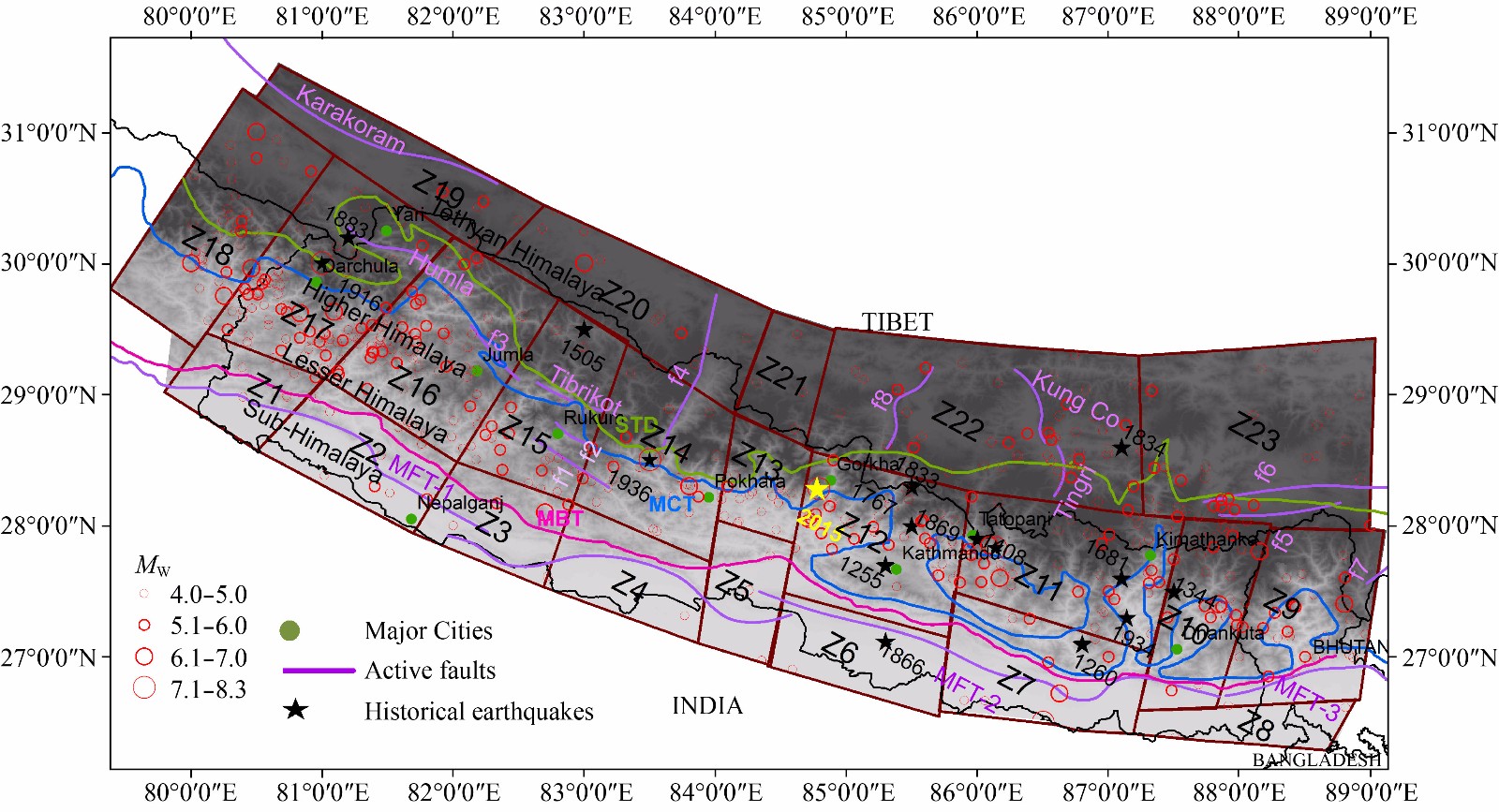
Citation: | Md Moklesur Rahman, Ling Bai. 2018: Probabilistic seismic hazard assessment of Nepal using multiple seismic source models. Earth and Planetary Physics, 2(4): 327-341. DOI: 10.26464/epp2018030 |
The potential for devastating earthquakes in the Himalayan orogeny has long been recognized. The 2015 MW7.8 Gorkha, Nepal earthquake has heightened the likelihood that major earthquakes will occur along this orogenic belt in the future. Reliable seismic hazard assessment is a critical element in development of policy for seismic hazard mitigation and risk reduction. In this study, we conduct probabilistic seismic hazard assessment using three different seismogenic source models (smoothed gridded, linear, and areal sources) based on the complicated tectonics of the study area. Two sets of ground motion prediction equations are combined in a standard logic tree by taking into account the epistemic uncertainties in hazard estimation. Long-term slip rates and paleoseismic records are also incorporated in the linear source model. Peak ground acceleration and spectral acceleration at 0.2 s and 1.0 s for 2% and 10% probabilities of exceedance in 50 years are estimated. The resulting maps show significant spatial variation in seismic hazard levels. The region of the Lesser Himalaya is found to have high seismic hazard potential. Along the Main Himalayan Thrust from east to west beneath the Main Central Thrust, large earthquakes have occurred regularly in history; hazard values in this region are found to be higher than those shown on existing hazard maps. In essence, the combination of long span earthquake catalogs and multiple seismogenic source models gives improved seismic hazard constraints in Nepal.
Due to the ongoing collision between the Indian and Eurasian plates since ~65 Ma (Ding L et al., 2005), the Himalayan mountain range has grown into the highest orogenic belt on Earth, experiencing widespread seismicity. Situated in the central segment of the Himalayan orogenic belt, Nepal is one of the most earthquake-prone countries in the world. The classical tectonic divisions of this region from south to north are the Sub-Himalaya, Lesser Himalaya, Higher Himalaya, and Tethyan Himalaya (Yin A, 2006), which are separated by four major E-W trending faults: the Main Frontal Thrust (MFT); the Main Boundary Thrust (MBT); the Main Central Thrust (MCT); and the South Tibetan Detachment System (STDS) (Figure 1). These surface faults have merged with the Main Himalayan Thrust (MHT), the detachment that separates the underthrusting Indian plate from the overriding Himalayan orogeny (Nábělek et al., 2009). The convergence rate between the Indian and Eurasian plates is 30–40 mm/year towards the NNE (Stevens and Avouac, 2015). About half of this rate is accumulating along the MHT (Bettinelli et al., 2006).
The Himalayan orogen has experienced many devastating earthquakes (Table 1), including the 2015 MW7.8 Gorkha, Nepal earthquake. Geodetic measurements (e.g., Stevens and Avouac, 2015) have identified that the MHT is fully locked at the shallower portion in its interseismic period and the accumulation process of the seismic strain continues until being released by large earthquakes (Bollinger et al., 2016; Rajendran et al., 2015; Sapkota et al., 2013). Previous studies have predicted that an earthquake of MW8.0 at 100 km east to the Kathmandu valley could potentially harm ~1.3% of the population and damage 50% of the buildings (Wyss, 2005). The 2015 MW7.8 Gorkha earthquake caused about 9000 casualties and destroyed over fifty thousands of buildings with huge economic losses (Bilham, 2015). However, the Gorkha Earthquake was considered to be smaller than the great earthquakes anticipated in the central Nepal (Bilham, 2015; Hand and Pulla, 2015) and eventually heightened the likelihood of catastrophic future earthquakes along the Himalayan orogenic belt.
Probabilistic seismic hazard assessment (PSHA) has long been employed in determining appropriate building design code provisions by many earthquake-resistant infrastructure projects (e.g., IBC, 2006). The implementation of seismic design provisions for buildings and other infrastructure is an effective way to reduce seismic risk and consequently to avoid earthquake-related disasters. After the pioneer work of Cornell (1968), PSHA has been widely studied on global, regional, and local scales (e.g., Bhatia et al., 1999; Chaulagain et al., 2015; Nath and Thingbaijam, 2012; Ordaz et al., 2014; Ram and Wang GX, 2013; Sawires et al., 2016; Zhang PZ et al., 1999). Earthquake catalogs (both instrumental and historical) are the primarily basis for PSHA studies. However, the complete part of the instrumental earthquake catalog is very short (e.g., around 55–60 years for this study region) compared to the time required for earthquake generation by tectonic processes (Bilham, 2013). This catalog limitation in PSHA causes low hazard value at some regions where great earhquakes have actually occurred (Kijko et al., 2016). This limitation is evident from some cases of big earthqukes, such as the 2008 Sichuan MW7.9, the 2010 Haiti MW7.0, and 2011 Japan MW9.0 earthquakes (Wang ZM et al., 2012). In this study therefore we accumulate all available historical and instrumental earthquake catalogs to assess the probabilistic seismic hazard for this area.
Seismic hazard maps for Nepal have been produced since the 1990’s (e.g., Chaulagain et al., 2015; Rahman et al., 2018; Ram and Wang GX, 2013; Zhang ZM et al., 1999). Most of these previous studies estimated the hazard value using only the complete part of the catalogs (about 60 years of data), based on single seismic source models and old attenuation equations. A recent study of the GEM Faulted Earth Project characterized the attribute of the Himalayan Frontal Thrust (MFT) fault in the seismic hazard assessment for the frontal Himalaya (Berryman et al., 2014). In this study, we use a methodology developed by Kijko and Smith (2012) to compute seismicity parameters (e.g., Gutenberg-Richter b value, rate of seismicity). In general, PSHA procedures assume that seismicity parameters are constant over time. But study of earthquake catalogs has shown that these parameters may change over time (Kijko et al., 2016). Thus we calculate the b value and seismicity rate by taking into account the incompleteness of the earthquake catalog since the 12th century. Moreover, three seismogenic source models (i.e. the smoothed gridded seismicity, the linear source model, and the areal source model) and two sets of ground motion prediction equations (GMPEs) are combined using a standard logic tree structure for capturing model-related epistemic uncertainties. The topographic elevation in the areal source and smoothed gridded seismicity models are also taken into account, while paleoseismic and geodetic records are incorporated into the linear source model. A set of seismic hazard maps for peak ground acceleration (PGA) and spectral acceleration (SA) are produced in this analysis for a better understanding of the spatial variation of seismic hazard.
Earthquake catalog provides the basic data for delineating seismogenic sources and computing the seismicity parameter, especially the mean seismic activity rate λ, the Gutenberg-Richter b value, and the maximum expected earthquake magnitude Mmax. We consider the incompleteness of the earthquake catalog which includes both historical earthquakes and the instrumental catalog, with different levels of completeness (Kijko and Smit, 2012; Kijko et al., 2016). Ambraseys and Douglas (2004) and Szeliga et al. (2010) provide information regarding historical major earthquakes for Himalaya and adjacent regions. We also use the historical earthquake catalogs from the National Oceanic and Atmospheric Administration (NOAA), GEM Global Historical Earthquake Archive (GEM-GHEA), and the National Seismological Centre of Nepal (NSC). Instrumental earthquake catalogs between 1906 and 2016 are collected from the U.S. Geological Survey (USGS) and International Seismological Centre (ISC).
Date | Latitude (°N) | Longitude (°E) | Location | Magnitude (MW) | Reference |
June 7, 1255 | 27.70 | 85.30 | Kathmandu | 7.7 | NOAA |
June 6, 1505 | 29.50 | 83.00 | Lo Mustang | 8.2 | A&D |
Jul. 25, 1720 | 30.00 | 80.00 | N Uttar-pradesh | 7.5 | A&D |
Aug. 26, 1833 | 27.70 | 85.70 | Nepal | 7.6 | A&D |
Oct. 14, 1911 | 31.00 | 80.50 | Tibet | 6.8 | ISC |
Aug. 28, 1916 | 29.90 | 80.50 | Uttaranchal | 7.2 | A&D |
Jan. 15, 1934 | 27.55 | 87.09 | Bihar-Nepal | 8.2 | S |
May 27, 1936 | 28.50 | 83.50 | Nepal | 7.0 | ISC |
June 4, 1945 | 30.00 | 80.00 | Nepal | 6.5 | ISC |
Aug. 15, 1950 | 28.70 | 96.70 | Assam | 8.5 | A&D |
Sep. 4, 1954 | 28.30 | 83.80 | Nepal | 6.5 | ISC |
Sep. 20, 1988 | 26.72 | 86.63 | Nepal | 6.6 | ISC |
Sep. 18, 2011 | 27.81 | 88.20 | Sikkim, India | 6.9 | ISC |
April 25, 2015 | 28.28 | 84.79 | Gorkha, Nepal | 7.8 | ISC |
May 12, 2015 | 27.83 | 86.14 | Nepal | 7.1 | ISC |
Notes: NOAA: National Oceanic and Atmospheric Administration; A&D:Ambraseys and Douglas (2004); S: Sapkota et al. (2013); ISC: International Seismological Centre. |
The catalogs from different sources are then compiled together and checked manually to remove duplicate events on the basis of location, time, and magnitude. We convert the different types of magnitude (e.g., body wave magnitude, surface wave magnitude, duration magnitude, and local magnitude) contained in the catalog into MW using the different empirical relationships shown in the Table 2. Approximately 2100 events with MW≥4.0 are recorded in the comprehensive catalog. The seismicity model usually assumes that the occurrence of an earthquake is independent. All foreshocks and aftershocks are therefore excluded from the catalog, deploying a declustering process following the algorithm of Gardner and Knopoff (1974). A total of 900 events (including 13 historical earthquakes with magnitude equal to or greater than 6.0) are included in the final catalog (Figure 1).
(Scordilis, 2006) | (Grünthal and Wahlström, 2003) | (Kaviris et al., 2008) |
MW = 0.67 MS + 2.07 for 3.0≤MS≤6.1 | MW = 0.67(±0.11) + 0.56(±0.08) ML + 0.046(±0.013) ML2 | MW = MD + 0.5 (MD<3.0) |
MW = 0.99 MS + 0.08 for 6.2≤MS≤8.2 | MW = MD + 0.6 (MD≥3.0) | |
MW = 0.85 Mb + 1.03 for 3.5≤Mb≤6.2 | ||
Notes: MW: Moment magnitude; MS: Surface wave magnitude; ML: Local magnitude; Mb: Body wave magnitude; MD: Duration magnitude. |
Assessment of different levels of completeness of various sub-catalogs for the instrumental earthquake catalog is very important. The reason is that records of large earthquakes are generally complete for longer periods while the catalogs of smaller earthquake are complete for shorter durations. The completeness of each earthquake catalog is governed by such considerations of socioeconomic and historical circumstances, demographic variations, and seismic station coverage. Failure to acknowledge the incompleteness of the catalogs would result in overestimate and underestimate of recurrence rates for small and large earthquakes, respectively. We first assume a threshold magnitude (M0) of MW4.0 and then estimate completeness by following the visual cumulative method (Tinti and Mulargia, 1985). The modern instrumental catalog is found to be complete since 1964 (Figure 2a). In utilizing the incomplete instrumental earthquake catalog since 1906, the entire catalog is divided into different sub-catalogs based on different levels of completeness. The complete time window for various magnitude intervals (e.g., 4.0–5.0; 5.0–6.0; 6.0–7.0) is assessed using the statistical method of Stepp (1972). The complete time windows are found for different levels of completeness of 4.0, 5.0 and 6.0 to be after 1964, 1925–1964, and 1906–1925, respectively (Figure 2b).
Proper seismic source zoning is essential in PSHA. Seismogenic sources with different characteristics are generally delineated according to tectonic settings, seismological and geological attributes, and fault characteristics. The Himalayan orogen is regarded as a very complex earthquake producing environment. We thus use a model that combines three different seismic sources: areal, linear, and smoothed gridded sources.
In general, the area of a seismic source zone is delineated using the basic principle that seismicity within a single source zone is uniform and homogenous. It is therefore assumed that every point within the source has an equal potential to generate a future earthquake (Baker, 2015). Recent studies (Chaulagain et al., 2015; Ram and Wang GX, 2013) have delineated 23 areal seismic source zones (Figure 1) for the study region based on quantitative analysis of the earthquake distribution, fault information, and seismotectonic settings. In this study, the same (23) seismic areal source zones are utilized. High seismicity clusters are present in western (zones 16, 17 and 18) and east central (zones 9, 10, 11 and 12) Nepal. The frontal edge of the Himalaya along the boundary of southern Nepal shows sparse seismicity.
Usually, major earthquakes are associated with active faults, and in seismic hazard assessment these active faults are considered as linear seismic sources. Although, there are numerous methods for earthquake source simulation (e.g., Zhang WB et al., 2006), for the Himalayan-Tibetan region, Styron et al. (2010) identified active faults using remote sensing imagery, aerial photographic interpretation, paleoseismic studies, and field investigations. For our study region, 14 active faults are assigned as linear seismic sources (Figure 1). Apart from the MFT, there are no well-constrained attributes for the other faults. Therefore, two magnitude-area and magnitude-length scaling empirical relationships of Yen YT and Ma KF (2011) and Wells and Coppersmith (1994) are applied to characterize these faults’ attributes. The compilation and evaluation of approximately 72 such kinds of relationships were carried out in the GEM Faulted Earth and Regionalisation Components project (Stirling and Goded, 2012). They found that the relationship of Yen YT and Ma KF (2011) is better in quality because of its updated wide-spread datasets with greater magnitude coverage than that of Wells and Coppersmith (1994) who used older datasets and relatively narrow magnitude coverage. They recommended the quality score of 1 (best available) and 2 (good) for Yen YT and Ma KF (2011) and Wells and Coppersmith (1994), respectively. Accordingly, we assign the weight for Yen YT and Ma KF (2011) and Wells and Coppersmith (1994) as two-thirds and one-third, respectively. For large magnitude earthquakes, the occurrence rate along these faults is determined using long-term slip rates as:
Recurrence Interval=M0/μ˙uLW, | (1) |
where μ is shear modulus 3.0 × 1011 dyne/cm2, L is rupture length, W is rupture width,
log(M0)=1.5M+16.05. | (2) |
Small scale fault attributes (e.g., dip, dip direction, slip, and slip rate) are not well documented for this study region. We therefore assign to small-scale faults the same values for the above mentioned parameters as those of nearby known faults (MFT). The Main Frontal Thrust (MFT) is one of the well-constrained active faults in this study area (e.g., Ader et al., 2012). The GEM faulted earth project (Berryman et al., 2014) characterized the different attributes of the MFT for all of the three different segments in the study area. In this study, we used the same attributes (e.g., rup- ture length, width, dip, dip direction, slip, slip rate, and expected maximum magnitude) for the three segments of MFT (i.e. MFT-1, MFT-2, and MFT-3, respectively from west to east).
We deployed two magnitude-frequency distribution models in the linear source model: the Characteristic-earthquake model for the empirically estimated earthquakes with magnitude equal or higher than MW≥6.5, and the Gutenberg-Richter model for instrumentally recorded earthquakes. The computed linear source parameters (e.g., seismic moment, slip rates, rupture length, rupture) for active faults are illustrated in Table 3 for the Himalayan region.
As earthquake hypocenters are widely scattered in relation to fault locations, we use a zone-free approach for the spatial smoothing of seismicity (Frankel, 1995; Woo, 1996) to avoid subjectivity in delineating areal seismic sources. This approach is termed “kernel estimation”; it implements spatial smoothing of seismicity and depicts the seismicity’s non-uniform spatial distribution, by assuming that seismicity varies from place-to-place. For smoothed gridded seismicity, thousands of 0.1º × 0.1º grid cells are made for the entire study area. At each grid cell, earthquakes of MW≥4.0 are counted for different magnitude intervals in estimating the seismicity rates for corresponding magnitude intervals. The final seismic activity rate is then obtained by smoothing the calculated seismicity rates using the multivariate kernel probability density function following the equation of Woo (1996) as:
^ni=∑jnje−Δ2ij/c2∑je−Δ2ij/c2, | (3) |
where nj is the rate of seismicity in the j-th grid cell,
Fault Name | Length (km) | M0* | Width (km) | Slip rate (mm/year) | b | σb | λ |
MFT-1 | 1300 | 2.67888E+22 | 100 | 20.5 | 0.76 | 0.09 | 4.334 |
MFT-2 | 330 | 1.6133E+21 | 100 | 18.0 | 0.61 | 0.04 | 2.703 |
MFT-3 | 800 | 9.86865E+21 | 100 | 20.0 | 0.73 | 0.09 | 1.371 |
Tibrikot | 62 | 5.50155E+19 | 39 | 20.5 | 0.69 | 0.14 | 0.055 |
Humla | 76 | 8.27582E+19 | 47 | 20.5 | 0.61 | 0.13 | 0.370 |
Tingri | 44 | 2.77124E+19 | 30 | 18.0 | 0.51 | 0.1 | 0.233 |
Kung Co | 68 | 6.62049E+19 | 43 | 18.0 | 0.44 | 0.09 | 0.090 |
Karakoram | 300 | 1.3287E+21 | 110 | 10.0 | 0.63 | 0.09 | 0.460 |
f1 | 70 | 7.01679E+19 | 44 | 20.5 | 0.54 | 0.12 | 0.154 |
f2 | 55 | 4.32837E+19 | 36 | 20.5 | 0.55 | 0.22 | 0.048 |
f3 | 65 | 6.04792E+19 | 41 | 20.5 | 1.2 | 0.12 | 0.164 |
f4 | 139 | 2.79178E+20 | 76 | 20.5 | 0.69 | 0.16 | 0.425 |
f5 | 92 | 1.21496E+20 | 54 | 18.0 | 0.71 | 0.1 | 0.223 |
f6 | 179 | 4.65654E+20 | 93 | 18.0 | 0.63 | 0.1 | 0.148 |
f7 | 103 | 1.52508E+20 | 60 | 18.0 | 0.48 | 0.1 | 0.066 |
f8 | 82 | 9.64029E+19 | 50 | 18.0 | 0.54 | 0.21 | 0.102 |
Notes: Fault numbering (f1–f8) is chosen arbitrarily since no naming appears on the source files. Rupture width for MFT-1–MFT-3 is taken from Berryman et al. (2014); and for the rest of the faults is estimated using the relationship of Yen YT and Ma KF (2011). Slip rates are recorded from Ader et al. (2012). λ, b value, and σb are used for the Gutenberg-Richter seismicity model. M0* is the weighted seismic moment. |
The maximum expected earthquake magnitude (Mmax) for every seismic source zone is computed on the basis of historical (e.g., 1505 MW8.2 and 1934 MW8.2 earthquakes) and recent earthquake data, but also considering great earthquakes that occurred in nearby seismic zones with similar tectonic settings. The estimate of Mmax is computed following the algorithm of Kijko and Singh (2011). This estimation takes into account the uncertainty in the earthquake magnitude determination. The estimated maximum expected magnitude of an earthquake for each areal source zone, along with its standard deviation, is given in Table 4. The computed Mmax values vary between 6.5 and 8.6. For smoothed gridded seismicity, the same range of Mmax is used for the respective grids.
Accurate determination of earthquake location along the mountain belt is usually difficult because of insufficient station coverage. The ISC catalog reveals that earthquakes are distributed throughout the thickness of the Tibetan and Indian crust ranging between 0 and 90 km. Moreover, some recent studies have found that the hypocenters of most of the earthquakes in Nepal are located at depths ranging between 0 and 30 km (Pandey et al., 1999; Priestley et al., 2008). In addition, hypocenter relocation results from a nearby broadband seismic array found that the focal depths for the Gorkha earthquake sequence ranged primarily between 10 km and 20 km (Adhikari et al., 2015; Bai et al., 2016; Letort et al., 2016). Thus, in this analysis, the average focal depth of 15 km is assigned as a constant depth for all the source models. It is essential to note that the topographic elevation of the study area varies significantly from south to north. The elevation of the source zones along the south frontal border is less than 1 km and in the central and northern zones is about 3 km and 5 km, respectively. Seismic intensity attenuates reasonably with respect to distance from the source and thereby the topography has a considerable effect on hazard value. Thus, the topographic elevation is considered for each source zone along with the assigned average focal depth.
Zones | a-value | b-value | σb* | λ* | Mu* | σ(Mu)* |
Z1 | 4.48 | 0.85 | 0.20 | 0.086 | 6.5 | 0.86 |
Z2 | 4.16 | 0.77 | 0.18 | 0.115 | 6.5 | 0.57 |
Z3 | 3.40 | 0.70 | 0.33 | 0.013 | 6.5 | 0.49 |
Z4 | 4.22 | 0.82 | 0.12 | 0.060 | 6.5 | 0.29 |
Z5 | 4.10 | 0.82 | 0.12 | 0.033 | 6.5 | 0.47 |
Z6 | 4.84 | 0.82 | 0.12 | 0.020 | 7.2 | 0.86 |
Z7 | 3.22 | 0.47 | 0.20 | 0.033 | 7.5 | 0.72 |
Z8 | 4.84 | 0.82 | 0.12 | 0.019 | 7.7 | 1.00 |
Z9 | 4.04 | 0.70 | 0.07 | 0.157 | 8.1 | 0.30 |
Z10 | 3.75 | 0.56 | 0.05 | 0.137 | 8.5 | 0.46 |
Z11 | 4.23 | 0.60 | 0.06 | 0.240 | 8.5 | 0.45 |
Z12 | 4.16 | 0.53 | 0.04 | 0.240 | 8.5 | 0.70 |
Z13 | 4.46 | 0.80 | 0.17 | 0.187 | 7.0 | 0.34 |
Z14 | 4.31 | 0.66 | 0.11 | 0.198 | 8.0 | 0.93 |
Z15 | 4.10 | 0.66 | 0.17 | 0.138 | 8.5 | 0.43 |
Z16 | 4.40 | 0.68 | 0.06 | 0.493 | 8.5 | 0.56 |
Z17 | 4.67 | 0.64 | 0.05 | 0.482 | 8.6 | 0.51 |
Z18 | 4.63 | 0.69 | 0.08 | 0.243 | 8.5 | 0.31 |
Z19 | 3.98 | 0.71 | 0.14 | 0.068 | 6.5 | 0.36 |
Z20 | 4.48 | 0.77 | 0.15 | 0.081 | 7.0 | 0.44 |
Z21 | 4.96 | 0.82 | 0.12 | 0.080 | 6.5 | 0.36 |
Z22 | 4.51 | 0.63 | 0.09 | 0.362 | 6.5 | 0.30 |
Z23 | 4.38 | 0.64 | 0.09 | 0.201 | 6.6 | 0.30 |
Notes: σb* is the standard error of b value; λ* is the rate of seismicity per year; σ(Mu)* is the standard deviation of expected maximum magnitude (Mu*). |
To estimate the earthquake magnitude exceedance rates, a Modified Gutenberg-Richter (MGR)-Poisson model is chosen as a seismicity model for all seismogenetic sources except characteristic earthquakes. Herein, the seismicity is expressed as (Cornell and Van Marke, 1969):
λ(M)=λ0exp(−βM)−exp(−βMmax | (4) |
where λ0 is the rate of earthquake activity with threshold magnitude M0 (here M0 = 4.0), β is a parameter equivalent to the b value (β = 2.303×b), and Mmax is the maximum expected magnitude for the sources. In computing the λ(M) value, the uncertainty of both Mmax and β are taken into account.
Conventionally, seismicity parameters are calculated based on the minimum magnitude of completeness (MC) of the catalog. The Maximum Curvature (MAXC) method of Wiemer and Wyss (2000) is used to evaluate the MC value. The estimated MC value of MW4.0 is taken as the M0 because earthquakes smaller than MW4.0 are not likely to cause any damage to infrastructure, from an engineering point of view.
In this study, the essential seismicity parameters are computed by taking into account the incompleteness of the instrumental catalog and the historical earthquake records. The Gutenberg-Richter b value and its standard error for every source zone are determined following the joint maximum likelihood function of Kijko and Smit (2012) based on the historical and instrumental earthquake catalog. This new approach is the extension of the Aki-Utsu (Aki, 1965; Utsu, 1965) b value estimator which takes into account both incompleteness of the earthquake catalogs and temporal variation of seismicity. It provides straightforward approximations for the standard errors and confidence intervals (Kijko and Smit, 2012). In this method, the whole span of the earthquake catalog is divided into different sub-catalogs and the parameter, β (β = 2.303×b) is calculated as:
L\left( \beta \right) = \mathop \prod \limits_{i = 1}^s \mathop \prod \limits_{j = 1}^{{n_i}} f\left( {m_j^i,\beta } \right) = \mathop \prod \limits_{i = 1}^s \mathop \prod \limits_{j = 1}^{{n_i}} \beta \exp\left[ { - \beta \left( {m_j^i - m_{\min}^i} \right)} \right], | (5) |
where mji is the sample of ni earthquake magnitudes recorded during the time span of the i-th sub-catalog (i=1, 2, …, s). In addition, maximization of the likelihood function in equation (5) provides the generalized Aki–Utsu
\hat \beta = {\left( {\frac{{{r_1}}}{{{{\hat \beta }_1}}} + \frac{{{r_2}}}{{{{\hat \beta }_2}}} + \ldots + \frac{{{r_s}}}{{{{\hat \beta }_s}}}} \right)^{ - 1}}, | (6) |
where
However, in this analysis, the b value and its standard errors are estimated for the whole region, as well as for each areal source zone. For the data of the whole region, the estimated b value is 0.82 and the standard error is 0.12. These values are assigned to zones 4, 5, 6, 8, and 21 because of low seismicity. On the other hand, for rest of the zones, the individual b value and its standard error are assigned for hazard computation (Table 4). In case of smoothed gridded sources, the b value and its standard error of 0.82 and 0.12 are employed to all of the grids.
Once the
\hat \lambda \left( {{m_{\min}}} \right) = \frac{n}{{\displaystyle \sum \nolimits_{i = 1}^s {t_i}\exp\left[ { - \hat \beta \left( {m_{\min}^i - {m_{\min}}} \right)} \right]}}. | (7) |
The ground motion prediction equations (GMPEs), also known as attenuation relationships, are essential in estimating the ground motion parameters (e.g., PGA, SA). These equations usually give ground motion intensity as a function of many variables such as earthquake magnitude, source-to-site distance, and soil condition. In general, the attenuation relationships are obtained empirically using a statistical regression method on a particular set of strong motion data.
The seismotectonic setting of the Himalayan orogenic belt is very complex. This study area is a tectonically active interplate region (Nath and Thingbaijam, 2012) and the earthquake occurrence pattern of this region is not simple and even rather compound in combination. For example, Nath and Thingbaijam (2012) identified that this study region belongs to the active shallow crustal regime (ACR) with many subduction zone interface (SZI) earthquakes across the Himalaya. Earthquake focal depth and mechanism study (Bai et al., 2017) stated that the occurrence of large earthquakes is fairly associated with subduction interface in this region. Moreover, it was assumed by some previous seismic hazard studies that the Himalaya and its surrounding region belongs to either SIZ or ACR (e.g., Ram and Wang GX, 2013), or both (e.g., Chaulagain et al., 2015). In avoiding the controversy, we consider that the study region behaves as both of these environments. However, the strong ground motion data for the Tibetan-Himalayan region are insufficient and consequently no particular GMPEs have been studied previously.
Usually, this problem is resolved by selecting the GMPEs developed in other regions of similar seismotectonic settings, which could represent sufficiently the ground motion in this region. Therefore, a total of 8 GMPEs are selected in this analysis according to the criteria (e.g., tectonic regime, functional form, regression coefficients, frequency range of the model, journal types, datasets, etc.) of Cotton et al. (2006), and a number of trellis plots (i.e. predicted spectral acceleration (PSA) versus magnitude, PSA versus distance, PSA versus structural periods) as Stewart et al. (2013). Two Next Generation Attenuation (NGA-west2) models: Abrahamson et al. (2014) and Chiou and Youngs (2014) from the global datasets; one model from Europe and Middle East datasets of Ambraseys et al. (2005); and other one from mainly Japanese datasets of Zhao JX et al. (2006) are selected for ACR. The weight assessment and thereby the ranking of different GMPEs are not carried out because of scarcity of strong motion records in the Himalayan-Tibetan region. Due to insufficient observed data, many seismic hazard studies for Asian countries (e.g., Chaulagain et al., 2015; Kolathayar and Sitharam, 2012; Nath and Thingbaijam, 2012; Ornthammarath et al., 2011) used equal weight for all models. In this study, therefore, for each of these four models, equal probabilistic weights (1/4) are assigned. Besides, three models from global datasets covering the large distance, namely BC Hydro 2016 known as Abrahamson et al. (2016), Atkinson and Boore (2003), and Youngs et al., (1997); and one for local dataset of Zhao JX et al. (2006) are selected for SIZ. Equal probabilities (1/4) of weight to each of the models are assigned, as for ACR.
Compatibility among these selected GMPEs is checked when applied in the CRISIS2015 (Ordaz et al., 2015) program. Finally, these two sets of GMPEs are combined together using a logic tree. In the similar way, GMPEs are employed for all three source models.
Uncertainties are commonly associated with different models used in PSHA. The application of a logic tree allows the capturing of epistemic uncertainties in different input models (Bommer et al., 2005; Sabetta et al., 2005) by deploying alternative models in the hazard estimation. In this study, three types of seismogenic source models and two sets of GMPEs (each consisting of four different GMPEs) are combined using the logic tree. Equal weights (1/3) are assigned to each of the source models, as there is no substantial observed data for the study region in evaluating the ranking of the weight. For the linear source model, equal weight (1/2) is assigned for the Characteristic Earthquake and Gutenberg-Richter seismicity models as there is no reason to prefer one model over the other. Figure 3 displays the standard logic tree framework used for the linear source model in this analysis.
To estimate the seismic hazards on the basis of computed seismicity parameters, the seismic hazard module CRISIS2015 (Ordaz et al., 2015) is used. This module has high efficiency in hazard calculation and flexibility in model selection (Danciu et al., 2010). For areal and linear seismic sources, the principle of CRISIS in computing the seismic hazard is based on the standard Cornell approach (Cornell, 1968). The assumption of the Module is that within a source zone, seismicity is evenly distributed by unit area and thereby all the points might be a potential earthquake focus. A spatial integration by subdividing the original sources is performed by the CRISIS program in obtaining accurate hazard value. Once a source is subdivided into sub-sources, the acceleration exceedance rate due to the i-th single source can be computed using the equation:
{\nu _{i\left( a \right)}} = \mathop \sum \limits_j {W_{ij}}\mathop\int _{{M_0}}^{M_{\max}} \left( {\frac{{ - {\rm d}\lambda \left( M \right)}}{{{\rm d} M}}} \right)Pr\left( {A > a\big| M,{R_{ij}}} \right){\rm d}M, | (8) |
where M0 and Mmax are the threshold and maximum magnitudes, respectively;
\nu (a) = \sum\nolimits_{(n = 1)}^N {\int_{{M_0}}^{{M_{\max }}} {\left( {\frac{{ -{\rm d}\lambda \left( M \right)}}{{{\rm d}M}}} \right)} } Pr(A > a\left| {M,{R_{ij}}} \right.){\rm d}M. | (9) |
For smoothed gridded seismicity, once the seismicity parameters and the GMPEs are known for each of the nodes of the grids, the hazard at a given node is calculated based on the effects of the totality of the nodes and their corresponding distances to the site of interest as in equations (8) and (9).
For seismic hazard estimation, the entire study region is divided into small grids with the size of 0.1º×0.1º and the total number of grid cells is approximately 5000. At the center of each grid cell, the PGA and SA values are computed for a referenced bedrock condition. The contribution of all the potential sources to hazard value within the radial distance of 300 km from the center of each grid cell is taken into account. We estimate the hazard value by disaggregating the hypocentral distance into small intervals of 1 km, and the magnitude range (between the minimum and maximum magnitude) into small incremental values of 0.1. Initially, the hazard value at each grid cell is computed using all of the eight different attenuation models for all three seismogenic sources separately. Afterward, the final hazard value at each grid cell is obtained by the combination of these individual values, using the logic tree structure. This analysis produces different seismic hazard maps for PGA value and SA value at short (0.2 s) and long (1.0 s) natural periods at 5% critical damping factor for 10% and 2% probabilities of exceedance in 50 years, corresponding to 475 and 2,475 year return periods, respectively. PGA hazard maps for the probabilities of exceedance of 10% and 2% in 50 years are shown in Figure 4a, b. Figure 5a, b illustrates the spatial variations of SA value obtained at 0.2 s and 1.0 s natural periods for 2% probability of exceedance.
The overall spatial distribution of hazard value regarding both PGA and SA is non-uniform. Because of heterogeneous characteristics of the seismotectonic settings at the study region, there are clear lateral variations in the computed seismic hazard maps. The predicted hazard distribution pattern largely follows the background seismicity distribution across the study area. The regions of highest potential hazard are likely distributed along strike of the Indian-Eurasian plate collision zones. However, the hazard estimate for 10% probability of exceedance shows a wide range of values, and the minimum and maximum hazard values are found as low as 0.21g, and as high as 0.64g, respectively (Figure 4a). The zones of highest hazard are identified in the far western and central to northeast central Nepal (Figure 4a) where the rate of seismicity is very high compared to other parts of Nepal (Figure 1). For far western Nepal (i.e. south of Darchula city), we obtain peak hazard values as high as 0.64g. In central Nepal around the Kathmandu valley, peak hazard values range up to 0.59g. The southern-most part along the Nepal-India boundary is identified as a low seismic hazard zone. MHT has long been predicted as a potential source for producing great earthquakes in the Himalayan region (Avouac et al., 2015; Rajendran et al., 2015; Sapkota et al., 2013). Large earthquakes in the central portion of the Himalayan orogenic belt may greatly affect the densely-populated cities of Nepal, such as Kathmandu and Pokhara (Bollinger et al., 2016).
Nonetheless, the predicted seismic hazard value is increased significantly with decreasing probability of exceedance. For example, at 2% probability of exceedance in 50 years, the computed hazard level for central and northeast central Nepal is about a factor of 1.8 higher than that of 10% in 50 years; for far western Nepal, the comparable factor is nearly 1.5 (Figure 4b). The maximum and minimum PGA value for this case is obtained as low as 0.40g and as high as 1.02g, respectively. We produce the SA maps (Figure 5a, b) as per the requirement of the modern building design provisions, which are analogous with the PGA maps in terms of hazard distribution pattern. SA value at 0.2 s and 1.0 s natural periods for 2% probability of exceedance in 50 years is found in the order of 0.87–2.38g, and 0.29–1.19g, respectively.
To understand how different source models contribute to the computed seismic hazard values, we estimate the hazard value for each model individually. The resulting hazard maps for these source models show that the levels of maximum and minimum hazard value are quite different from model to model. For instance, the linear source model produces maximum values as high as 0.60g whereas the maximum hazard values for the smoothed gridded and areal source models are 0.71g and 0.54g, respectively. We also find that the effect of sources on hazard value varies spatially. In regions with high hazard, all the source models (areal, smoothed gridded, and linear) produce nearly similar ground motion values (Figure 6). However, for northern-most, southern-most, and the central west regions where background seismicity is low, different levels of effect are observed among the models. For example, in the southern and central west regions of Nepal (e.g., around the cities of Bara, and Rukum), the linear source model contributes comparatively higher seismic hazards than the other two source models, and consequently augments the combined hazard value levels for these regions (Figure 6). On the other hand, in northern Nepal (near Gorkha city), the contribution from areal and smoothed gridded sources is comparatively higher than that of the linear source model. In the southwest region (near Kailali city), the highest contribution is from the smoothed seismicity source, and the areal source contributes the least hazard value, while the linear source model yields an intermediate effect. Moreover, to infer the attenuation pattern of seismic hazard with respect to various natural periods, the uniform seismic hazard spectra at 10% probability of exceedance in 50 years for different cities is computed (Figure 7). The highest hazard value is obtained at the short natural period of 0.2 s for all cities. With the increase of the natural periods, the hazard value falls rapidly, and at the longer periods of about 1.5 s and above, the values became nearly flattened and exhibit very gentle slopes of attenuation.
To produce seismic hazard maps of Nepal, we incorporate the latest datasets, multiple seismogenic source considerations, updated GMPEs, topographic elevation data, well constrained focal depth data, and consideration of inherent uncertainties associated with each of these inputs. An updated uniform declustered earthquake catalog since 12th century is utilized. 23 areal sources, 14 linear sources and hundreds of smoothed gridded sources are combined using a logic tree to account for the epistemic uncertainties. Essential seismicity parameters (e.g., b value and rate of seismicity, λ) are computed using the joint maximum likelihood method (Kijko and Smit, 2012). The incompleteness of the catalog (i.e. different complete sub-catalogs) and the uncertainty in earthquake magnitude determination are considered for seismicity parameters computation. The modified Gutenberg-Richter and Characteristic-earthquake magnitude-frequency models, two sets of GMPEs (four GMPEs for the active shallow crust and four GMPEs for the subduction interface) are utilized. In essence, the combination of past seismicity data (both historical and instrumental), paleoseismic and geodetic data, along with three sesimogenic source models, thus provides more comprehensive and explainable constraints to the computation of seismic hazard values.
The spatial variation pattern of the hazard value shows good agreement with the maps of Zhang PZ et al. (1999), Ram and Wang GX (2013), and Rahman et al. (2018). In addition, the resulting hazard values for the Nepal area are comparable with results for the adjacent regions in India (e.g., Kolathayar and Sitharam, 2012; Nath and Thingbaijam, 2012) and Tibet (Rahman et al., 2018). The rock-site PGA value around the epicenter of the 2015 Gorkha earthquake shown in previous studies (e.g., Chaulagain et al., 2015; Ram and Wang GX, 2013; Zhang PZ et al., 1999) is below 0.4g. The hazard value for the source area of the Gorkha earthquake has been estimated to be low (Chaulagain et al., 2015; Ram and Wang GX, 2013) based on the single source model and the short span earthquake catalog. However, the PGA values obtained in this study are up to 0.45g at the same probability of exceedance (i.e. 10%). We suggest that this difference is probably a result of our consideration of multiple seismogenic sources along with the wide span of our earthquake catalog including historic large earthquakes.
The hazard value is assessed in terms of PGA for 10% and 2% probabilities of exceedance on bedrock level. The spectral acceleration distribution maps at 0.2 s and 1.0 s natural periods are produced for 2% probability of exceedance in 50 years. The region of the Lesser Himalaya from east to west is found to be has high seismic hazard potential. The seismic hazards estimated in this study would help constrain the national building design provisions for the central segment of the Himalayan region. Better seismic hazard estimate in a tectonically active region requires detail paleoseismic, geological, and geodetics studies. Strong ground motion data is essential in order to develop the new attenuation relationships for this tectonically active region.
This research is supported by the grants of the National Nature Science Foundation of China (No. 41761144076, 41490611), the collaborative research program of the Disaster Prevention Research Institute of Kyoto University (No. 29W-03) and the COX visiting professor fellowship of the Stanford University to L.B., and by the Chinese Academy of Sciences (CAS) and The World Academy of Sciences (TWAS) President’s PhD Fellowship to M.M.R.. We used earthquake catalogs from the ISC, USGS, GEM, NOAA and NSC. We are grateful to Andrzej Kijko and his colleagues for providing the “AUE” program, to Mario Ordaz for providing us the recently developed version of the CRISIS software, and to Jack Baker and Fabrice Cotton for useful discussion. We thank the editor Xiaodong Song, Wenbo Zhang and an anonymous reviewer for their constructive comments.
Abrahamson, N. A., Silva, W. J., and Kamai, R. (2014). Summary of the ASK14 ground motion relation for active crustal regions. Earthq. Spectra, 30(3), 1025–1055. https://doi.org/10.1193/070913EQS198M
|
Abrahamson, N., Gregor, N., and Addo, K. (2016). BC hydro ground motion prediction equations for subduction earthquakes. Earthq. Spectra, 32(1), 23–44. https://doi.org/10.1193/051712EQS188MR
|
Ader, T., Avouac, J. P., Liu-Zeng, J., Lyon-Caen, H., Bollinger, L., Galetzka, J., Genrich, J., Thomas, M., Chanard, K., … Flouzat, M. (2012). Convergence rate across the Nepal Himalaya and interseismic coupling on the Main Himalayan Thrust: Implications for seismic hazard. J. Geophys. Res.: Solid Earth, 117(B4), B04403. https://doi.org/10.1029/2011JB009071
|
Adhikari, L. B., Gautam, U. P., Koirala, B. P., Bhattarai, M., Kandel, T., Gupta, R. M., Timsina, C., Maharajan, N., Maharajan, K., … Bollinger, L. (2015). The aftershock sequence of the 2015 April 25 Gorkha-Nepal earthquake. Geophys. J. Int., 203(3), 2119–2124. https://doi.org/10.1093/gji/ggv412
|
Aki, K. (1965). Maximum likelihood estimate of b in the formula logN=a−bM and its confidence limits. Bull. Earthq. Res. Inst., 43(2), 237–239.
|
Ambraseys, N. N., Douglas, J., Sarma, S. K., and Smit, P. M. (2005). Equations for the estimation of strong ground motions from shallow crustal earthquakes using data from Europe and the middle east: horizontal peak ground acceleration and spectral acceleration. Bull. Earthq. Eng., 3(1), 1–53. https://doi.org/10.1007/s10518-005-0183-0
|
Ambraseys, N. N., and Douglas, J. (2004). Magnitude calibration of north Indian earthquakes. Geophys. J. Int., 159(1), 165–206. https://doi.org/10.1111/j.1365-246X.2004.02323.x
|
Atkinson, G. M., and Boore, D. M. (2003). Empirical ground-motion relations for subduction-zone earthquakes and their application to Cascadia and other regions. Bull. Seismol. Soc. Am., 93(4), 1703–1729. https://doi.org/10.1785/0120020156
|
Avouac, J.-P., Meng, L. S., Wei, S. J., Wang, T., and Ampuero, J.-P. (2015). Lower edge of locked Main Himalayan Thrust unzipped by the 2015 Gorkha earthquake. Nat. Geosci., 8(9), 708–711. https://doi.org/10.1038/ngeo2518
|
Bai, L., Liu, H. B., Ritsema, J., Mori, J., Zhang, T. Z., Ishikawa, Y., and Li, G. H. (2016). Faulting structure above the Main Himalayan Thrust as shown by relocated aftershocks of the 2015 Mw7.8 Gorkha, Nepal, earthquake. Geophys. Res. Lett., 43(2), 637–642. https://doi.org/10.1002/2015GL066473
|
Bai, L., Li, G. H., Khan, N. G., Zhao, J. M., and Ding, L. (2017). Focal depths and mechanisms of shallow earthquakes in the Himalayan–Tibetan region. Gondwana Res., 41, 390–399. https://doi.org/10.1016/j.gr.2015.07.009
|
Baker, J. W. (2015). Introduction to Probabilistic Seismic Hazard Analysis. White Paper Version 2.1.
|
Berryman, K., Ries, W., and Litchfield, N. (2014). The Himalayan Frontal Thrust: Attributes for seismic hazard Version 1.0. GEM Faulted Earth Project, available from http://www.nexus.globalquakemodel.org/. Accessed 10 Apr 2017
|
Bettinelli, P., Avouac, J.-P., Flouzat, M., Jouanne, F., Bollinger, L., Willis, P., and Chitrakar, G. R. (2006). Plate Motion of India and Interseismic Strain in the Nepal Himalaya from GPS and DORIS Measurements. J. Geod., 80(8-11), 567–589. https://doi.org/10.1007/s00190-006-0030-3
|
Bhatia, S. C., Kumar, M. R., and Gupta, H. K. (1999). A probabilistic seismic hazard map of India and adjoining regions. Ann. di Geofis., 42(6), 1153–1164.
|
Bilham, R. (2015). Raising Kathmandu. Nat. Geosci., 8(8), 582–584. https://doi.org/10.1038/ngeo2498
|
Bilham, R. (2013). Societal and observational problems in earthquake risk assessments and their delivery to those most at risk. Tectonophys, 584, 166–173. https://doi.org/10.1016/j.tecto.2012.03.023
|
Bollinger, L., Tapponnier, P., Sapkota, S. N., and Klinger, Y. (2016). Slip deficit in central Nepal: omen for a repeat of the 1344 AD earthquake?. Earth Planet. Space, 68, 12. https://doi.org/10.1186/s40623-016-0389-1
|
Bommer, J. J., Scherbaum, F., Bungum, H., Cotton, F., Sabetta, F., and Abrahamson, N. A. (2005). On the use of logic trees for ground-motion prediction equations in seismic-hazard analysis. Bull. Seismol. Soc. Am., 95(2), 377–389. https://doi.org/10.1785/0120040073
|
Chaulagain, H., Rodrigues, H., Silva, V., Spacone, E., and Varum, H. (2015). Seismic risk assessment and hazard mapping in Nepal. Nat. Hazards, 78(1), 583–602. https://doi.org/10.1007/s11069-015-1734-6
|
Chiou, B. S. J., and Youngs, R. R. (2014). Update of the Chiou and Youngs NGA model for the average horizontal component of peak ground motion and response spectra. Earthq. Spectra, 30(3), 1117–1153. https://doi.org/10.1193/072813EQS219M
|
Cornell, C. A. (1968). Engineering seismic risk analysis. Bull. Seismol. Soc. Am., 58(5), 1583–1606.
|
Cornell, C. A, and Van Marke, E. H. (1969). The major influences on seismic risk. In Proceedings of the Third World Conference on Earthquake Engineering (pp. 69–73). Santiago, Chile.
|
Cotton, F., Scherbaum, F., Bommer, J. J., and Bungum, H. (2006). Criteria for selecting and adjusting ground-motion models for specific target regions: Application to central Europe and rock sites. J. Seismol., 10(2), 137–156. https://doi.org/10.1007/s10950-005-9006-7.
|
Danciu, L., Pagani, M., Monelli, D., and Wiemer, S. (2010). GEM1 Hazard : Overview of PSHA Software. GEM Technical Report 2010-2, Pavia, Italy: GEM Foundation.
|
Ding, L., Kapp, P., and Wan, X. Q. (2005). Paleocene-Eocene record of ophiolite obduction and initial India-Asia collision, south central Tibet. Tectonics, 24(3), TC3001. https://doi.org/10.1029/2004TC001729
|
Frankel, A. (1995). Mapping seismic hazard in the central and eastern United States. Seismol. Res. Lett., 66(4), 8–21. https://doi.org/10.1785/gssrl.66.4.8
|
Gardner, J., and Knopoff, L. (1974). Is the sequence of earthquakes in Southern California, with aftershocks removed, Poissonian?. Bull. Seismol. Soc. Am., 64(5), 1363–1367.
|
Grünthal, G., and Wahlström, R. (2003). An MW based earthquake Catalogue for central, northern and northwestern Europe using a hierarchy of magnitude conversions. J. Seismol., 7(4), 507–531. https://doi.org/10.1023/B:JOSE.0000005715.87363.13
|
Hand, E., and Pulla, P. (2015). Nepal disaster presages a coming megaquake. Science, 348(6234), 484–485. https://doi.org/10.1126/science.348.6234.484
|
Hanks, T. C., and Kanamori, H. (1979). A moment magnitude scale. J. Geophys. Res.: Solid Earth, 84(B5), 2348–2350. https://doi.org/10.1029/JB084iB05p02348
|
IBC. (2006). International Building Code. Washington DC: International Code Council.
|
Kaviris, G., Papadimitriou, P., Chamilothoris, L., and Makropoulos, K. (2008). Moment magnitudes for small and intermediate earthquakes. In Proceedings of the 31st General Assembly of the European Seismological Commission. ESC.
|
Kijko, A., and Singh, M. (2011). Statistical tools for maximum possible earthquake magnitude estimation. Acta Geophys., 59(4), 674–700. https://doi.org/10.2478/s11600-011-0012-6
|
Kijko, A., and Smit, A. (2012). Extension of the aki-utsu b-value estimator for incomplete catalogs. Bull. Seismol. Soc. Am., 102(3), 1283–1287. https://doi.org/10.1785/0120110226
|
Kijko, A., Smit, A., and Sellevoll, M. A. (2016). Estimation of earthquake hazard parameters from incomplete data files. Part Ⅲ. Incorporation of uncertainty of earthquake-occurrence model. Bull. Seismol. Soc. Am., 106(3), 1210–1222. https://doi.org/10.1785/0120150252
|
Kolathayar, S., and Sitharam, T. G. (2012). Comprehensive probabilistic seismic hazard analysis of the Andaman-Nicobar regions. Bull. Seismol. Soc. Am., 102(5), 2063–2076. https://doi.org/10.1785/0120110219
|
Letort, J., Bollinger, L., Lyon-Caen, H., Guilhem, A., Cano, Y., Baillard, C., and Adhikari, L. B. (2016). Teleseismic depth estimation of the 2015 Gorkha-Nepal aftershocks. Geophys. J. Int., 207(3), 1584–1595. https://doi.org/10.1093/gji/ggw364
|
Nábělek, J., Hetényi, G., Vergne, J., Sapkota, S., Kafle, B., Jiang, M., Su, H. P., Chen, J., Huang, B.-S., and the Hi-CLIMB Team. (2009). Underplating in the Himalaya-Tibet collision zone revealed by the Hi-CLIMB experiment. Science, 325(5946), 1371–1374. https://doi.org/10.1126/science.1167719
|
Nath, S. K., and Thingbaijam, K. K. S. (2012). Probabilistic seismic hazard assessment of India. Seismol. Res. Lett., 83(1), 135–149. https://doi.org/10.1785/gssrl.83.1.135
|
Ordaz, M. G., Cardona, O.-D., Salgado-Gálvez, M. A., Bernal-Granados, G. A., Singh, S. K., and Zuloaga-Romero, D. (2014). Probabilistic seismic hazard assessment at global level. Int. J. Disaster Risk Reduct., 10, 419–427. https://doi.org/10.1016/j.ijdrr.2014.05.004
|
Ordaz, M., Faccioli, E., Martinelli, F., Aguilar, A., Arboleda, J., Meletti, C., and D’Amico, V. (2015). CRISIS2015 version 2.2: Computer program for computing seismic hazard. Instituto de Ingenieria, UNAM, Mexico.
|
Ornthammarath, T., Warnitchai, P., Worakanchana, K., Zaman, S., Sigbjörnsson, R., and Lai, C. G. (2011). Probabilistic seismic hazard assessment for Thailand. Bull. Earthq. Eng., 9(2), 367–394. https://doi.org/10.1007/s10518-010-9197-3
|
Pandey, M. R., Tandukar, R. P., Avouac, J. P., Vergne, J., and Héritier, T. (1999). Seismotectonics of the Nepal Himalaya from a local seismic network. J. Asian Earth Sci., 17(5-6), 703–712. https://doi.org/10.1016/S1367-9120(99)00034-6
|
Priestley, K., James, J., and Mckenzie, D. (2008). Lithospheric structure and deep earthquakes beneath India, the Himalaya and southern Tibet. Geophys. J. Int., 172(1), 345–362. https://doi.org/10.1111/j.1365-246X.2007.03636.x
|
Rahman, M. M., Bai, L., Khan, N. G., and Li, G. H. (2018). Probabilistic seismic hazard assessment for Himalayan-Tibetan region from historical and instrumental earthquake Catalogs. Pure Appl. Geophys., 175(2), 685–705. https://doi.org/10.1007/s00024-017-1659-y
|
Rajendran, C. P., John, B., and Rajendran, K. (2015). Medieval pulse of great earthquakes in the central Himalaya: Viewing past activities on the frontal thrust. J. Geophys. Res.: Solid Earth, 120(3), 1623–1641. https://doi.org/10.1002/2014JB011015
|
Ram, T. D., and Wang, G. X. (2013). Probabilistic seismic hazard analysis in Nepal. Earthq. Eng. Eng. Vibra., 12(4), 577–586. https://doi.org/10.1007/s11803-013-0191-z
|
Sabetta, F., Lucantoni, A., Bungum, H., and Bommer, J. J. (2005). Sensitivity of PSHA results to ground motion prediction relations and logic-tree weights. Soil Dyn. Earthq. Eng., 25(4), 317–329. https://doi.org/10.1016/j.soildyn.2005.02.002
|
Sapkota, S. N., Bollinger, L., Klinger, Y., Tapponnier, P., Gaudemer, Y., and Tiwari, D. (2013). Primary surface ruptures of the great Himalayan earthquakes in 1934 and 1255. Nat. Geosci., 6(1), 71–76. https://doi.org/10.1038/ngeo1669.
|
Sawires, R., Peláez, J. A., Fat-Helbary, R. E., and Ibrahim, H. A. (2016). Updated probabilistic seismic-hazard values for Egypt. Bull. Seismol. Soc. Am., 106(4), 1788–1801. https://doi.org/10.1785/0120150218
|
Scordilis, E. M. (2006). Empirical global relations converting MS and mb to moment magnitude. J. Seismol., 10(2), 225–236. https://doi.org/10.1007/s10950-006-9012-4
|
Stepp, J. C. (1972). Analysis of completeness of the earthquake sample in the Puget Sound area and its effect on statistical estimates of earthquake hazard. In Proceedings of the 1st International Conference on Microzonazion (vol. 2, pp. 897–910), Seattle.
|
Stevens, V., and Avouac, J.-P. (2015). Interseismic coupling on the Main Himalayan Thrust. Geophys. Res. Lett., 42(14), 5828–5837. https://doi.org/10.1002/2015GL064845
|
Stewart, J. P., Douglas, J., Javanbarg, M. B., Di Alessandro, C., Bozorgnia, Y., Abrahamson, N. A., Boore, D. M., Campbell, K. W., Delavaud, E., and Stafford, P. J. (2013). GEM-PEER Task 3 Project: Selection of a Global Set of Ground Motion Prediction Equations. PEER Report 2013/22.
|
Stirling, M., and Goded, T. (2012). Magnitude scaling relationships. Report Produced for the GEM Faulted Earth & Regionalisation Global Componets, GNS Science Miscellaneous Series, 42, 35.
|
Styron, R., Taylor, M., and Okoronkwo, K. (2010). Database of active structures from the Indo-Asian Collision. EOS, 91(20), 181–182. https://doi.org/10.1029/2010EO200001
|
Szeliga, W., Hough, S., Martin, S., and Bilham, R. (2010). Intensity, magnitude, location, and attenuation in India for felt earthquakes since 1762. Bull. Seismol. Soc. Am., 100(2), 570–584. https://doi.org/10.1785/0120080329
|
Tinti, S., and Mulargia, F. (1985). An improved method for the analysis of the completeness of a seismic catalogue. Lett. Nuovo Cimento, 42(1), 21–27. https://doi.org/10.1007/BF02739471
|
Utsu, T. (1965). A method for determining the value of b in a formula logN=a−bM showing the magnitude-frequency relation for earthquakes. Geophys. Bull. Hokkaido Univ., 13, 99–103.
|
Wang, Z. M., Butler, D. T., Woolery, E. W., and Wang, L. M. (2012). Seismic hazard assessment for the Tianshui urban area, Gansu Province, China. Int. J. Geophys., 2012, 461863. https://doi.org/10.1155/2012/461863
|
Wells, D. L., and Coppersmith, K. J. (1994). New empirical relationships among magnitude, rupture length, rupture width, rupture area, and surface displacement. Bull. Seismol. Soc. Am., 84(4), 974–1002.
|
Wiemer, S., and Wyss, M. (2000). Minimum magnitude of completeness in earthquake catalogs: examples from Alaska, the Western United States, and Japan. Bull. Seismol. Soc. Am., 90(4), 859–869. https://doi.org/10.1785/0119990114
|
Woo, G. (1996). Kernel estimation methods for seismic hazard area source modeling. Bull. Seismol. Soc. Am., 86(2), 353–362.
|
Wyss, M. (2005). Human losses expected in Himalayan earthquakes. Nat. Hazards, 34(3), 305–314. https://doi.org/10.1007/s11069-004-2073-1
|
Yen, Y. T., and Ma, K. F. (2011). Source-Scaling relationship for M 4.6-8.9 earthquakes, specifically for earthquakes in the Collision Zone of Taiwan. Bull. Seismol. Soc. Am., 101(2), 464–481. https://doi.org/10.1785/0120100046
|
Yin, A. (2006). Cenozoic tectonic evolution of the Himalayan orogen as constrained by along-strike variation of structural geometry, exhumation history, and foreland sedimentation. Earth-Sci. Rev., 76(1-2), 1–131. https://doi.org/10.1016/j.earscirev.2005.05.004
|
Youngs, R. R., Chiou, S.-J., Silva, W. J., and Humphrey, J. R. (1997). Strong ground motion attenuation relationships for subduction zone earthquakes. Seismol. Res. Lett., 68(1), 58–73. https://doi.org/10.1785/gssrl.68.1.58
|
Zhang, P. Z., Yang, Z. X., Gupta, H. K., Bhatia, S. C., and Shedlock, K. M. (1999). Global seismic hazard assessment program (GSHAP) in continental Asia. Ann. Di Geof., 42(6), 1167–1190. https://doi.org/10.4401/ag-3778
|
Zhang, W. B., Iwata, T., and Irikura, K. (2006). Dynamic simulation of a dipping fault using a three-dimensional finite difference method with nonuniform grid spacing. J. Geophys. Res., 111, B05301. https://doi: 10.1029/2005JB003725
|
Zhao, J. X., Zhang, J., Asano, A., Ohno, Y., Oouchi, T., Takahashi, T., Ogawa H., Irikura K., Thio H. K., Fukushima, Y. (2006). Attenuation relations of strong ground motion in Japan using site classification based on predominant period. Bull. Seismol. Soc. Am., 96(3), 898–913. https://doi.org/10.1785/0120050122
|
LianXing Wen, Sheng Yu. 2023: The China Seismological Reference Model project. Earth and Planetary Physics, 7(5): 521-532. DOI: 10.26464/epp2023078 | |
Zheng Xiang, BinBin Ni, YangXiZi Liu, XuDong Gu, Song Fu, Wei Xu, Xing Cao, Xin Ma, DeYu Guo, JunHu Dong, JingLe Hu. 2023: Modeling the energetic electron fluxes in the inner radiation belt based on a drift-source model. Earth and Planetary Physics, 7(1): 100-108. DOI: 10.26464/epp2023012 | |
MoRan Liu, Chen Zhou, Ting Feng. 2022: Electron acceleration by Langmuir turbulence in ionospheric heating. Earth and Planetary Physics, 6(6): 529-535. DOI: 10.26464/epp2023001 | |
QiZhen Du, WanYu Wang, WenHan Sun, Li-Yun Fu. 2022: Seismic attenuation compensation with spectral-shaping regularization. Earth and Planetary Physics, 6(3): 259-274. DOI: 10.26464/epp2022024 | |
BinBin Liao, XiaoDong Chen, JianQiao Xu, JiangCun Zhou, HePing Sun. 2022: Theoretical calculation of tidal Love numbers of the Moon with a new spectral element method. Earth and Planetary Physics, 6(3): 241-247. DOI: 10.26464/epp2022025 | |
XinZhou Li, ZhaoJin Rong, JiaWei Gao, Yong Wei, Zhen Shi, Tao Yu, WeiXing Wan. 2020: A local Martian crustal field model: Targeting the candidate landing site of the 2020 Chinese Mars Rover. Earth and Planetary Physics, 4(4): 420-428. DOI: 10.26464/epp2020045 | |
Zhi Wei, Li Zhao. 2019: Lg-Q model and its implication on high-frequency ground motion for earthquakes in the Sichuan and Yunnan region. Earth and Planetary Physics, 3(6): 526-536. DOI: 10.26464/epp2019054 | |
YouShan Liu, Tao Xu, YangHua Wang, JiWen Teng, José Badal, HaiQiang Lan. 2019: An efficient source wavefield reconstruction scheme using single boundary layer values for the spectral element method. Earth and Planetary Physics, 3(4): 342-357. DOI: 10.26464/epp2019035 | |
Xu Zhang, Zhen Fu, LiSheng Xu, ChunLai Li, Hong Fu. 2019: The 2018 MS 5.9 Mojiang Earthquake: Source model and intensity based on near-field seismic recordings. Earth and Planetary Physics, 3(3): 268-281. DOI: 10.26464/epp2019028 | |
BoJing Zhu, Hui Yan, David A Yuen, YaoLin Shi. 2019: Electron acceleration in interaction of magnetic islands in large temporal-spatial turbulent magnetic reconnection. Earth and Planetary Physics, 3(1): 17-25. DOI: 10.26464/epp2019003 |
Date | Latitude (°N) | Longitude (°E) | Location | Magnitude (MW) | Reference |
June 7, 1255 | 27.70 | 85.30 | Kathmandu | 7.7 | NOAA |
June 6, 1505 | 29.50 | 83.00 | Lo Mustang | 8.2 | A&D |
Jul. 25, 1720 | 30.00 | 80.00 | N Uttar-pradesh | 7.5 | A&D |
Aug. 26, 1833 | 27.70 | 85.70 | Nepal | 7.6 | A&D |
Oct. 14, 1911 | 31.00 | 80.50 | Tibet | 6.8 | ISC |
Aug. 28, 1916 | 29.90 | 80.50 | Uttaranchal | 7.2 | A&D |
Jan. 15, 1934 | 27.55 | 87.09 | Bihar-Nepal | 8.2 | S |
May 27, 1936 | 28.50 | 83.50 | Nepal | 7.0 | ISC |
June 4, 1945 | 30.00 | 80.00 | Nepal | 6.5 | ISC |
Aug. 15, 1950 | 28.70 | 96.70 | Assam | 8.5 | A&D |
Sep. 4, 1954 | 28.30 | 83.80 | Nepal | 6.5 | ISC |
Sep. 20, 1988 | 26.72 | 86.63 | Nepal | 6.6 | ISC |
Sep. 18, 2011 | 27.81 | 88.20 | Sikkim, India | 6.9 | ISC |
April 25, 2015 | 28.28 | 84.79 | Gorkha, Nepal | 7.8 | ISC |
May 12, 2015 | 27.83 | 86.14 | Nepal | 7.1 | ISC |
Notes: NOAA: National Oceanic and Atmospheric Administration; A&D:Ambraseys and Douglas (2004); S: Sapkota et al. (2013); ISC: International Seismological Centre. |
(Scordilis, 2006) | (Grünthal and Wahlström, 2003) | (Kaviris et al., 2008) |
MW = 0.67 MS + 2.07 for 3.0≤MS≤6.1 | MW = 0.67(±0.11) + 0.56(±0.08) ML + 0.046(±0.013) ML2 | MW = MD + 0.5 (MD<3.0) |
MW = 0.99 MS + 0.08 for 6.2≤MS≤8.2 | MW = MD + 0.6 (MD≥3.0) | |
MW = 0.85 Mb + 1.03 for 3.5≤Mb≤6.2 | ||
Notes: MW: Moment magnitude; MS: Surface wave magnitude; ML: Local magnitude; Mb: Body wave magnitude; MD: Duration magnitude. |
Fault Name | Length (km) | M0* | Width (km) | Slip rate (mm/year) | b | σb | λ |
MFT-1 | 1300 | 2.67888E+22 | 100 | 20.5 | 0.76 | 0.09 | 4.334 |
MFT-2 | 330 | 1.6133E+21 | 100 | 18.0 | 0.61 | 0.04 | 2.703 |
MFT-3 | 800 | 9.86865E+21 | 100 | 20.0 | 0.73 | 0.09 | 1.371 |
Tibrikot | 62 | 5.50155E+19 | 39 | 20.5 | 0.69 | 0.14 | 0.055 |
Humla | 76 | 8.27582E+19 | 47 | 20.5 | 0.61 | 0.13 | 0.370 |
Tingri | 44 | 2.77124E+19 | 30 | 18.0 | 0.51 | 0.1 | 0.233 |
Kung Co | 68 | 6.62049E+19 | 43 | 18.0 | 0.44 | 0.09 | 0.090 |
Karakoram | 300 | 1.3287E+21 | 110 | 10.0 | 0.63 | 0.09 | 0.460 |
f1 | 70 | 7.01679E+19 | 44 | 20.5 | 0.54 | 0.12 | 0.154 |
f2 | 55 | 4.32837E+19 | 36 | 20.5 | 0.55 | 0.22 | 0.048 |
f3 | 65 | 6.04792E+19 | 41 | 20.5 | 1.2 | 0.12 | 0.164 |
f4 | 139 | 2.79178E+20 | 76 | 20.5 | 0.69 | 0.16 | 0.425 |
f5 | 92 | 1.21496E+20 | 54 | 18.0 | 0.71 | 0.1 | 0.223 |
f6 | 179 | 4.65654E+20 | 93 | 18.0 | 0.63 | 0.1 | 0.148 |
f7 | 103 | 1.52508E+20 | 60 | 18.0 | 0.48 | 0.1 | 0.066 |
f8 | 82 | 9.64029E+19 | 50 | 18.0 | 0.54 | 0.21 | 0.102 |
Notes: Fault numbering (f1–f8) is chosen arbitrarily since no naming appears on the source files. Rupture width for MFT-1–MFT-3 is taken from Berryman et al. (2014); and for the rest of the faults is estimated using the relationship of Yen YT and Ma KF (2011). Slip rates are recorded from Ader et al. (2012). λ, b value, and σb are used for the Gutenberg-Richter seismicity model. M0* is the weighted seismic moment. |
Zones | a-value | b-value | σb* | λ* | Mu* | σ(Mu)* |
Z1 | 4.48 | 0.85 | 0.20 | 0.086 | 6.5 | 0.86 |
Z2 | 4.16 | 0.77 | 0.18 | 0.115 | 6.5 | 0.57 |
Z3 | 3.40 | 0.70 | 0.33 | 0.013 | 6.5 | 0.49 |
Z4 | 4.22 | 0.82 | 0.12 | 0.060 | 6.5 | 0.29 |
Z5 | 4.10 | 0.82 | 0.12 | 0.033 | 6.5 | 0.47 |
Z6 | 4.84 | 0.82 | 0.12 | 0.020 | 7.2 | 0.86 |
Z7 | 3.22 | 0.47 | 0.20 | 0.033 | 7.5 | 0.72 |
Z8 | 4.84 | 0.82 | 0.12 | 0.019 | 7.7 | 1.00 |
Z9 | 4.04 | 0.70 | 0.07 | 0.157 | 8.1 | 0.30 |
Z10 | 3.75 | 0.56 | 0.05 | 0.137 | 8.5 | 0.46 |
Z11 | 4.23 | 0.60 | 0.06 | 0.240 | 8.5 | 0.45 |
Z12 | 4.16 | 0.53 | 0.04 | 0.240 | 8.5 | 0.70 |
Z13 | 4.46 | 0.80 | 0.17 | 0.187 | 7.0 | 0.34 |
Z14 | 4.31 | 0.66 | 0.11 | 0.198 | 8.0 | 0.93 |
Z15 | 4.10 | 0.66 | 0.17 | 0.138 | 8.5 | 0.43 |
Z16 | 4.40 | 0.68 | 0.06 | 0.493 | 8.5 | 0.56 |
Z17 | 4.67 | 0.64 | 0.05 | 0.482 | 8.6 | 0.51 |
Z18 | 4.63 | 0.69 | 0.08 | 0.243 | 8.5 | 0.31 |
Z19 | 3.98 | 0.71 | 0.14 | 0.068 | 6.5 | 0.36 |
Z20 | 4.48 | 0.77 | 0.15 | 0.081 | 7.0 | 0.44 |
Z21 | 4.96 | 0.82 | 0.12 | 0.080 | 6.5 | 0.36 |
Z22 | 4.51 | 0.63 | 0.09 | 0.362 | 6.5 | 0.30 |
Z23 | 4.38 | 0.64 | 0.09 | 0.201 | 6.6 | 0.30 |
Notes: σb* is the standard error of b value; λ* is the rate of seismicity per year; σ(Mu)* is the standard deviation of expected maximum magnitude (Mu*). |