., 21(2), 639–650. https://doi.org/10.1007/s10291-016-0554-9
|
Das, S. K., and Shukla, A. K. (2011). Two-dimensional ionospheric tomography over the low-latitude Indian region: An intercomparison of ART and MART algorithms. Radio Sci., 46(2), RS2005. https://doi.org/10.1029/2010rs004350
|
Hu, T. Y., Xu, X. H., and Luo, J. (2024). Improving the computerized ionospheric tomography performance through a neural network-based initial IED prediction model. IEEE Trans. Geosci. Remote Sens., 62, 5800117. https://doi.org/10.1109/TGRS.2023.3339166
|
Jin, S. G., and Li, D. (2018). 3-D ionospheric tomography from dense GNSS observations based on an improved two-step iterative algorithm. Adv. Space Res., 62(4), 809–820. https://doi.org/10.1016/j.asr.2018.05.032
|
Lu, W. J., Ma, G. Y., and Wan, Q. T. (2021a). A review of voxel-based computerized ionospheric tomography with GNSS ground receivers. Remote Sens., 13(17), 3432. https://doi.org/10.3390/rs13173432
|
Lu, W. J., Ma, G. Y., Wan, Q. T., Li, J. H., Wang, X. L., Fu, W. Z., and Maruyama, T. (2021b). Virtual reference station-based computerized ionospheric tomography. GPS Solut., 25(1), 8. https://doi.org/10.1007/s10291-020-01039-1
|
Luo, X. M., Gu, S. F., Lou, Y. D., Xiong, C., Chen, B. Y., and Jin, X. Y. (2018). Assessing the performance of GPS precise point positioning under different geomagnetic storm conditions during solar cycle 24. Sensors, 18(6), 1784. http://doi.org/10.3390/s18061784
|
Nandi, S., and Bandyopadhyay, B. (2015). Study of low-latitude ionosphere over Indian region using simultaneous algebraic reconstruction technique. Adv. Space Res., 55(2), 545–553. https://doi.org/10.1016/j.asr.2014.10.018
|
Ou, M., Zhang, H. B., and Zhen, W. M. (2012). GPS-based ionospheric tomography with constrained IRI as a regularization. In ISAPE2012 (pp. 656–659). Xi’an, China: IEEE. http://doi.org/10.1109/ISAPE.2012.6408856
|
Prol, F. D. S., and Camargo, P. D. O. (2016). Ionospheric tomography using GNSS: Multiplicative algebraic reconstruction technique applied to the area of Brazil. GPS Solut., 20(4), 807–814. https://doi.org/10.1007/s10291-015-0490-0
|
Prol, F. S., Camargo, P. O., and Muella, M. T. A. H. (2017). Numerical simulations to assess ART and MART performance for ionospheric tomography of chapman profiles. An. Acad. Bras. Ciênc., 89(3), 1531–1542. https://doi.org/10.1590/0001-3765201720170116
|
Prol, F. S., Kodikara, T., Hoque, M. M., and Borries, C. (2021). Global-scale ionospheric tomography during the March 17, 2015 geomagnetic storm. Space Weather, 19(12), e2021SW002889. https://doi.org/10.1029/2021sw002889
|
Qian, L. Y., Burns, A. G., Emery, B. A., Foster, B., Lu, G., Maute, A., Richmond, A. D., Roble, R. G., Solomon, S. C., and Wang, W. B. (2014). The NCAR TIE-GCM: A community model of the coupled thermosphere/ionosphere system. In J. Huba, et al. (Eds.), Modeling the Ionosphere–Thermosphere System (pp. 73–83). Washington, DC: American Geophysical Union. http://doi.org/10.1002/9781118704417.ch7
|
Raymund, T. D., Austen, J. R., Franke, S. J., Liu, C. H., Klobuchar, J. A., and Stalker, J. (1990). Application of computerized tomography to the investigation of ionospheric structures. Radio Sci., 25(5), 771–789. https://doi.org/10.1029/RS025i005p00771
|
Ren, X. D., Mei, D. K., Zhang, X. H., Freeshah, M., and Xiong, S. (2021). Electron density reconstruction by ionospheric tomography from the combination of GNSS and upcoming LEO constellations. J. Geophys. Res.: Space Phys., 126(10), e2020JA029074. https://doi.org/10.1029/2020JA029074
|
Song, R., Hattori, K., Zhang, X. M., Liu, J. Y., and Yoshino, C. (2021). Detecting the ionospheric disturbances in Japan using the three-dimensional computerized tomography. J. Geophys. Res.: Space Phys., 126(6), e2020JA028561. https://doi.org/10.1029/2020ja028561
|
Ssessanga, N., Yamamoto, M., Saito, S., Saito, A., and Nishioka, M. (2021). Complementing regional ground GNSS-STEC computerized ionospheric tomography (CIT) with ionosonde data assimilation. GPS Solut., 25(3), 93. https://doi.org/10.1007/s10291-021-01133-y
|
Tang, J., Yao, Y. B., and Zhang, L. (2015). A TV-MART algorithm applied to computerized ionospheric tomography. Geomat. Inf. Sci. Wuhan Univ. (in Chinese), 40(7), 870–876. https://doi.org/10.13203/j.whugis20130049
|
Tsai, L. C., Liu, C. H., Tsai, W. H., and Liu, C. T. (2002). Tomographic imaging of the ionosphere using the GPS/MET and NNSS data. J. Atmos. Sol. Terr. Phys., 64(18), 2003–2011. https://doi.org/10.1016/S1364-6826(02)00218-3
|
van Dijke, M. C. A., van der Vorst, H. A., and Viergever, M. A. (1992). On the relation between ART, block-ART and SIRT. In A. E. Todd-Pokropek, et al. (Eds.), Medical Images: Formation, Handling and Evaluation (pp. 377–396). Berlin: Springer. http://doi.org/10.1007/978-3-642-77888-9_16
|
Wan, X., Xiong, C., Gao, S. Z., Huang, F. Q., Liu, Y. W., Aa, E., Yin, F., and Cai, H. T. (2021). The nighttime ionospheric response and occurrence of equatorial plasma irregularities during geomagnetic storms: A case study. Satell. Navig., 2(1), 23. https://doi.org/10.1186/s43020-021-00055-x
|
Wang, C., Zhao, H. S., Guo, W. L., Liu, B., and Chen, L. (2021). Improved computerized ionospheric tomography based on the spaceborne SAR, ionosonde and GPS data. In 2021 7th Asia-Pacific Conference on Synthetic Aperture Radar (APSAR) (pp. 1–4). Bali, Indonesia: IEEE. http://doi.org/10.1109/APSAR52370.2021.9688437
|
Wen, D. B., Wang, Y., and Norman, R. (2012). A new two-step algorithm for ionospheric tomography solution. GPS Solut., 16(1), 89–94. https://doi.org/10.1007/s10291-011-0211-2
|
Wen, D. B., Mei, D. K., and Du, Y. N. (2020). Adaptive smoothness constraint ionospheric tomography algorithm. Sensors, 20(8), 2404. https://doi.org/10.3390/s20082404
|
Xiong, C., Stolle, C., and Lühr, H. (2016). The Swarm satellite loss of GPS signal and its relation to ionospheric plasma irregularities. Space Weather, 14(8), 563–577. https://doi.org/10.1002/2016sw001439
|
Xiong, C., Xu, J. S., Stolle, C., van den Ijssel, J., Yin, F., Kervalishvili, G. N., and Zangerl, F. (2020). On the occurrence of GPS signal amplitude degradation for receivers on board LEO satellites. Space Weather, 18(2), e2019SW002398. https://doi.org/10.1029/2019SW002398
|
Yao, Y. B., Zhai, C. Z., Kong, J., Zhao, C. J., Luo, Y. Y., and Liu, L. (2020). An improved constrained simultaneous iterative reconstruction technique for ionospheric tomography. GPS Solut., 24(3), 68. https://doi.org/10.1007/s10291-020-00981-4
|
ZhiPeng Ren, YunBo Liu, WeiXing Wan, Yong Wei, XinAn Yue. 2025: MITM: A new Mars Ionosphere−Thermosphere Model. Earth and Planetary Physics, 9(2): 337-345. DOI: 10.26464/epp2024064 | |
Angga Yolanda Putra, Theodosius Marwan Irnaka, Prayitno Abadi, La Ode Muhammad Musafar Kilowasid, Fitri Nuraeni, Erlansyah, Suraina1, Afif Rakhman. 2025: Occurrence of Ionospheric Scintillation During Geomagnetic Storms in Indonesia (2003–2024) Using Superposed Epoch Analysis. Earth and Planetary Physics, 9. DOI: 10.26464/epp2025048 | |
Duan Zhang, QingHe Zhang, Kjellmar Oksavik, ZanYang Xing, L. R. Lyons, HuiGen Yang, Tong Xu, Marc Hairston, XiangYu Wang, YuZhang Ma, GuoJun Li, Yong Wang, Sheng Lu, and Jin Wang. 2025: A statistical survey of polar cap cold and hot patches in the Southern Hemisphere using a DMSP Satellite. Earth and Planetary Physics, 9(0). DOI: 10.26464/epp2025044 | |
Jin Hu, HaiBing Ruan, FuQing Huang, ShengYang Gu, XianKang Dou. 2024: Assessment of International GNSS Service Global Ionosphere Map products over China region based on measurements from the Crustal Movement Observation Network of China. Earth and Planetary Physics, 8(2): 400-407. DOI: 10.26464/epp2024004 | |
JunJie Chen, WenBin Wang, JiuHou Lei, Tong Dang. 2023: Solar cycle variations in equatorial ionospheric zonal electric fields near sunrise. Earth and Planetary Physics, 7(3): 378-388. DOI: 10.26464/epp2023037 | |
ChunHua Jiang, Rong Tian, LeHui Wei, GuoBin Yang, ZhengYu Zhao. 2022: Modeling of kilometer-scale ionospheric irregularities at Mars. Earth and Planetary Physics, 6(2): 213-217. DOI: 10.26464/epp2022011 | |
Yi Liu, Chen Zhou, Tong Xu, Qiong Tang, ZhongXin Deng, GuanYi Chen, ZhuangKai Wang. 2021: Review of ionospheric irregularities and ionospheric electrodynamic coupling in the middle latitude region. Earth and Planetary Physics, 5(5): 462-482. DOI: 10.26464/epp2021025 | |
Tong Dang, JiuHou Lei, WenBin Wang, MaoDong Yan, DeXin Ren, FuQing Huang. 2020: Prediction of the thermospheric and ionospheric responses to the 21 June 2020 annular solar eclipse. Earth and Planetary Physics, 4(3): 231-237. DOI: 10.26464/epp2020032 | |
LiBo Liu, WeiXing Wan. 2020: Recent ionospheric investigations in China (2018–2019). Earth and Planetary Physics, 4(3): 179-205. DOI: 10.26464/epp2020028 | |
LiBo Liu, WeiXing Wan. 2018: Chinese ionospheric investigations in 2016–2017. Earth and Planetary Physics, (2): 89-111. DOI: 10.26464/epp2018011 |
Algorithm | Processing | Reference |
ART | Assimilates GNSS rays one by one through linear iteration | Austen et al. (1988) |
MART | Assimilates GNSS rays one by one through nonlinear iteration | Raymund et al. (1990) |
SIRT | Assimilates GNSS rays simultaneously through linear iteration | van Dijke et al. (1992) |