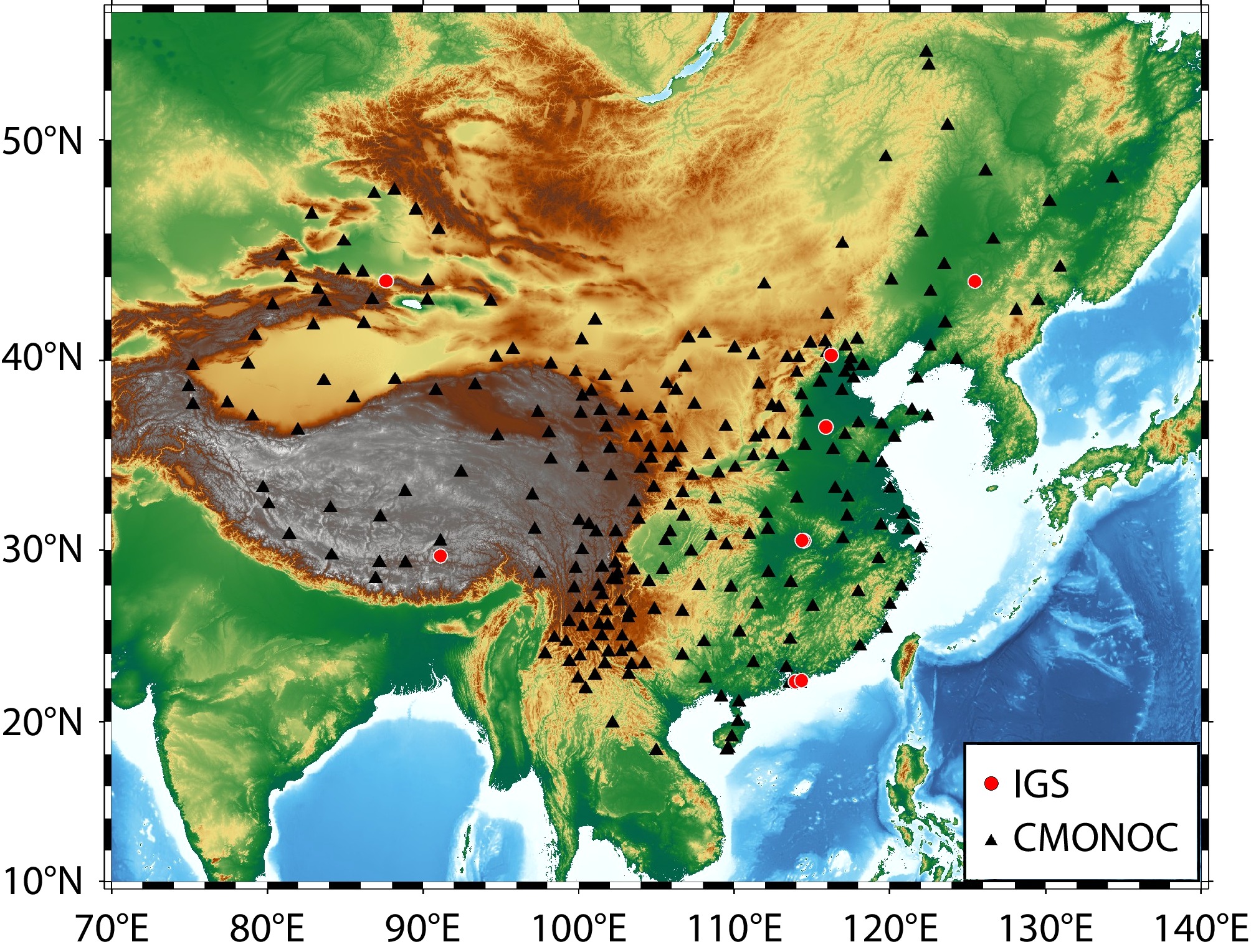
Citation: | Hu, J., Ruan, H. B., Huang, F. Q., Gu, S. Y., and Dou, X. K. (2024). Assessment of International GNSS Service Global Ionosphere Map products over China region based on measurements from the Crustal Movement Observation Network of China. Earth Planet. Phys., 8(2), 400–407. DOI: 10.26464/epp2024004 |
The global ionosphere maps (GIM) provided by the International GNSS Service (IGS) are extensively utilized for ionospheric morphology monitoring, scientific research, and practical application. Assessing the credibility of GIM products in data-sparse regions is of paramount importance. In this study, measurements from the Crustal Movement Observation Network of China (CMONOC) are leveraged to evaluate the suitability of IGS-GIM products over China region in 2013–2014. The indices of mean error (ME), root mean square error (RMSE), and normalized RMSE (NRMSE) are then utilized to quantify the accuracy of IGS-GIM products. Results revealed distinct local time and latitudinal dependencies in IGS-GIM errors, with substantially high errors at nighttime (NRMSE: 39%) and above 40° latitude (NRMSE: 49%). Seasonal differences also emerged, with larger equinoctial deviations (NRMSE: 33.5%) compared with summer (20%). A preliminary analysis implied that the irregular assimilation of sparse IGS observations, compounded by China’s distinct geomagnetic topology, may manifest as error variations. These results suggest that modeling based solely on IGS-GIM observations engenders inadequate representations across China and that a thorough examination would proffer the necessary foundation for advancing regional total electron content (TEC) constructions.
Total electron content (TEC) is an important physical parameter used in describing ionospheric properties. Highly accurate TEC models play a vital role in forecasting and elucidating ionospheric variability. Substantial efforts have been made to recreate the ionospheric morphology and evolution as realistically as possible through empirical modeling and numerical simulations (e.g., Chiu, 1975; Roble et al., 1988; Huba et al., 2000; Schunk et al., 2003, 2004, 2005; Aa et al., 2015; Lin CY et al., 2020; Tang RX et al., 2020; Dang T et al., 2020). A systematic assessment of model specifications benefits both practical applications and scientific research.
In recent decades, several empirical ionospheric models have been established (Hernández-Pajares et al., 2009; Goss et al., 2019; Calabia and Jin SG, 2020; Li ZS et al., 2020). The empirical model of the International Reference Ionosphere (IRI), developed by using ionosonde, incoherent scatter, rocket, topside sounder, and various satellite observations (Bilitza et al., 2016), provides global-scale ionospheric parameters from the lower boundary to a user-specified upper boundary and is widely utilized in the ionospheric community. The three-dimensional electron density is also mapped by combining fully polarimetric synthetic aperture radar (SAR) images and the IRI model, with good performance (Zhu W et al., 2020). Nevertheless, various efforts have illustrated that the IRI model is still subjected to in-depth study. Wang C et al. (2016) noted that significant discrepancies exist between the IRI data and the observed values, particularly during geomagnetic storms. Studies evaluating IRI-2012 (Akala et al., 2015) and IRI-2016 (Rajana et al., 2022) against the global positioning system (GPS) TEC also highlight the need for continued IRI improvement. Similar GPS TEC comparisons have quantified accuracy limitations in the NeQuick model (Jodogne et al., 2005; Coïsson et al., 2006; Bidaine and Warnant, 2010; Wang H and Zhang KD, 2017). Notably, empirical models provide well-parameterized climatological behaviors instead of their dynamic evolution.
The International GNSS (Global Navigation Satellite System) Service (IGS) assimilates diverse observations to generate and provide real-time TEC at a global scale (e.g., Wang L et al., 2018; Schmölter et al., 2021; Chen Z et al., 2022), which are widely used in practical applications and scientific research. Yang JL et al. (2023) analyzed the performance of the global dilution of precision and positioning for the BeiDou Navigation Satellite System Phase III (BDS-3). Alkan et al. (2022) examined the performance in real-time Precise Point Positioning (RT-PPP) applications in Antarctica. Moreover, the IGS products are used in understanding and monitoring ionospheric behaviors, including variability investigations, earthquake responses, and empirical model development (Stankov et al., 2010; Chakraborty et al., 2015; Şentürk, 2020; Şentürk et al., 2021). However, the more than 400 IGS stations display a nonuniform global distribution, with a disproportionate concentration in the Northern Hemisphere and Europe/North America (Zhang Q and Zhao QL, 2019). The limited observational coverage with only 7 stations across China results in considerable challenges in validating extant models or constructing regional Chinese ionospheric models when leveraging IGS Global Ionosphere Map (GIM) products.
In this work, a systematic validation of IGS-GIM products in China is conducted by using independent Crustal Movement Observation Network of China (CMONOC) observations from 2013 to 2014. The mean error (ME), root mean square error (RMSE), and normalized root mean square error (NRMSE) are selected to quantify the suitability of IGS-GIM products. Section 2 briefly details the observations and their data preprocessing. Section 3 presents a comparison between the IGS-GIM and CMONOC-TEC. The results reveal a significant local time and latitudinal dependence and seasonal variation in discrepancies of the IGS-GIM products. Section 4 provides a summary of the main findings.
The IGS is a collaborative organization founded by a consortium of universities, research centers, and geodetic and space agencies worldwide. Its primary mission is to provide the highest quality GNSS data and related products on a freely accessible basis for scientific advancement and public benefit (Dow et al., 2009; Montenbruck et al., 2017). The GIMs used in this study are among the IGS products released by the Center for Orbit Determination of Europe from IGS products based on the expression of spherical harmonic functions, and they are commonly used for ionospheric studies. The time interval of GIMs within each day is 2 h, which produces 2-hourly snapshots of the global ionosphere. The spatial resolution of each TEC map is 5° × 2.5° in the geographic longitude and latitude, respectively. The red circles in Figure 1 show the distribution of IGS stations over China region, which illustrates an uneven distribution and results in sparse data coverage.
The TEC measurements from approximately 260 stations of the CMONOC are used to quantify the accuracy of IGS-GIM products over China region in the years 2013–2014. The CMONOC stations are shown by the black triangles in Figure 1, with the region covering from 70°E to 140°E in longitude and 15°N to 55°N in latitude. Slant TEC (sTEC) represents the integrated electron density of the ionosphere along the line of sight from the satellite to receiver, which is estimated by using the carrier-phase signal observations from ground-based GPS stations (Thomas et al., 2018; Ren XD et al., 2019). The equation is expressed as
sTEC=f21f2240.28(f21−f22)(L1−L2+λ1(N1+b1)−λ2(N2+b2)+ε), | (1) |
where
VTEC=sTEC×cos(arcsin(RsinZR+H)), | (2) |
where R is the Earth radius, H represents the height of the ionosphere, and Z stands for the satellite elevation angle for the point of observation.
Given the spatial distribution of CMONOC stations, measurements in the region within the longitudes of 90°E–120°E and latitudes of 20°N–45°N are utilized in the following investigation. Because the time resolution of the CMONOC-TEC is 30 s, CMONOC measurements within 6 min centered at the current time of GIM products are first collected, and these time-filtered data are then interpolated into the GIM grids, that is, spatial bins with a resolution of 5° (longitude) × 2.5° (latitude). As a consequence, the ME, RMSE, and NRMSE are utilized to quantify the uncertainties in IGS-GIM data over China region. The following equations express the computation (Lin CH et al., 2010; Wang H and Zhang KD, 2017):
ME=∑ni=1(TECIGS−TECCMONOC)n, | (3) |
RMSE=√∑ni=1(TECIGS−TECCMONOC)2n, | (4) |
NRMSE=√∑ni=1(2×(TECIGS−TECCMONOC)TECIGS+TECCMONOC)2n×100%, | (5) |
where n is the sample number at each spatial grid,
Figure 2 gives the monthly average of the IGS-GIM and the CMONOC-TEC over China region, associated with their deviations, at 12:00 universal time (UT) for June and December in the year 2013. As shown in Figure 2a, the IGS-GIM decreases with an increase in latitude. In addition, a clear longitudinal variation exists at low latitudes, which could be a reference to the local time dependence. Figure 2b presents the situation of the CMONOC-TEC. As shown, the CMONOC-TEC also displays a similar significant latitudinal characteristic. However, the TEC is much weaker at high latitudes in Figure 2b than in Figure 2a, and the longitudinal structure at low latitudes is smoothed. The
Since the longitudinal variation refers to the local time dependence in this work, the dependence of the variables of ME, RMSE, and NRMSE on the local time, latitude, and seasons are further investigated. Figure 3 depicts the variations in ME, RMSE, and NRMSE as a function of latitude and local time in different months. As shown in Figure 3a, the ME is significantly larger at higher latitudes before 10:00 local time (LT), and the maximum is approximately 8 TECu. This situation is quite different after 10:00 LT, when the ME peaks at a latitude of ~30° with an amplitude of approximately 8 TECu. In addition, the ME is negative with a minimum of −8 TECu in the 20°–25° latitude region, and the amplitude of the negative peak at a latitude of ~35° is approximately −2 TECu. Figure 3b illustrates the situation in June, which depicts a similar pattern as the one in Figure 3a, except that the magnitudes in Figure 3b are relatively small. Figure 3c is similar to Figure 3a except for the situation in September. However, the entire pattern is close to the one in March, with a comparable magnitude. The situation in December is shown in Figure 3d, which again depicts a similar variation, except that the 30° peak at 15:00 LT in Figure 3a shifts to a lower latitude, and the peak amplitude is approximately 7 TECu.
Figures 3e–3h show the local time and latitudinal variations of the RMSE. The RMSE exhibits an equatorward-extending trend that the peak time is generally earlier at higher latitudes. In March (Figure 3e), the peak of the RMSE in the 20°–30° latitudinal region occurs at 20:00 LT with an amplitude of 14 TECu, whereas the maximum of 35°–45° latitudes appears around 8:00 LT, and the peak value is approximately 7 TECu. The RMSE is comparably low in June (Figure 3f). The noticeable maximum in June occurs at 15:00 LT in the low latitudinal region of 20°–30°, and the magnitude is approximately 6–8 TECu. Figures 3g and 3h depict the situations in September and December, respectively, which are quite similar to the ones in March.
Figures 3i–3l illustrate variations in the NRMSE. The most significant phenomenon is that the maximum NRMSE is generally located at high latitudes during midnight. Besides these noticeable local time and latitudinal dependencies, the NRMSE also shows an obvious seasonal variation. The midnight maximum NRMSE is significantly larger in December (Figure 3l), with a magnitude greater than 100%, compared with those in other seasons, and it is the smallest in June (Figure 3j; ~60%). A secondary peak also occurs at the latitudes of 25°–30° before midnight except for the situation in June.
Figure 4 exhibits the index structures evaluated at different local times, which is more beneficial for quantifying latitudinal errors in the IGS-GIM products. As shown in Figure 4a, the ME becomes higher with latitude, increasing from 2:00 to 10:00 LT, and the magnitude achieves ~6 TECu at a latitude of 45°. However, the latitudinal variations from 12:00 to 24:00 LT are significantly different at another local time. The maximum ME is more than 8 TECu at a latitude of 45°, and the amplitude of the secondary peak at ~25° is approximately 6 TECu. Figure 4b presents the situation for the RMSE. The minimum RMSE from 2:00 to 10:00 LT appears around the 25°–30° latitudinal region, whereas the one at 12:00 LT occurs around 35° latitude. It is noteworthy that this minimum shifts toward lower latitudes in the post-noon period. The variations in the NRMSE (Figure 4c) exhibit a local time dependence similar to that observed for the ME. The peaks of structures increase with latitude, whereas the secondary peaks from 14:00 to 24:00 LT occur around 25°–30° latitude.
Figure 5 presents variations in the ME, RMSE, and NRMSE as a function of the month and latitude at different local times. As depicted in Figure 5a, the ME is markedly larger at higher latitudes, peaking in March and October with an amplitude of approximately 7 TECu. In addition, the ME is negative in summer at low latitudes, with a minimum of −6 TECu. The pattern at 8:00 LT (Figure 5b) is quite similar but with more pronounced magnitudes. In contrast, the variation in ME at 14:00 LT (Figure 5c) exhibits significant differences from the morphology at 2:00 LT. Compared with 2:00 LT, the two peaks shift to middle–low latitudes with a maximum of ~7 TECu. Moreover, a negative ME occurs in the 20°–25° latitude region. The morphology at 20:00 LT (Figure 5d) resembles that at 14:00 LT except that the peaks extend into the winter months. Additionally, the peaks at 20:00 LT are the highest compared with those at other local times, with an amplitude exceeding 8 TECu.
The seasonal and latitudinal variations in the RMSE are shown in Figures 5e–5h. At 2:00 LT (Figure 5e), the RMSE maximum of ~7 TECu emerges at ~45° latitude in April and October, whereas the minimum of 3 TECu occurs in July at 30° latitude. The overall structure at 8:00 LT (Figure 5f) resembles that at 2:00 LT, with some differences. Notably, the RMSE at 8:00 LT is comparatively higher, with a peak of 8 TECu in October and a slightly weaker maximum of 7 TECu in April. In contrast, the morphology at 14:00 LT (Figure 5g) diverges substantially from that at 2:00 LT. Compared with 2:00 LT, distinct peaks in the RMSE materialize around the equator with greater magnitudes, exceeding 11 TECu, in March and November. The distribution at 20:00 LT (Figure 5h) mirrors that at 14:00 LT but with relatively larger amplitudes.
Figures 5i–5l exhibit variations in the NRMSE. As shown, the NRMSE maxima materialize in the 40°–45° latitude region, with markedly higher peak values at nighttime (Figures 5i and 5l) compared with daytime (Figures 5j and 5k). Taking 20:00 LT as an illustrative example, Figure 5l shows the NRMSE peaking in the winter months, with magnitudes exceeding 80%, whereas the minima occur in July. An additional secondary peak emerges in winter at 30° latitude.
The ME, RMSE, and NRMSE at different local times are presented in Figure 6. For more direct comparisons, representative local times (i.e., 6:00, 12:00, 18:00, and 24:00 LT) are selected to quantitatively depict the seasonal variation. As shown in Figure 6a, the ME has two peaks in the first half and second half of the year, with the November maximum exceeding that in the first half year. The RMSE pattern (Figure 6b) resembles that of the ME aside from some key differences. Notably, the RMSE is higher during the daytime, whereas the ME exhibits the opposite dependence. Regarding the NRMSE (Figure 6c), the nighttime values are again markedly higher, with maxima concentrated in the winter months.
As discussed previously, the errors between the IGS-GIM product and the CMONOC-TEC exhibit local time and latitudinal dependencies, along with significant seasonal variations. Notably, the IGS-GIM dataset analyzed coincides with periods of high solar activity, potentially contributing to the observed deviations. Apart from solar influences, the modeling approaches used by IGS institutions further affect the accuracy of the IGS-GIM. Figure 7 shows the distribution of geomagnetic declination and geomagnetic latitude in geographic coordinates. The data are derived from the International Geomagnetic Reference Field. As depicted in Figure 7, the discrepancies between geographic (gray lines) and magnetic coordinates (blue and red lines) also introduce a considerable modulation of the ionospheric plasma owing to the ion drag along the geomagnetic field lines (Zhang SR et al., 2011; Hu J et al., 2022). The GIM products are superposed by the spherical harmonic functions requiring substantial measurements, whereas this is insufficient for IGS station coverage over China. This implies that interpolation of the TEC using sparse observations and spherical harmonics is ill-posed, yielding an unrealistic overestimated or negative TEC, degrading the model reliability. Additionally, intense atmospheric disturbances induce pronounced, intricate electron density fluctuations that cannot be captured rapidly by IGS models, inducing deviations from the CMONOC-TEC. However, the reliability of IGS-GIM products in other years and regions warrants further investigation.
This study evaluates the accuracy of IGS-GIM products from 2013 to 2014 in China by comparing them with CMONOC measurements. Discrepancies between the CMONOC-TEC and IGS-GIM are quantified by using the ME, RMSE, and NRMSE metrics. The key findings are as follows:
(1) A distinct local time dependence is evident in IGS-GIM deviations, with a higher average NRMSE (39%) at nighttime compared with other times (19%).
(2) After averaging by the local time, notable latitudinal variations emerge in IGS-GIM errors, with a peak NRMSE of 49% occurring above 40° latitude, whereas a minimum NRMSE of 18% is observed within the 20°–25° latitudinal band.
(3) Significant seasonal differences manifest in IGS-GIM deviations, with larger biases in spring (NRMSE: 30%) and autumn (37%) versus summer (20%).
(4) Discrepancies in the IGS-GIM likely stem from the geomagnetic environment and sparse observations in China.
These results elucidate the characteristics of IGS-GIM error variations over China. However, the limited measurements herein necessitate further investigation into the mechanisms underlying the identified biases.
This work was supported by the National Key R&D Program of China (Grant No. 2022YFF0503702), the National Natural Science Foundation of China (Grant Nos. 42074186, 41831071, 42004136, and 42274195), the Natural Science Foundation of Jiangsu Province (Grant No. BK20211036), the Specialized Research Fund for State Key Laboratories, and the University of Science and Technology of China Research Funds of the Double First-Class Initiative (Grant No. YD2080002013).
Aa, E., Huang, W. G., Yu, S. M., Liu, S. Q., Shi, L. Q., Gong, J. C., Chen, Y. H., and Shen, H. (2015). A regional ionospheric TEC mapping technique over China and adjacent areas on the basis of data assimilation. J. Geophys. Res.: Space Phys., 120(6), 5049–5061. https://doi.org/10.1002/2015JA021140
|
Akala, A. O., Somoye, E. O., Adewale, A. O., Ojutalayo, E. W., Karia, S. P., Idolor, R. O., Okoh, D., and Doherty, P. H. (2015). Comparison of GPS-TEC observations over Addis Ababa with IRI-2012 model predictions during 2010–2013. Adv. Space Res., 56(8), 1686–1698. https://doi.org/10.1016/j.asr.2015.07.017
|
Alkan, R. M., Erol, S., and Mutlu, B. (2022). Real-time multi-GNSS Precise Point Positioning using IGS-RTS products in Antarctic region. Polar Sci., 32, 100844. https://doi.org/10.1016/j.polar.2022.100844
|
Bidaine, B., and Warnant, R. (2010). Assessment of the NeQuick model at mid-latitudes using GNSS TEC and ionosonde data. Adv. Space Res., 45(9), 1122–1128. https://doi.org/10.1016/j.asr.2009.10.010
|
Bilitza, D., Altadill, D., Reinisch, B., Galkin, I., Shubin, V., and Truhlik, V. (2016). The international reference ionosphere: Model update 2016. In EGU General Assembly Conference Abstracts (no. EPSC2016-9671). Vienna: EGU.
|
Calabia, A., and Jin, S. G. (2020). New modes and mechanisms of long-term ionospheric TEC variations from global ionosphere maps. J. Geophys. Res.: Space Phys., 125(6), e2019JA027703. https://doi.org/10.1029/2019JA027703
|
Chakraborty, M., Kumar, S., De, B. K., and Guha, A. (2015). Effects of geomagnetic storm on low latitude ionospheric total electron content: A case study from Indian sector. J. Earth Syst. Sci., 124(5), 1115–1126. https://doi.org/10.1007/s12040-015-0588-3
|
Chen, Z., Liao, W. T., Li, H. M., Wang, J. S., Deng, X. H., and Hong, S. (2022). Prediction of global ionospheric TEC based on deep learning. Space Weather, 20(4), e2021SW002854. https://doi.org/10.1029/2021SW002854
|
Chiu, Y. T. (1975). An improved phenomenological model of ionospheric density. J. Atmos. Terr. Phys., 37(12), 1563–1570. https://doi.org/10.1016/0021-9169(75)90035-5
|
Coïsson, P., Radicella, S. M., Leitinger, R., and Nava, B. (2006). Topside electron density in IRI and NeQuick: Features and limitations. Adv. Space Res., 37(5), 937–942. https://doi.org/10.1016/j.asr.2005.09.015
|
Dang, T., Lei, J. H., Wang, W. B., Yan, M. D., Ren, D. X., and Huang, F. Q. (2020). Prediction of the thermospheric and ionospheric responses to the 21 June 2020 annular solar eclipse. Earth Planet. Phys., 4(3), 231–237. https://doi.org/10.26464/epp2020032
|
Dow, J. M., Neilan, R. E., and Rizos, C. (2009). The International GNSS Service in a changing landscape of global navigation satellite systems. J. Geod., 83(3), 191–198. https://doi.org/10.1007/s00190-008-0300-3
|
Goss, A., Schmidt, M., Erdogan, E., Görres, B., and Seitz, F. (2019). High-resolution vertical total electron content maps based on multi-scale B-spline representations. Ann. Geophys., 37(4), 699–717. https://doi.org/10.5194/angeo-37-699-2019
|
Hernández-Pajares, M., Juan, J. M., Sanz, J., Orus, R., Garcia-Rigo, A., Feltens, J., Komjathy, A., Schaer, S. C., and Krankowski, A. (2009). The IGS VTEC maps: A reliable source of ionospheric information since 1998. J. Geod., 83(3), 263–275. https://doi.org/10.1007/s00190-008-0266-1
|
Hu, J., Ruan, H. B., Lei, J. H., Zhong, J. H., and Owolabi, C. (2022). An investigation on the longitudinal variation in mid-latitude ionosphere based on CHAMP satellite observations. Earth Space Sci., 9(10), e2022EA002496. https://doi.org/10.1029/2022EA002496
|
Huang, F. Q., Dou, X. K., Lei, J. H., Lin, J., Ding, F., and Zhong, J. H. (2016). Statistical analysis of nighttime medium-scale traveling ionospheric disturbances using airglow images and GPS observations over central China. J. Geophys. Res.: Space Phys., 121(9), 8887–8899, https://doi.org/10.1002/2016JA022760
|
Huba, J. D., Joyce, G., and Fedder, J. A. (2000). Sami2 is Another Model of the Ionosphere (SAMI2): A new low-latitude ionosphere model. J. Geophys. Res.: Space Phys., 105(A10), 23035–23053. https://doi.org/10.1029/2000JA000035
|
Jodogne, J. C., Nebdi, H., and Warnant, R. (2005). GPS TEC and ITEC from digisonde data compared with NEQUICK model. Adv. Radio Sci., 2, 269–273. https://doi.org/10.5194/ars-2-269-2004
|
Li, Z. S., Wang, N. B., Hernández-Pajares, M., Yuan, Y. B., Krankowski, A., Liu, A., Zha, J., García-Rigo, A., Roma-Dollase, D., .. Blot, A. (2020). IGS real-time service for global ionospheric total electron content modeling. J. Geod., 94(3), 32. https://doi.org/10.1007/s00190-020-01360-0
|
Lin, C. H., Liu, C. H., Liu, J. Y., Chen, C. H., Burns, A. G., and Wang, W. (2010). Midlatitude summer nighttime anomaly of the ionospheric electron density observed by FORMOSAT-3/COSMIC. J. Geophys. Res.: Space Phys., 115(A3), A03308. https://doi.org/10.1029/2009ja014084
|
Lin, C. Y., Lin, C. C. H., Liu, J. Y., Rajesh, P. K., Matsuo, T., Chou, M. Y., Tsai, H. F., and Yeh, W. H. (2020). The early results and validation of FORMOSAT-7/COSMIC-2 space weather products: Global ionospheric specification and Ne-aided Abel electron density profile. J. Geophys. Res.: Space Phys., 125(10), e2020JA028028. https://doi.org/10.1029/2020JA028028
|
Montenbruck, O., Steigenberger, P., Prange, L., Deng, Z. G., Zhao, Q. L., Perosanz, F., Romero, I., Noll, C., Stürze, A., .. Schaer, S. (2017). The Multi-GNSS Experiment (MGEX) of the International GNSS Service (IGS)—Achievements, prospects and challenges. Adv. Space Res., 59(7), 1671–1697. https://doi.org/10.1016/j.asr.2017.01.011
|
Rajana, S. S. K., Shrungeshwara, T. S., Vivek, C. G., Panda, S. K., and Jade, S. (2022). Evaluation of long-term variability of ionospheric total electron content from IRI-2016 model over the Indian sub-continent with a latitudinal chain of dual-frequency geodetic GPS observations during 2002 to 2019. Adv. Space Res., 69(5), 2111–2125. https://doi.org/10.1016/j.asr.2021.12.005
|
Ren, X. D., Chen, J., Li, X. X., Zhang, X. H., and Freeshah, M. (2019). Performance evaluation of real-time global ionospheric maps provided by different IGS analysis centers. GPS Solut., 23(4), 113. https://doi.org/10.1007/s10291-019-0904-5
|
Roble, R. G., Ridley, E. C., Richmond, A. D., and Dickinson, R. E. (1988). A coupled thermosphere/ionosphere general circulation model. Geophys. Res. Lett., 15(12), 1325–1328. https://doi.org/10.1029/GL015i012p01325
|
Schmölter, E., Berdermann, J., and Codrescu, M. (2021). The delayed ionospheric response to the 27-day solar rotation period analyzed with GOLD and IGS TEC data. J. Geophys. Res.: Space Phys., 126(2), e2020JA028861. https://doi.org/10.1029/2020JA028861
|
Schunk, R., Scherliess, L., Sojka, J. J., Thompson, D. C., and Zhu, L. (2005). Ionospheric weather forecasting on the horizon. Space Weather, 3(8). https://doi.org/10.1029/2004SW000138
|
Schunk, R. W., Scherliess, L., and Sojka, J. J. (2003). Recent approaches to modeling ionospheric weather. Adv. Space Res., 31(4), 819–828. https://doi.org/10.1016/S0273-1177(02)00791-3
|
Schunk, R. W., Scherliess, L., Sojka, J. J., Thompson, D. C., Anderson, D. N., Codrescu, M., Minter, C., Fuller-Rowell, T. J., Heelis, R. A., .. Howe, B. M. (2004). Global assimilation of ionospheric measurements (GAIM). Radio Sci., 39(1), RS1S02. https://doi.org/10.1029/2002RS002794
|
Şentürk, E. (2020). Investigation of global ionospheric response of the severe geomagnetic storm on June 22–23, 2015 by GNSS-based TEC observations. Astrophys. Space Sci., 365(7), 110. https://doi.org/10.1007/s10509-020-03828-z
|
Şentürk, E., Adil, M. A., and Saqib, M. (2021). Ionospheric total electron content response to annular solar eclipse on June 21, 2020. Adv. Space Res., 67(6), 1937–1947. https://doi.org/10.1016/j.asr.2020.12.024
|
Stankov, S. M., Stegen, K., and Warnant, R. (2010). Seasonal variations of storm-time TEC at European middle latitudes. Adv. Space Res., 46(10), 1318–1325. https://doi.org/10.1016/j.asr.2010.07.017
|
Tang, R. X., Zeng, F. T., Chen, Z., Wang, J. S., Huang, C. M., and Wu, Z. P. (2020). The comparison of predicting storm-time ionospheric TEC by three methods: ARIMA, LSTM, and Seq2Seq. Atmosphere, 11(4), 316. https://doi.org/10.3390/atmos11040316
|
Thomas, D., Bagiya, M. S., Sunil, P. S., Rolland, L., Sunil, A. S., Mikesell, T. D., Nayak, S., Mangalampalli, S., and Ramesh, D. S. (2018). Revelation of early detection of co-seismic ionospheric perturbations in GPS-TEC from realistic modelling approach: Case study. Sci. Rep., 8, 12105. https://doi.org/10.1038/s41598-018-30476-9
|
Wang, C., Shi, C., Zhang, H. P., and Fan, L. (2016). Improvement of global ionospheric VTEC maps using the IRI 2012 ionospheric empirical model. J. Atmos. Solar–Terr. Phys., 146, 186–193. https://doi.org/10.1016/j.jastp.2016.05.014
|
Wang, H., and Zhang, K. D. (2017). Longitudinal structure in electron density at mid-latitudes: Upward-propagating tidal effects. Earth, Planets Space, 69(1), 11. https://doi.org/10.1186/s40623-016-0596-9
|
Wang, L., Li, Z. S., Ge, M. R., Neitzel, F., Wang, Z. Y., and Yuan, H. (2018). Validation and assessment of multi-GNSS real-time Precise Point Positioning in simulated kinematic mode using IGS real-time service. Remote Sens., 10(2), 337. https://doi.org/10.3390/rs10020337
|
Yang, J. L., Li, S., Yang, Z. C., Yang, H. L., Zhao, J. B., and Liu, L. (2023). Assessment and analysis of global DOP and positioning performance for BDS-3 based on iGMAS and IGS. In 2023 3rd International Conference on Frontiers of Electronics, Information and Computation Technologies (ICFEICT) (pp. 283–287). Yangzhou: IEEE. https://doi.org/10.1109/ICFEICT59519.2023.00055
|
Zhang, Q., and Zhao, Q. L. (2019). Evaluation and analysis of the global ionosphere maps from Wuhan University IGS Ionosphere Associate Analysis Center. Chinese J. Geophys. (in Chinese), 62(12), 4493–4505. https://doi.org/10.6038/cjg2019N0021
|
Zhang, S. R., Foster, J. C., Coster, A. J., and Erickson, P. J. (2011). East–West Coast differences in total electron content over the continental US. Geophys. Res. Lett., 38(19), L19101. https://doi.org/10.1029/2011GL049116
|
Zhu, W., Chen, J. Y., Zhang, Q., and Zhang, J. M. (2020). Mapping of high-spatial-resolution three-dimensional electron density by combing of full-polarimetric SAR and IRI model. Front. Earth Sci., 8, 181. https://doi.org/10.3389/feart.2020.00181
|
Ihsan Naufal Muafiry, Prayitno Abadi, Teguh N. Pratama, Dyah R. Martiningrum, Sri Ekawati, Yuandhika G. Wismaya, Febrylian F. Chabibi, Gatot H. Pramono. 2025: 3D tomographic analysis of equatorial plasma bubble using GNSS-TEC data from Indonesian GNSS Network. Earth and Planetary Physics, 9(1): 127-136. DOI: 10.26464/epp2024075 | |
Prayitno Abadi, Ihsan N. Muafiry, Teguh N. Pratama, Angga Y. Putra, Suraina, Gatot H. Pramono, Sidik T. Wibowo, Febrylian F. Chabibi, Umar A. Ahmad, Wildan P. Tresna, Asnawi. 2025: Leveraging ROTI map derived from Indonesian GNSS receiver network for advancing study of Equatorial Plasma Bubble in Southeast/East Asia. Earth and Planetary Physics, 9(1): 101-116. DOI: 10.26464/epp2025007 | |
Xiaowen Yuan, Yuzhen Wang, Yang Li, Yuhao Liu, Weiling Xu, Lizi Wang, Ruihan Deng. 2025: Impacts of ENSO on Wintertime Total Column Ozone over the Tibetan Plateau Based on the Historical Simulations of Community Earth System Model. Earth and Planetary Physics, 9(0). DOI: 10.26464/epp2024080 | |
Yang Lin, JianYong Lu, BaoHang Qu, Xi Wang. 2024: Assessing the performance of magnetopause models based on THEMIS data. Earth and Planetary Physics, 8(5): 776-786. DOI: 10.26464/epp2024053 | |
Yuan Lei, Xing Cao, BinBin Ni, Song Fu, TaoRong Luo, XiaoYu Wang. 2023: A data assimilation-based forecast model of outer radiation belt electron fluxes. Earth and Planetary Physics, 7(6): 620-630. DOI: 10.26464/epp2023079 | |
Aiza Amjad, Muhammad Shahid Riaz, Urooj Shakir, Muhammad Younis Khan, Muhammad Ali Shah. 2023: Evolution of Coulomb failure stress in Sulaiman Lobe, Pakistan, and its implications for seismic hazard assessment. Earth and Planetary Physics, 7(2): 282-294. DOI: 10.26464/epp2023034 | |
HongLin Jin, Yuan Gao, XiaoNing Su, GuangYu Fu. 2019: Contemporary crustal tectonic movement in the southern Sichuan-Yunnan block based on dense GPS observation data. Earth and Planetary Physics, 3(1): 53-61. DOI: 10.26464/epp2019006 | |
Liang Chen, Ming Ou, YaPing Yuan, Fang Sun, Xiao Yu, WeiMin Zhen. 2018: Preliminary observation results of the Coherent Beacon System onboard the China Seismo-Electromagnetic Satellite-1. Earth and Planetary Physics, 2(6): 505-514. DOI: 10.26464/epp2018049 | |
Qiao Wang, JianPing Huang, XueMin Zhang, XuHui Shen, ShiGeng Yuan, Li Zeng, JinBin Cao. 2018: China Seismo-Electromagnetic Satellite search coil magnetometer data and initial results. Earth and Planetary Physics, 2(6): 462-468. DOI: 10.26464/epp2018044 | |
JianPing Huang, XuHui Shen, XueMin Zhang, HengXin Lu, Qiao Tan, Qiao Wang, Rui Yan, Wei Chu, YanYan Yang, DaPeng Liu, Song Xu. 2018: Application system and data description of the China Seismo-Electromagnetic Satellite. Earth and Planetary Physics, 2(6): 444-454. DOI: 10.26464/epp2018042 |
1. | Zhang, S., Yang, Y., Xie, Y. et al. GNSS Signal Extraction Using CEEMDAN–WPD for Deformation Monitoring of Ropeway Pillars. Remote Sensing, 2025, 17(2): 224. DOI:10.3390/rs17020224 | |
2. | Liu, H., Lei, D., Yuan, J. et al. Ionospheric TEC prediction based on Attention-LSTM | [基于注意力机制 LSTM 的电离层 TEC 预测]. Acta Geophysica Sinica, 2024, 67(2): 439-451. DOI:10.6038/cjg2022Q0603 |