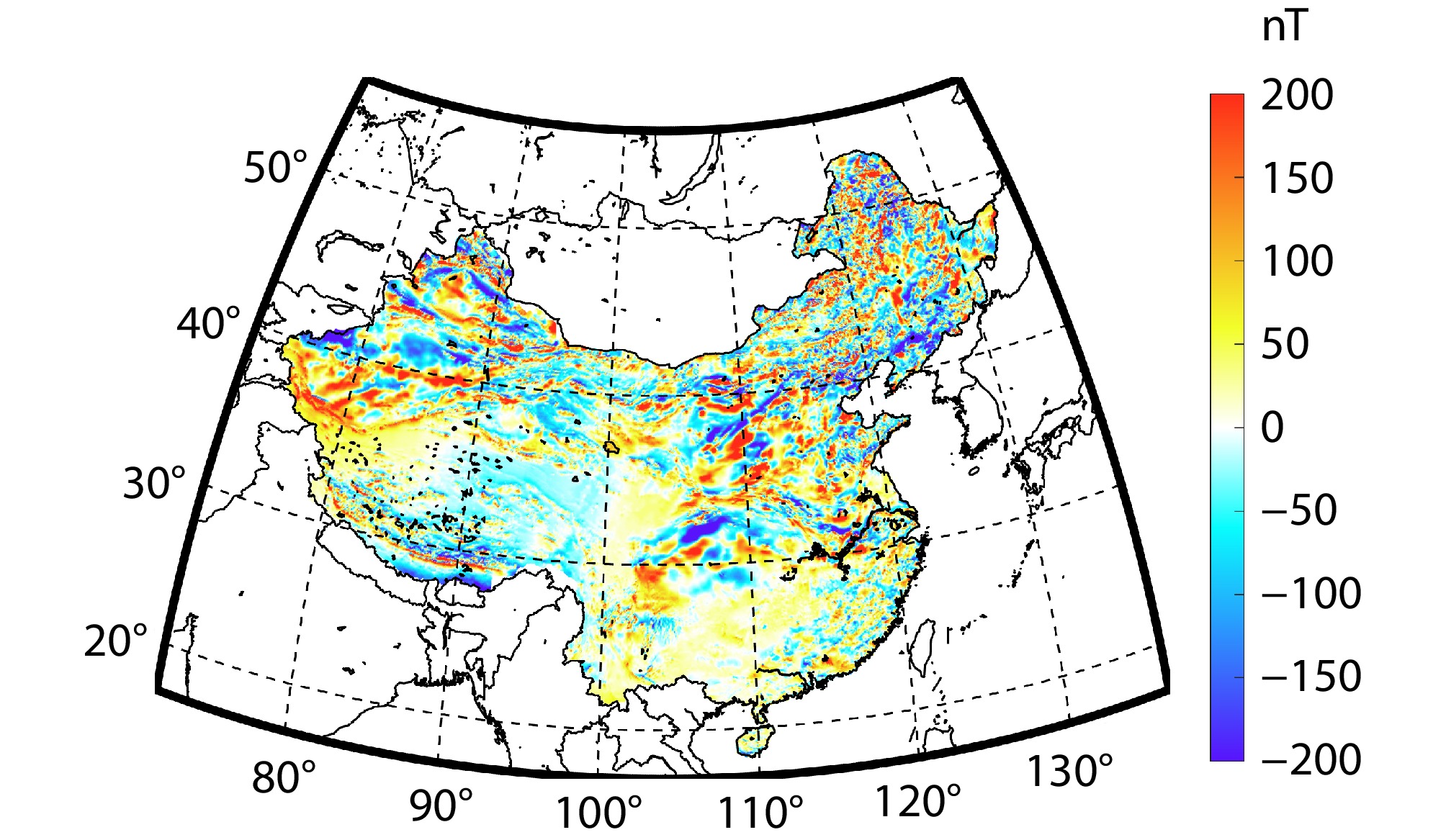
Citation: | Zhang, P., Du, J. S., Yin, L., Yan, Q., Zhang, J. X., Li, H. P., Zhang, K., and Chen, C. (2025). A high-resolution lithospheric magnetic field model of China and surroundings by using CHAMP, Swarm, CSES-1, and MSS-1 satellite magnetic measurements and aeromagnetic anomaly data. Earth Planet. Phys., 9(3), 686–696. DOI: 10.26464/epp2025057 |
The CUG_CLMFM3D series comprises high-resolution three-dimensional lithospheric magnetic field models for China and its surroundings. The first version, CUG_CLMFM3Dv1, is a spherical cap harmonic model integrating the WDMAMv2 (World Digital Magnetic Anomaly Map version 2) global magnetic anomaly grid and nearly a decade of CHAMP (Challenging Minisatellite Payload for Geophysical Research and Application) satellite vector data. It achieves a ~5.7 km resolution but has limitations: the WDMAMv2 grid lacks high-resolution data in the southern Xinjiang and Tibet regions, which leads to missing small- to medium-scale anomalies, and unfiltered CHAMP data introduce low-frequency conflicts with global spherical harmonic models. Above the altitude of 150 km, correlations with global models drop below 0.9. The second version, CUG_CLMFM3Dv2, addresses these issues by incorporating 5-km-resolution aeromagnetic data and rigorously processed satellite data from CHAMP, Swarm, CSES-1 (China Seismo-Electromagnetic Satellite 1), and MSS-1 (Macau Science Satellite 1). The comparison analysis shows that the CUG_CLMFM3Dv2 captures finer high-frequency details and more stable long-wavelength signals, offering improved magnetic anomaly maps for further geological and geophysical studies.
Modeling and understanding the underlying mechanisms of the Earth’s magnetic field and its spatiotemporal variations are not only essential for scientific exploration but also vital for numerous industrial and technological applications. Geomagnetic field observations are widely understood to reflect a complex, real-time superposition of multiple sources (Finlay et al., 2020; Sabaka et al., 2020), including (1) the core field generated by electric currents in the fluid outer core; (2) the induced field generated by the conductive mantle, crust, and oceans; (3) the crustal magnetization field; (4) electric current activity in the ionosphere and magnetosphere; and (5) noise from measurement instrument imperfections.
Among these magnetic field sources, the core field alone generates a magnetic intensity of nearly 25,000 to 65,000 nanoteslas (nT) at the Earth’s near-surface region (e.g., Finlay et al., 2020; Sabaka et al., 2020; Alken et al., 2021). The lithosphere also contributes a substantial proportion that cannot be ignored, and lithospheric magnetic anomalies reaching several to tens of thousands of nanoteslas can be found, particularly in regions with strong magnetization (e.g., Lesur et al., 2016; Xiong et al., 2016; Meyer et al., 2017; Djomani et al., 2019). High-resolution and high-precision lithospheric magnetic field models are thus crucial for describing these high-amplitude, small-scale anomalies, as they provide valuable insights for resource and energy exploration, geological interpretation, and magnetic-field-based navigation.
Modeling the magnetic field in China and its surrounding areas has been a prominent research topic (An ZC, 1993, 2003; An ZC et al., 1998; Gu ZW et al., 2006; Ou JM et al., 2013; Feng Y et al., 2016, 2017, 2023a, b; Jiang Y et al., 2021; Wang J et al., 2021, 2023, 2025; Zhang P et al., 2024). In particular, the launches of the China Seismo-Electromagnetic Satellite 1 (CSES-1; Shen XH et al., 2018; Yang YY et al., 2021) and the Macau Science Satellite 1 (MSS-1, Zhang K, 2023), along with the public release of the airborne magnetic anomaly map of continental China (Xiong SQ et al., 2016; https://geocloud.cgs.gov.cn/), have further intensified interest in this field.
Previous studies have shown that lithospheric magnetic field models based solely on satellite data can achieve a resolution of approximately 108 km (e.g., Lithospheric model derived from CHAMP and Swarm satellite data, version 1 [LCS-1]; Olsen et al., 2017). However, when near-surface data are incorporated, the resolution can be improved to approximately 19–25 km (e.g., Enhanced Magnetic Model 2017 [EMM2017], www.ngdc.noaa.gov/geomag/EMM/; World Digital Magnetic Anomaly Map version 2 [WDMAMv2], Lesur et al., 2016; A Spherical Harmonic Model of Earth's Lithospheric Magnetic Field up to Degree 1050, [SH1050], Thébault et al., 2021). These global models, however, rely on near-surface data from sources such as the Earth Magnetic Anomaly Grid (2-arc-min resolution), version 3 (EMAG2v3; Meyer et al., 2017) or WDMAMv2 (Lesur et al., 2016), which use datasets with limitations in East Asia. Specifically, the lack of high-resolution data in the southern regions of Xinjiang and Tibet leads to missing small- to medium-scale details in these areas (e.g., Luo Y et al., 2024).
A physically robust modeling method, based on well-processed near-surface and satellite data, is essential for constructing a high-resolution and high-precision magnetic field model. Feng Y et al. (2023a, b) adopted the airborne magnetic anomaly map of continental China and vector measurements from the CHAMP mission to construct a high-resolution model for the Xinjiang and Tibet areas by using the Three-Dimensional Surface Spline (3DSS) method. Although the model achieved superior resolution and reliability in representing the total magnetic intensity field, the 3DSS method is limited by its inability to convert the total magnetic intensity field into a vector field. Jiang Y et al. (2021) proposed the long-wavelength lithospheric magnetic field model of China (CLAS), using the airborne magnetic anomaly map of continental China and synthetic satellite data with a depleted basis spherical harmonic analysis approach. Although CLAS demonstrates strong physical properties and reliability, its resolution is limited to approximately 50 km. We previously developed the CUG_CLMFM3Dv1 (Zhang P et al., 2024), a model based on revised spherical cap harmonic analysis (R-SCHA; Thébault, 2003; Thébault et al., 2004, 2006a), which combines near-surface data from WDMAMv2 and vector measurements from the CHAMP mission. This model achieves a resolution of up to 5.7 km but, as noted, lacks small- to medium-scale details in western China. Additionally, insufficient along-track filtering and leveling of satellite data resulted in reduced consistency with satellite data-based spherical harmonic models at higher altitudes. Nonetheless, this case study demonstrated that the CUG_CLMFM3D series is capable of modeling the lithospheric magnetic field with high resolution and precision by using multiple data sources.
Through extensive data collection and processing and careful model construction, the limitations identified in the first version of CUG_CLMFM3D have been successfully addressed. In the following sections, we introduce our updated regional model of China and surroundings. Section 2 provides the basic methodology of the R-SCHA, Section 3 outlines the data processing, Sections 4 and 5 present the results and discussion, respectively, and Section 6 concludes the study.
Revised spherical cap harmonic analysis (Thébault, 2003; Thébault et al., 2004, 2006a) is a potential field modeling technique applied at a regional scale. The modeling region is defined between two spherical surfaces with radii r1 and r2, and a cone with its vertex at the Earth’s center. The aperture of the cone is denoted as θ0 (e.g., Thébault et al., 2004, 2006a). For such a boundary value problem, solving Laplace’s equation yields the series expansion expression of the magnetic potential V:
V(r,θ,λ)=a∑k⩾ | (1) |
where r, θ, and λ represent the radius, colatitude, and longitude, respectively. The constant a refers to the Earth’s reference radius, which is taken as 6371.2 km in this study;
The completeness, orthogonality, and convergence of the basis functions, along with the null flux condition of the method, make R-SCHA effective for potential field modeling and forward prediction. As a result, this analysis has been widely used in lithospheric magnetic field modeling (e.g., Vervelidou et al., 2018; Thébault et al., 2021; Zhang P et al., 2024), core field modeling (e.g., Talarn et al., 2017), and paleomagnetic field modeling (e.g., Pavón-Carrasco et al., 2021; Fan YC et al., 2024), among others.
Scalar data are treated as a projection of the vector in the direction of the core field (Blakely, 1995) and are referred to as the total magnetic intensity (TMI). The core field model used in this study comprises the spherical harmonic coefficients with degrees/orders 1–15 from the newly released CHAOS 8.2 model (http://www.spacecenter.dk/files/magnetic-models/CHAOS-8/index.html; Finlay et al., 2020).
In practical situations, magnetic data are discretized and contaminated by noise. Modeling such data involves an inverse process of the previously described physical system (i.e., estimating the R-SCHA harmonic coefficients). By separating the R-SCHA coefficients from the series expression, the forward process can be described as a linear equation:
{\boldsymbol {Gm}} = {\boldsymbol {d}} , | (2) |
where G is the kernel matrix containing the observation system information and special functions, d is the vector of the observed data, and m is the vector of the R-SCHA harmonic coefficients. We estimate the weighted least squares solution as follows:
{{\boldsymbol {m}}^{{\text{est}}}} = {\left( {{{\boldsymbol {G}}^{\text{T}}}{{\boldsymbol {W}}_d}{\boldsymbol {G}} + \lambda {\boldsymbol {I}}} \right)^{ - 1}}{{\boldsymbol {G}}^{\text{T}}}{{\boldsymbol {W}}_d}{\boldsymbol {d}} , | (3) |
where Wd represents the data weighting vector, λ represents the damping parameter, and I represents an identity matrix.
The kernel matrix of R-SCHA is ill-conditioned; therefore, a preconditioned matrix S is introduced to address the nonconvergence issue in the optimization process (Pilkington, 1997). Thus, Equation (3) can be rewritten as
{{\boldsymbol {m}}^{{\text{est}}}} = {\left( {{\boldsymbol {S}}{{\boldsymbol {G}}^{\text{T}}}{{\boldsymbol {W}}_d}{\boldsymbol {G}} + \lambda {\boldsymbol {I}}} \right)^{ - 1}}{\boldsymbol {SS}}{{\boldsymbol {G}}^{\text{T}}}{{\boldsymbol {W}}_d}{\boldsymbol {d}} . | (4) |
The Bi-Conjugate Gradient Stabilized method is used as the optimization algorithm to solve the linear equation (van der Vorst, 1992).
The formula for the power spectrum of the R-SCHA model is provided by Vervelidou and Thébault (2015):
\begin{gathered} E = \sum\limits_{m = 0}^\infty {\frac{{\left( {1 + {\delta _{m,0}}} \right)}}{{2\left( {1 - \cos {\theta _0}} \right)}}} \sum\limits_{k = 0}^\infty {\left\{ {\left( {{n_k} + 1} \right)\left( {2{n_k} + 1} \right){{\left( {\frac{a}{r}} \right)}^{2{n_k} + 4}}\left[ {{{\left( {g_{{n_k}}^{i,m}} \right)}^2} + {{\left( {h_{{n_k}}^{i,m}} \right)}^2}} \right]} \right.} \\ \left. { + {n_k}\left( {2{n_k} + 1} \right){{\left( {\frac{r}{a}} \right)}^{2{n_k} - 2}}\left[ {{{\left( {g_{{n_k}}^{e,m}} \right)}^2} + {{\left( {h_{{n_k}}^{e,m}} \right)}^2}} \right]} \right\}{\left\| {P_{{n_k}}^m} \right\|^2}, \\ \end{gathered} | (5) |
where E denotes the energy in the modeling region and δm,0 denotes the Dirac delta function.
The central point of the modeling area for CUG_CLMFM3Dv2 is located at 114°E and 39°N. Near-surface and satellite magnetic data are selected within a spherical cap with a 26° aperture.
The compiled airborne magnetic anomaly map of continental China is used as the near-surface data for the modeling. According to Xiong SQ et al. (2016), 143 high-precision and 266 medium-precision measurements from a total of 409 survey areas were compiled into a 5-km-resolution magnetic anomaly map, covering a land area of more than 9 million square kilometers, excluding Taiwan Province. The corresponding data are presented in Figure 1.
To maintain the integrity of the near-surface data, we embedded the continental China magnetic data into the EMAG2v3 compiled global magnetic anomaly grid (Meyer et al., 2017), as shown in Figure 2a. Unlike satellite-surveyed data, long-wavelength components in compiled magnetic anomaly grids are often unreliable (Ravat et al., 2003). To prevent computational instability due to low-frequency conflicts between near-surface and satellite-surveyed data, we utilized an extraction and replacement scheme (Zhang P et al., 2022). The low-frequency replaced near-surface data are shown in Figure 2b. This scheme extracts long-wavelength components of spherical harmonic degrees/orders 16–90, shown in Figure 2c, from the regional magnetic data and replaces the extracted long-wavelength components with the corresponding components from the LCS-1 model (Olsen et al., 2017), shown in Figure 2d. When comparing Figure 2c with Figure 2d, we found relatively strong inconsistencies between the long-wavelength components of the lithospheric magnetic field from the near-surface data and the satellite data-derived model. Thus, it is necessary to replace the long-wavelength components of the near-surface data with those of the satellite data-derived model. Otherwise, the fusion of the near-surface and satellite data sets will fail.
The L1B products from the CHAMP (Maus, 2007; time period: 07/19/2000 to 09/17/2010), Swarm Alpha and Bravo (Friis-Christensen et al., 2006; Olsen et al., 2016; time period: 01/01/2014 to 12/31/2024), CSES-1 (Shen XH et al., 2018; Yang YY et al., 2021; time period: 01/01/2024 to 07/31/2024), and MSS-1 (Zhang K, 2023; time period: 11/02/2023 to 12/31/2024) satellite missions were collected.
Satellite measurements are generally considered as a superposition of various magnetic fields, including the core field, mantle induction field, lithospheric magnetic field, tidally generated magnetic field, and magnetic fields produced by complex current motions in the ionosphere and magnetosphere, among others. Within this real-time dynamic physical system, the signals from the lithospheric magnetic field are quasistatic and contain a substantial proportion of high-frequency components at satellite altitudes. Consequently, data selection, correction for contributions from other field sources, and high-pass filtering along the satellite orbit are key processes in lithospheric magnetic field modeling.
Only data from periods of the Earth’s relatively low magnetic activity were retained. We selected data from periods when the Kp index (which describes irregular perturbations caused by solar particle radiation; Dieminger et al., 1996; Kauristie et al., 2017) was below 2°, the time gradient of the RC index (which describes variations of the equatorial magnetospheric ring current; Olsen et al., 2014) changed by less than 2 nT/hour, and the Sun was at least 10° below the geographic horizon. The sampling rate of the data was reduced to 30 seconds. Additionally, the “F_error” and “Att_error” values in the Swarm product were restricted to 0–0.2, and 0–1.8, respectively, to exclude data affected by instrumentation disturbances.
The contributions from the core field and the external field were simulated and extracted using the CHAOS-8.2 model. However, even after this correction, the lithospheric signal remained mixed with uncorrected low-frequency signals and noise caused by space currents. To address this issue, a high-pass spherical harmonic filter (Thébault et al., 2017) with a degrees/orders of 15 was designed to remove the uncorrected low-frequency signals. During the filtering process, spherical harmonic degrees/orders 16–80 of the LCS-1 were removed and then restored to prevent any harm to the low-frequency components of the lithospheric magnetic signals (Thébault et al., 2021).
Finally, vector data from regions equatorward of ±55° magnetic latitude were further selected for periods when the merging electric field Em index (averaged over the previous 2 hours, describing the merging electric field at the magnetopause; Kan and Lee, 1979; Newell et al., 2007) was less than or equal to 0.8 mV/m, the interplanetary magnetic field (IMF) Bz at the magnetopause (averaged over the previous 2 hours) was positive, and the IMF By at the magnetopause (averaged over the previous 2 hours) was less than +3 nT in the northern magnetic hemisphere and greater than –3 nT in the southern magnetic hemisphere. Additionally, satellite vector data with amplitudes exceeding ±50 nT were excluded, as lithospheric magnetic field signals should not exhibit such high amplitudes at satellite altitudes. The static parameters of the satellite-surveyed data are presented in Table 1.
Satellite | TMI data | |||
Number of data | Altitude range (km) | Standard deviation (nT) | ||
CHAMP | 194 to 480 | ±3.28 | ||
CSES-1 | 5157 | 493 to 521 | ±1.74 | |
MSS-1 | 419 to 482 | ±2.15 | ||
Swarm Alpha | 422 to 503 | ±2.15 | ||
Swarm Bravo | 494 to 522 | ±1.73 | ||
Satellite | Vector data | |||
Number of data (vector triplets) | Altitude range (km) | Standard deviation (nT) | ||
CHAMP | Bx | 245 to 481 | ±3.39 | |
By | ±3.73 | |||
Bz | ±3.62 | |||
MSS-1 | Bx | 419 to 482 | ±1.86 | |
By | ±1.40 | |||
Bz | ±2.27 | |||
Swarm Alpha | Bx | 422 to 503 | ±2.20 | |
By | ±3.73 | |||
Bz | ±2.33 | |||
Swarm Bravo | Bx | 494 to 522 | ±1.91 | |
By | ±3.56 | |||
Bz | ±1.90 |
The aperture of the cone θ0 is set to 27° (1° larger than the data selection area), and the radii of the two spherical caps r1 and r2 are set to a–15 km and a+550 km, respectively. This setup mitigates the Gibbs effect by ensuring that the modeled area is fully covered and slightly larger than the coverage of the modeling data.
According to Thébault et al. (2006b), the internal Legendre basis contributes more in the lower part of the cone, primarily determining the resolution and fit of the near-surface data. In contrast, the external Legendre basis has a greater contribution in the upper part of the cone, primarily determining the resolution and fit of the satellite data. Therefore, the maximum truncation indexes of the internal and external Legendre basis are set to 530 and 18, respectively, allowing the model to achieve up to 3500 and 120 spherical harmonic degrees/orders at the near surface and 300 km altitude, respectively. The maximum truncation index for the Mehler and constant basis is set to 3, considering the data gap between the near-surface and satellite data. The inverse of the standard deviation for both near-surface and satellite data is used as the data weight in the optimization to balance the model fit across different data sets.
Magnetic models need to be reasonably damped when the modeling area is near the magnetic poles or equator (e.g., Olsen et al., 2017; Thébault et al., 2017). In regions near the magnetic poles, because of the intense activity of space currents, particularly between ±60° and ±80° magnetic latitude, the noise level in processed satellite data, especially in the northern and eastern vector components, increases significantly (e.g., Olsen et al., 2017). In such cases, damping is necessary, and the northern and eastern vector components may need to be discarded during modeling. Similarly, in regions close to the magnetic equator, the noise level in satellite data is raised by the equatorial magnetospheric ring current, and vector fields recovered from TMI data are subject to the Backus (1970) effect (e.g., Lesur et al., 2016; Thébault et al., 2021). This effect leads to an anomalous enhancement of the eastern and radial components near the magnetic equator. Damping must be applied to minimize components perpendicular to the core field to address this issue. Fortunately, neither of these scenarios is relevant to the study area in this research, so the damping factor λ is set to 0.
The model is constructed after estimating the R-SCH coefficients based on the current parameter settings. We then forward calculate the data at the modeling data points to analyze the misfit of the model to the observation data. The reconstructed near-surface data and the fitting residuals are shown in Figure 3. Additionally, the static parameters of the fitting residuals for the near-surface and satellite data are presented in Table 2. Figures of the reconstructed satellite data and the fitting residuals can be found in the Supplementary Material.
Data source | Data type | Minimum (nT) | Maximum (nT) | Mean (nT) | RMSE (nT) |
Near surface | TMI | −436.69 | 822.98 | 3.12 × 10−2 | ±12.04 |
CHAMP | TMI | −13.08 | 11.07 | 6.56 × 10−4 | ±0.54 |
Vector Bx | −49.58 | 45.02 | 1.11 × 10−3 | ±2.19 | |
Vector By | −43.83 | 45.18 | −3.24 × 10−4 | ±3.04 | |
Vector Bz | −26.97 | 22.00 | −7.42 × 10−3 | ±1.23 | |
CSES HPM6 | TMI | −6.36 | 10.34 | 2.08 × 10−3 | ±0.33 |
MSS-1 | TMI | −1.35 | 2.12 | −1.42 × 10−4 | ±0.13 |
Vector Bx | −2.16 | 3.27 | 1.28 × 10−3 | ±0.26 | |
Vector By | −5.48 | 9.04 | −1.69 × 10−2 | ±0.37 | |
Vector Bz | −5.08 | 6.01 | 3.20 × 10−4 | ±0.29 | |
Swarm Alpha | TMI | −14.10 | 13.32 | 8.12 × 10−4 | ±0.46 |
Vector Bx | −25.16 | 39.22 | 2.38 × 10−3 | ±1.42 | |
Vector By | −48.19 | 47.98 | −2.74 × 10−3 | ±3.46 | |
Vector Bz | −11.32 | 15.39 | 6.30 × 10−4 | ±0.71 | |
Swarm Bravo | TMI | −8.41 | 6.92 | −3.66 × 10−4 | ±0.35 |
Vector Bx | −28.46 | 27.45 | −2.85 × 10−3 | ±1.34 | |
Vector By | −47.87 | 49.36 | 8.23 × 10−4 | ±3.37 | |
Vector Bz | −18.50 | 23.02 | −2.34 × 10−3 | ±0.64 | |
aRMSE, root mean square error; HPM6, High-Precision Magnetometer No.6. |
The reconstructed data and fitting residuals demonstrate that the model has successfully captured the details of lithospheric magnetic anomalies across all scales. The unfitted anomalies in the near-surface data are predominantly short-wavelength features that fall beyond the resolution capability of the model. In the satellite data, the unfitted signals primarily appear in the northern and eastern components, where high-amplitude noise is distributed along the satellite orbit. Compared with CUG_CLMFM3Dv1, the low-frequency replacement process in the near-surface data and the along-track filtering applied to the satellite data have effectively eliminated any low-frequency conflicts between the two datasets.
The first- and second-rank magnetic tensor components, forward computed by the obtained R-SCHA model at 0 km altitude, are displayed in Figure 4. Additionally, the magnetic vector and module components at altitudes of 50, 100, 200, 300, and 400 km are presented in Figure 5.
To verify the reliability of CUG_CLMFM3Dv2, we compare this regional model with three other high-resolution global spherical harmonic models: EMM2017 (SH 16–790; www.ngdc.noaa.gov/geomag/EMM/), WDMAMv2 (SH 16–800; Lesur et al., 2016), and SH1050 (SH 16–1050; Thébault et al., 2021), in both spatial and frequency domains.
Two regions are selected for spatial domain comparison. The first region spans from 70°E to 100°E and 20°N to 60°N, whereas the second region spans from 105°E to 135°E and 20°N to 60°N. The TMI data within the modeling area and the selected area are shown in Figure 6.
Comparison between model-derived data and the magnetic anomaly map reveals that CUG_CLMFM3Dv2 exhibits a higher resolution. The morphology and amplitude of the small-scale magnetic anomalies in CUG_CLMFM3Dv2 are closer to observed data, capturing finer details with improved accuracy.
Notably, the global models mentioned above are constructed using datasets that have limitations in East Asia. According to Lesur et al. (2016) and Meyer et al. (2017), both models rely on East Asian data from Coordinating Committee for Geoscience Programmes in East and Southeast Asia (CCOP, https://ccop.asia/) and Eurasian data from National Centers for Environmental Information (NCEI, https://www.ncei.noaa.gov/), which lack aeromagnetic coverage in the southern regions of Xinjiang and Tibet areas. To compensate, these models use lower resolution, satellite-based spherical harmonic models in data-vacant areas, resulting in low-frequency characteristics and small-scale artifact oscillations. In contrast, CUG_CLMFM3Dv2 integrates high-resolution near-surface data, which supplements fine details of magnetic anomalies and, like CUG_CLMFM3Dv1, avoids small-scale oscillatory artifacts in regions previously limited to low-resolution data coverage.
Figure 7 presents spectral curves comparing localized global models and CUG_CLMFM3Dv2. The spectral analysis reveals that the regional model aligns well with global models up to spherical harmonic degrees/orders of 200 and exhibits higher energy in the medium- to high-frequency range. Whereas the global models show a sharp decay at their truncation degrees/orders, indicating no energy beyond the associated wavelengths, the CUG_CLMFM3Dv2 model demonstrates an exponential energy decrease with increasing R-SCH degree, without a sudden cutoff. This continuity suggests a more gradual and comprehensive representation of higher frequency components.
In the first version of the regional model, CUG_CLMFM3Dv1, satellite data were not sufficiently along-track filtered or leveled, as shown in Figure 8. This led to a decline in consistency with satellite-based spherical harmonic models at higher altitudes. In contrast, the second version of the regional model, CUG_CLMFM3Dv2, incorporates rigorously selected and filtered satellite data, enhancing its alignment with these models across altitudes, especially at higher altitudes.
We have updated the high-resolution lithospheric magnetic field model of China and surrounding regions, thereby addressing the issues of small- to medium-scale information loss in western China and the decrease in reliability with altitude found in the first version.
Whereas the first version relied on WDMAMv2 near-surface data, the second version incorporates the aeromagnetic map of continental China, which offers greater detail for areas such as southern Xinjiang and Tibet. An extraction and replacement scheme for unreliable low-frequency components has also been applied, enhancing consistency between near-surface and satellite data. Additionally, the first version used more than 10 years of CHAMP vector measurements, whereas the second version integrates both scalar and vector measurements from the CHAMP, Swarm Alpha and Bravo, CSES-1, and MSS-1 satellites. These datasets underwent strict selection and filtering, thereby improving the alignment of the model with global models at higher altitudes.
By jointly modeling rigorously selected and processed near-surface and satellite data through spherical cap harmonic analysis, CUG_CLMFM3Dv2 achieves high resolution and high accuracy. This model is expected to provide valuable insights across various geological and geophysical fields, as well as for engineering and military applications.
Additional Supplementary Material can be found in the online version of this article.
This work was supported by the National Natural Science Foundation of China (Grant Nos.
Alken, P., Thébault, E., Beggan, C. D., Amit, H., Aubert, J., Baerenzung, J., Bondar, T. N., Brown, W. J., Califf, S., … Zhou, B. (2021). International geomagnetic reference field: The thirteenth generation. Earth Planets Space, 73(1), 49. https://doi.org/10.1186/s40623-020-01288-x
|
An, Z. C. (1993). Spherical cap harmonic analysis of geomagnetic field for China. Acta Geophysica Sinica (in Chinese), 36(6), 753–764.
|
An, Z. C., Tan, D. H., Rotanova, N. M., and Golovkov, V. P. (1998). Spherical cap harmonic analysis of MAGSAT magnetic anomalies over Asia. Acta Geophysica Sinica (in Chinese), 41(2), 168–173.
|
An, Z. C. (2003). Spherical cap harmonic analysis of the geomagnetic residual field in China for 1950–1990. Chinese J. Geophys. (in Chinese), 46(6), 767–771.
|
Backus, G. E. (1970). Non-uniqueness of the external geomagnetic field determined by surface intensity measurements. J. Geophys. Res., 75(31), 6339–6341. https://doi.org/10.1029/ja075i031p06339
|
Blakely, R. J. (1995). Potential Theory in Gravity and Magnetic Applications. Cambridge, UK: Cambridge University Press. https://doi.org/10.1017/CBO9780511549816
|
Casotto, S., and Fantino, E. (2009). Gravitational gradients by tensor analysis with application to spherical coordinates. J. Geod., 83(7), 621–634. https://doi.org/10.1007/s00190-008-0276-z
|
Dieminger, W., Hartman, G. K., and Leitinger, R. (1996). Geomagnetic activity indices. In W. Dieminger, et al. (Eds.), The Upper Atmosphere (pp. 887–911). Berlin: Springer. https://doi.org/10.1007/978-3-642-78717-1_26
|
Djomani P., Minty B., Hutchens M., and Lane R. (2019). Total Magnetic Intensity (TMI) Grid of Australia 2019 – seventh edition – 80 m cell size. Commonwealth of Australia (Geosciences Australia), Australia. https://doi.org/10.26186/5e9cf3f2c0f1d.
|
Fan, Y. C., Cai, S. H., Pavón-Carrasco, F. J., Xiong, J. G., Deng, C. L., and Pan, Y. X. (2024). High-resolution paleomagnetic secular variation since ~13 ka from a loess section in Northwest China and a regional geomagnetic directional model for East Asia. J. Geophys. Res.: Solid Earth, 129(4), e2023JB028094. https://doi.org/10.1029/2023JB028094
|
Feng, Y., Jiang, Y., Jiang, Y., Liu, J. B., Jiang, J., Liu, Z. W., Ye, M. C., Wang, H. S., and Li, X. M. (2016). Spherical cap harmonic analysis of regional magnetic anomalies based on CHAMP satellite data. Appl. Geophys., 13(3), 561–569. https://doi.org/10.1007/s11770-016-0567-8
|
Feng, Y., Sun, H., Jiang, Y., An, Z. C., Jiang, Y., Liu, B. J., Huang, Y., Zhang, H., and Wu, Y. W. (2017). Joint establishment of the geomagnetic model for mainland China based on CHAMP satellite and surface vector data. Chinese J. Geophys. (in Chinese), 60(7), 2522–2533. https://doi.org/10.6038/cjg20170702
|
Feng, Y., Nasir, A., Li, Y. J., Zhang, J. Y., Zhang, J. X., and Huang, Y. (2023a). The study of the lithospheric magnetic field over Xinjiang and Tibet areas based on ground, airborne, and satellite data. Remote Sens., 15(8), 2002. https://doi.org/10.3390/rs15082002
|
Feng, Y., Zhang, J. X., Xiong, S. Q., Zhang, J. Y., Ou, J. M., Liu, S., Li, Y. J., and Huang, Y. (2023b). Regional modeling of the lithospheric magnetic field from local and satellite constraints: A case study of the Xinjiang region. Pure Appl. Geophys., 180(12), 4219–4235. https://doi.org/10.1007/s00024-023-03385-w
|
Finlay, C. C., Kloss, C., Olsen, N., Hammer, M. D., Tøffner-Clausen, L., Grayver, A., and Kuvshinov, A. (2020). The CHAOS-7 geomagnetic field model and observed changes in the South Atlantic Anomaly. Earth Planets Space, 72(1), 156. https://doi.org/10.1186/s40623-020-01252-9
|
Friis-Christensen, E., Lühr, H., and Hulot, G. (2006). Swarm: A constellation to study the Earth’s magnetic field. Earth Planets Space, 58(4), 351–358. https://doi.org/10.1186/bf03351933
|
Gu, Z. W., Zhan, Z. J., Gao, J. T., Han, W., An, Z. C., Yao, T. Q., and Chen, B. (2006). Geomagnetic survey and geomagnetic model research in China. Earth Planets Space, 58(6), 741–750. https://doi.org/10.1186/BF03351977
|
Jiang, Y., Holme, R., Xiong, S. Q., Jiang, Y., Feng, Y., and Yang, H. (2021). Long-wavelength lithospheric magnetic field of China. Geophys. J. Int., 224(3), 1780–1792. https://doi.org/10.1093/gji/ggaa490
|
Kan, J. R., and Lee, L. C. (1979). Energy coupling function and solar wind-magnetosphere dynamo. Geophys. Res. Lett., 6(7), 577–580. https://doi.org/10.1029/GL006i007p00577
|
Kauristie, K., Morschhauser, A., Olsen, N., Finlay, C. C., McPherron, R. L., Gjerloev, J. W., and Opgenoorth, H. J. (2017). On the usage of geomagnetic indices for data selection in internal field modelling. Space Sci. Rev., 206(1-4), 61–90. https://doi.org/10.1007/s11214-016-0301-0
|
Lesur, V., Hamoudi, M., Choi, Y., Dyment, J., and Thébault, E. (2016). Building the second version of the world digital magnetic anomaly map (WDMAM). Earth Planets Space, 68(1), 27. https://doi.org/10.1186/s40623-016-0404-6
|
Luo, Y., Li, J. M., Zheng, J. X., Yang, X., Yin, H., Zhang, W. Z., Meng, Q. K., Li, B., and Wang, L. F. (2024). Progress and challenges in modeling global lithospheric magnetic anomalies. Rev. Geophys. Planet. Phys. (in Chinese), 55(6), 681–692. https://dou.org/10.19975/j.dqyxx.2024-008
|
Maus, S. (2007). Champ. In D. Gubbins, et al. (Eds.), Encyclopedia of Geomagnetism and Paleomagnetism (pp. 59–60). Dordrecht: Springer. https://doi.org/10.1007/978-1-4020-4423-6_25
|
Meyer, B., Chulliat, A., and Saltus, R. (2017). Derivation and error analysis of the earth magnetic anomaly grid at 2 arc min resolution version 3 (EMAG2v3). Geochem. Geophys. Geosyst., 18(12), 4522–4537. https://doi.org/10.1002/2017gc007280
|
Newell, P. T., Sotirelis, T., Liou, K., Meng, C. I., and Rich, F. J. (2007). A nearly universal solar wind–magnetosphere coupling function inferred from 10 magnetospheric state variables. J. Geophys. Res.: Space Phys., 112(A1), A01206. https://doi.org/10.1029/2006JA012015
|
Olsen, N., Lühr, H., Finlay, C. C., Sabaka, T. J., Michaelis, I., Rauberg, J., and Tøffner-Clausen, L. (2014). The CHAOS-4 geomagnetic field model. Geophys. J. Int., 197(2), 815–827. https://doi.org/10.1093/gji/ggu033
|
Olsen, N., Stolle, C., Floberghagen, R., Hulot, G., and Kuvshinov, A. (2016). Special issue “Swarm science results after 2 years in space”. Earth Planets Space, 68(1), 172. https://doi.org/10.1186/s40623-016-0546-6
|
Olsen, N., Ravat, D., Finlay, C. C., and Kother, L. K. (2017). LCS-1: A high-resolution global model of the lithospheric magnetic field derived from CHAMP and Swarm satellite observations. Geophys. J. Int., 211(3), 1461–1477. https://doi.org/10.1093/gji/ggx381
|
Ou, J. M., Du, A. M., Thébault, E., Xu, W. Y., Tian, X. B., and Zhang, T. L. (2013). A high resolution lithospheric magnetic field model over China. Sci. China Earth Sci., 56(10), 1759–1768. https://doi.org/10.1007/s11430-013-4580-y
|
Pavón-Carrasco, F. J., Campuzano, S. A., Rivero-Montero, M., Molina-Cardín, A., Gómez-Paccard, M., and Osete, M. L. (2021). SCHA.DIF.4k: 4,000 years of paleomagnetic reconstruction for Europe and its application for dating. J. Geophys. Res.: Solid Earth, 126(3), e2020JB021237. https://doi.org/10.1029/2020JB021237
|
Pilkington, M. (1997). 3-D magnetic imaging using conjugate gradients. Geophysics, 62(4), 1132–1142. https://doi.org/10.1190/1.1444214
|
Ravat, D., Hildenbrand, T. G., and Roest, W. (2003). New way of processing near-surface magnetic data: The utility of the comprehensive model of the magnetic field. Lead. Edge, 22(8), 784–785. https://doi.org/10.1190/1.1605082
|
Sabaka, T. J., Tøffner-Clausen, L., Olsen, N., and Finlay, C. C. (2020). CM6: A comprehensive geomagnetic field model derived from both CHAMP and Swarm satellite observations. Earth Planets Space, 72(1), 80. https://doi.org/10.1186/s40623-020-01210-5
|
Shen, X. H., Zhang, X. M., Yuan, S. G., Wang, L. W., Cao, J. B., Huang, J. P., Zhu, X. H., Piergiorgio, P., and Dai, J. P. (2018). The state-of-the-art of the China Seismo-Electromagnetic Satellite mission. Sci. China Technol. Sci., 61(5), 634–642. https://doi.org/10.1007/s11431-018-9242-0
|
Talarn, À., Pavón-Carrasco, F. J., Torta, J. M., and Catalán, M. (2017). Evaluation of using R-SCHA to simultaneously model main field and secular variation multilevel geomagnetic data for the North Atlantic. Phys. Earth Planet. Inter., 263, 55–68. https://doi.org/10.1016/j.pepi.2016.11.008
|
Thébault, E. (2003). Modélisation régionale du champ magnétique terrestre. Strasbourg, France: Université Louis Pasteur.
|
Thébault, E., Schott, J. J., Mandea, M., and Hoffbeck, J. P. (2004). A new proposal for spherical cap harmonic modelling. Geophys. J. Int., 159(1), 83–103. https://doi.org/10.1111/j.1365-246X.2004.02361.x
|
Thébault, E., Schott, J. J., and Mandea, M. (2006a). Revised spherical cap harmonic analysis (R-SCHA): Validation and properties. J. Geophys. Res.: Solid Earth, 111(B1), B01102. https://doi.org/10.1029/2005jb003836
|
Thébault, E., Mandea, M., and Schott, J. J. (2006b). Modeling the lithospheric magnetic field over France by means of revised spherical cap harmonic analysis (R-SCHA). J. Geophys. Res.: Solid Earth, 111(B5), B05102, https://doi.org/10.1029/2005JB004110
|
Thébault, E., Lesur, V., Kauristie, K., and Shore, R. (2017). Magnetic field data correction in space for modelling the lithospheric magnetic field. Space Sci. Rev., 206(1-4), 191–223. https://doi.org/10.1007/s11214-016-0309-5
|
Thébault, E., Hulot, G., Langlais, B., and Vigneron, P. (2021). A spherical harmonic model of Earth’s lithospheric magnetic field up to degree 1050. Geophys. Res. Lett., 48(21), e2021GL095147. https://doi.org/10.1029/2021GL095147
|
van Der Vorst, H. A. (1992). Bi-CGSTAB: A fast and smoothly converging variant of Bi-CG for the solution of nonsymmetric linear systems. SIAM J. Sci. Stat. Comput., 13(2), 631–644. https://doi.org/10.1137/0913035
|
Vervelidou, F., and Thébault, E. (2015). Global maps of the magnetic thickness and magnetization of the Earth’s lithosphere. Earth Planets Space, 67(1), 173, https://doi.org/10.1186/s40623-015-0329-5
|
Vervelidou, F., Thébault, E., and Korte, M. (2018). A high-resolution lithospheric magnetic field model over southern Africa based on a joint inversion of CHAMP, Swarm, WDMAM, and ground magnetic field data. Solid Earth, 9(4), 897–910. https://doi.org/10.5194/se-9-897-2018
|
Wang, J., Shen, X. H., Yang, Y. Y., Zeren, Z. M., Hulot, G., Olsen, N., Zhou, B., Magnes, W., De Santis, A., … Yu, J. B. (2021). Initial scalar lithospheric magnetic anomaly map of China and surrounding regions derived from CSES satellite data. Sci. China Technol. Sci., 64(5), 1118–1126. https://doi.org/10.1007/s11431-020-1727-0
|
Wang, J., Shen, X. H., Yang Y. Y., Zeren, Z. M., Huang, J. P., Zhao, S. F., Wang, Q., Lu, H. X., and Guo, F. (2023). The spherical cap harmonic model of lithospheric magnetic anomaly in Chinese region derived from CSES satellite data. Chinese J. Geophys. (in Chinese), 66(7), 2973–2982. https://doi.org/10.6038/cjg2022Q0857
|
Wang, J., Yang, Y. Y., Zeren, Z., Huang, J. P., and Lu, H. X. (2025). Evaluation of influence of detrending CSES satellite data on lithospheric magnetic field modeling. Earth Planet. Phys., 9(2), 346–356. https://doi.org/10.26464/epp2024068
|
Xiong, S. Q., Tong, J., Ding, Y. Y., and Li, Z. K. (2016). Aeromagnetic data and geological structure of continental China: A review. Appl. Geophys., 13(2), 227–237. https://doi.org/10.1007/s11770-016-0552-2
|
Yang, Y. Y., Zhou, B., Hulot, G., Olsen, N., Wu, Y. Y., Xiong, C., Stolle, C., Zeren, Z. M., Huang, J. P., .. Shen, X. H. (2021). CSES high precision magnetometer data products and example study of an intense geomagnetic storm. J. Geophys. Res.: Space Phys., 126(4), e2020JA028026. https://doi.org/10.1029/2020JA028026
|
Zhang, K. (2023). A novel geomagnetic satellite constellation: Science and applications. Earth Planet. Phys., 7(1), 4–21. https://doi.org/10.26464/epp2023019
|
Zhang, P., Du, J. S., Wang, Z., Yang, M. Y., and Chen, C. (2022). Extraction, evaluation and replacement techniques of long wavelength components from compiled regional aeromagnetic anomaly data. Chinese J. Geophys. (in Chinese), 65(7), 2595–2612. https://doi.org/10.6038/cjg2022P0776
|
Zhang, P., Du, J. S., and Li, H. P. (2024). CUG_CLMFM3Dv1: A high-resolution revised spherical cap harmonic model of three-dimensional lithospheric magnetic field over China and surroundings. Chinese J. Geophys. (in Chinese), 67(5), 1866–1880. https://doi.org/10.6038/cjg2023Q0856
|
Satellite | TMI data | |||
Number of data | Altitude range (km) | Standard deviation (nT) | ||
CHAMP | 194 to 480 | ±3.28 | ||
CSES-1 | 5157 | 493 to 521 | ±1.74 | |
MSS-1 | 419 to 482 | ±2.15 | ||
Swarm Alpha | 422 to 503 | ±2.15 | ||
Swarm Bravo | 494 to 522 | ±1.73 | ||
Satellite | Vector data | |||
Number of data (vector triplets) | Altitude range (km) | Standard deviation (nT) | ||
CHAMP | Bx | 245 to 481 | ±3.39 | |
By | ±3.73 | |||
Bz | ±3.62 | |||
MSS-1 | Bx | 419 to 482 | ±1.86 | |
By | ±1.40 | |||
Bz | ±2.27 | |||
Swarm Alpha | Bx | 422 to 503 | ±2.20 | |
By | ±3.73 | |||
Bz | ±2.33 | |||
Swarm Bravo | Bx | 494 to 522 | ±1.91 | |
By | ±3.56 | |||
Bz | ±1.90 |
Data source | Data type | Minimum (nT) | Maximum (nT) | Mean (nT) | RMSE (nT) |
Near surface | TMI | −436.69 | 822.98 | 3.12 × 10−2 | ±12.04 |
CHAMP | TMI | −13.08 | 11.07 | 6.56 × 10−4 | ±0.54 |
Vector Bx | −49.58 | 45.02 | 1.11 × 10−3 | ±2.19 | |
Vector By | −43.83 | 45.18 | −3.24 × 10−4 | ±3.04 | |
Vector Bz | −26.97 | 22.00 | −7.42 × 10−3 | ±1.23 | |
CSES HPM6 | TMI | −6.36 | 10.34 | 2.08 × 10−3 | ±0.33 |
MSS-1 | TMI | −1.35 | 2.12 | −1.42 × 10−4 | ±0.13 |
Vector Bx | −2.16 | 3.27 | 1.28 × 10−3 | ±0.26 | |
Vector By | −5.48 | 9.04 | −1.69 × 10−2 | ±0.37 | |
Vector Bz | −5.08 | 6.01 | 3.20 × 10−4 | ±0.29 | |
Swarm Alpha | TMI | −14.10 | 13.32 | 8.12 × 10−4 | ±0.46 |
Vector Bx | −25.16 | 39.22 | 2.38 × 10−3 | ±1.42 | |
Vector By | −48.19 | 47.98 | −2.74 × 10−3 | ±3.46 | |
Vector Bz | −11.32 | 15.39 | 6.30 × 10−4 | ±0.71 | |
Swarm Bravo | TMI | −8.41 | 6.92 | −3.66 × 10−4 | ±0.35 |
Vector Bx | −28.46 | 27.45 | −2.85 × 10−3 | ±1.34 | |
Vector By | −47.87 | 49.36 | 8.23 × 10−4 | ±3.37 | |
Vector Bz | −18.50 | 23.02 | −2.34 × 10−3 | ±0.64 | |
aRMSE, root mean square error; HPM6, High-Precision Magnetometer No.6. |