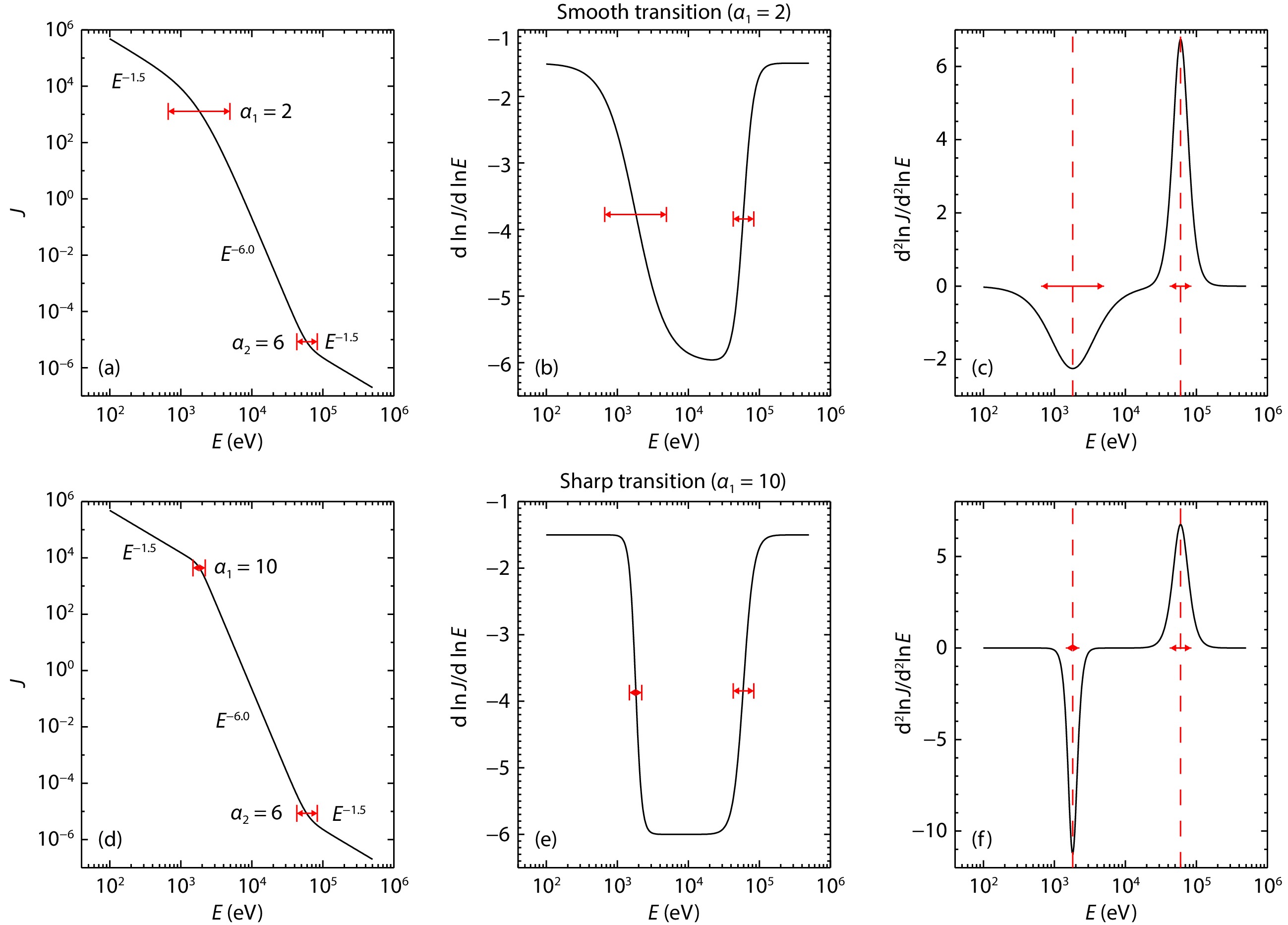
Citation: | Li, W. Y., Wang, L. H., Wang, Y. F., Guo, X. N., and Ma, Q. Y. (2025). Extended pan-spectrum fitting of energetic particle spectra. Earth Planet. Phys., 9(2), 366–374. DOI: 10.26464/epp2025005 |
The energy spectrum of energetic particles in space often shows a non-thermal spectral shape with two spectral transitions/breaks over a wide energy range, carrying crucial information about their acceleration, release and transportation process. To self-consistently characterize the spectral features of energetic particles, here we propose a novel extended pan-spectrum (EPS) formula to fit the particle energy-flux spectrum, which has the merit that can incorporate many commonly used spectrum functions with one spectral transition, including the pan-spectrum, double-power-law, Kappa, Ellison-Ramaty (ER) functions, etc. The formula can also determine the spectral shape with two spectral transitions, including the triple-power-law function, Kappa distribution (at low energy) plus power law (at high energy), power law (at low energy) plus ER function, etc. Considering the uncertainties in both J and E, we can fit this EPS formula well to the representative energy spectra of various particle phenomena in space, including solar energetic particles (electrons, protons, 3He and heavier ions), anomalous cosmic rays, solar wind suprathermal particles (halo and superhalo electrons; pick-up ions and the suprathermal tail), etc. Therefore, the EPS fitting can help us self-consistently determine the spectral features of different particle phenomena, and improve our understanding of the physical nature of the origin, acceleration, and transportation of energetic particles in space.
Energetic particles are commonly observed in space, including solar energetic particles (SEPs) (Ellison and Ramaty, 1985; Lin RP, 1985; Lin RP and Schwartz, 1987; Mason et al., 2002; Mewaldt et al., 2005; Wang LH et al., 2006, 2022; Krucker et al., 2009; Wang XD et al., 2022; Wei WW et al., 2022), solar wind suprathermal particles (Lin RP, 1998; Gloeckler, 2003; Mason and Gloeckler, 2012; Yoon et al., 2012; Wang LH et al., 2015, 2022; Tao JW et al., 2016, 2021), galactic cosmic rays (Cummings et al., 2016), anomalous cosmic rays (ACRs) (Senanayake et al., 2015), energetic particles in magnetosphere (Zong Q-G et al., 2017; Ye YG et al., 2021; Chen JL et al., 2024), etc. The space-borne and ground observations suggest that, in a wide energy range, these energy particle populations show a non-thermal spectrum of particle intensity versus energy, often with one or two spectral transitions/breaks (Lin RP and Schwartz, 1987; Krucker et al., 2009; Wang LH, 2022). For example, Lin RP (1985) reported that the SEP electrons exhibited a power-law flux energy spectrum bending upward at energies above ~10 keV, then turning downward at energies above ~100 keV. The observed energy spectral shape can reflect the physical nature of these energetic particles’ origins, acceleration, and transportation processes.
It is customary to characterize the spectral features by fitting the observed particle energy spectrum to a parameterized formula. However, many previous studies obtained the spectral features by fitting the energetic particle observation to a function with an assumed spectral shape involving no more than one spectral transition. For instance, the SEP electrons (Wang LH et al., 2006; Krucker et al., 2009) were often fitted to a single-power-law (SPL) energy spectrum expressed as
J(E)={A×(EE0)−β1,E<E0A×(EE0)−β2,E>E0, | (1) |
where E is the particle energy, J is the particle intensity (flux or fluence), A is the amplitude,
J(E)=A×E−βe−EEc, |
where
J(E)=En0πm1/2e(2κW0)3/2Γ(κ+1)Γ(κ−12)Γ(32)(1+EκW0)−(κ+1), | (2) |
with the Kappa index
Recently, Liu ZX et al. (2020) constructed a pan-spectrum fitting formula with continuous energy derivatives of J at any orders, which incorporates a generalized DPL function, SPL function, ER function, Kappa function, Maxwellian function, logarithmic-parabola (LP) function, etc. Therefore, the pan-spectrum formula can be utilized for self-consistently determining the spectral shape of energetic/suprathermal particles with no more than one spectral transition/break, as well as characterizing the sharpness/width of a spectral transition. Furthermore, they improved the spectrum fitting method by considering the uncertainties in both particle intensity and energy.
In this paper, we further propose an extended pan-spectrum fitting formula with eight parameters, in order to self-consistently determine the spectral shape of energetic particles over a wide energy range with two spectral transitions/breaks. Moreover, we develop a fitting method that can help to comparatively investigate the spectral properties between various energetic particle phenomena.
To fit the single-break energy spectra, Liu ZX et al. (2020) proposed a pan-spectrum formula,
J(E)=A×E−β1[1+(EE0)α]β1−β2α, |
integrated from
dlnJdlnE=−β1+β22+β1−β22tanh(α(lnE−lnE0)2), |
with continuous derivatives at any orders. It’s noted that the pan-spectrum formula was so named because it can incorporate many previously used spectrum formulae, typically with a single break energy, including classical DPL, ER, Kappa, Maxwellian function, etc., as special cases.
In order to achieve a full-range energy spectrum fitting for the common situations of one or two break energies, we extend the formalism of pan-spectrum to a formula named the “Extended pan-spectrum (EPS) Formula”, that can fit a spectral shape with 0, 1, or 2 spectral transitions/breaks. The EPS formula simultaneously meets the following requirements: (1) it can be divided into three segments clearly; (2) it can merge the three segments into one or two parts. We utilize two smooth tanh functions at break energies:
dlnJdlnE=−β1+β32+β1−β22tanh(α1(lnE−lnE1)2)+β2−β32tanh(α2(lnE−lnE2)2). | (3) |
Based on the property that when
dlnJdlnE={−β1,E≪E1−β2,E1≪E≪E2−β3,E≫E2. | (4) |
After integrating Equation (3) over the energy E, we obtain the formula of
J(E)=A×E−β1[1+(EE1)α1]β1−β2α1[1+(EE2)α2]β2−β3α2, | (5) |
where A is the amplitude coefficient,
(1) Generalized triple-power-law (TPL)
The formula can be rewritten as a continuous function (Wang W et al., 2023):
J(E)∝{E−β1[1+(EE1)α1]β1−β2α1[1+(EE2)α2]β2−β3α2,E<E1e−2α1E−β1+β22[(EE1)α12+(EE1)−α12]β1−β2α1[1+(EE2)α2]β2−β3α2,E1e−2α1≤E≤E1e2α1E−β2[1+(EE1)−α1]β1−β2α1[1+(EE2)α2]β2−β3α2,E1e2α1<E<E2e−2α2E−β2+β32[1+(EE1)−α1]β1−β2α1[(EE2)α22+(EE2)−α22]β2−β3α2,E2e−2α2≤E≤E2e2α2E−β3[1+(EE1)−α1]β1−β2α1[1+(EE2)−α2]β2−β3α2,E>E2e2α2 | (6) |
where the three segments are connected by two smooth transitions (when
[E1e−2α1,E1e2α1]and[E2e−2α2,E2e2α2]. |
When both
limα1→∞α2→∞J(E)={A×(EE1)−β1,(EE1)α1≪1A×(EE1)−β2,(EE2)α2≪1≪(EE1)α1A×(EE1)−β3,(EE2)α2≫1. | (7) |
(2) Kappa+SPL
When
limβ1=−1α1=1J(E)∝{E(1+EE1)−(β2+1)[1+(EE2)α2]β2−β3α2,EE2<e−2α2E1+β22−β32(1+EE1)−(β2+1)[(EE2)α22+(EE2)−α22]β2−β3α2,e−2α2≤EE2<e2α2E−β3E(1+β2)(1+EE1)−(β2+1)[1+(EE2)α2]β2−β3α2,EE2>e2α2 | (8) |
At low energies,
limβ1=−1,α1=1E2≫E1J(E)∝{E(1+EE1)−(β2+1),E≤E2,E−β3,E>E2. | (9) |
(3) SPL+ER
When
limβ3→∞E2→∞J(E)=A×E−β1[1+(EE1)α1]β1−β2α1×e−(EEc)α2,Ec=E2(α2β3−β2)1α2, | (10) |
where
limβ3→∞E2→∞J(E)∝{E−β1[1+(EE1)α1]β1−β2α1×e−(EEc)α2,EE1<e−2α1E−β2+β32[(EE1)α12+(EE1)−α12]β1−β2α1×e−(EEc)α2,e−2α1≤EE1<e2α1E−β2e−(EEc)α2[1+(EE1)α1]β1−β2α1,EE1>e2α1 | (11) |
when
limβ3→∞,E2→∞E1≪EcJ(E)∝{E−β1,E≪E1,E−β2e−(EEc)α2,E≫E1. | (12) |
(4) LP+LP
When
limα2→0J(E)=A×E−β1[1+(EE1)α1]β1−β2α1Eβ2−β32eα2(β2−β3)ln2E8. | (13) |
For simplicity, we use
φ(αi,E)=αi(βi−βi+1)8ln2E,i=1,2 |
to refer to the quadratic term of Taylor expression. In Equation (13),
eα2(β2−β3)ln2E8 |
is rewritten as
Limα2→0J(E)∝{E−β1[1+(EE1)α1]β1−β2α1Eβ2−β32eφ(α2,E),EE1<e−2α1E−β2+β32[(EE1)α12+(EE1)−α12]β1−β2α1Eβ2−β32eφ(α2,E),e−2α1≤EE1<e2α1E−β2[1+(EE1)−α1]β1−β2α1Eβ2−β32eφ(α2,E).EE1>e2α1 | (14) |
When
limα1→∞α2→0J(E)∝{E−β1+β2−β32eφ(α2,E),E≪E1,E−β2+β32eφ(α2,E),E≫E1, | (15) |
that is a combination of two logarithmic parabola at energies below or above
On one hand, when
J(E)=A×E−β1[1+(EE1)α1]β1−β2α1, |
which can fit well to a one- or two-segment spectrum. On the other hand, when
limα1→0α2→0J(E)∝E−β1+β32eφ(α1,E)+φ(α2,E) | (16) |
or the form:
limα1→0α2→0lnJ(E)=−β1+β32lnE+φ(α1,E)+φ(α2,E)+constant, | (17) |
with an infinite transition energy interval of [0,
To summarize, the EPS formula can cover several formulae mentioned above (Equations (16)−(17)), under different limiting approximations, representing a powerful and versatile, generalized formula for interplanetary energetic spectra.
Suggested by Liu ZX et al. (2020), we utilize the non-linear least-square algorithms to fit a series of n data points
In this study, for a serious of n data points (
{\chi }_{{\nu }}^{2}\left(\boldsymbol {a}\right)=\frac{1}{n-m}{\sum _{i=1}^{n}}\frac{{\left({J}_{i}-f\left({E}_{i},a\right)\right)}^{2}}{{{\sigma }}_{{J}_{i}}^{2}+{f}_{{E}_{i}}^{\;'2}{{\sigma }}_{{E}_{i}}^{2}}, | (18) |
where
We utilize the Broyden-Fletcher-Goldfarb-Shanno (BFGS) algorithm (Broyden, 1970; Fletcher, 1987) to find the minimum of
Step (1) starts with a given initial estimate
Step (2) calculates the gradient
Step (3) obtains the searching direction by solving the quasi-Newton equation,
{\boldsymbol {B}}_{k}{\boldsymbol {p}}_{k}=-\nabla f\left({\boldsymbol {a}}_{k}\right), | (19) |
where
Step (4) performs a one-dimensional optimization (line search) to find an acceptable step size αk in the direction obtained in Step (3). αk =
Step (5) sets
Step (6) calculates
{\boldsymbol {B}}_{k+1}={\boldsymbol {B}}_{k}+\frac{{ {{\boldsymbol{y}}}}_{k}{ {{\boldsymbol{y}}}}_{k}^{\text{T}}}{{ {{\boldsymbol{y}}}}_{k}^{\text{T}}{\boldsymbol {s}}_{k}}-\frac{{\boldsymbol {B}}_{k}{\boldsymbol {s}}_{k}{\boldsymbol {B}}_{k}^{{\mathrm{T}}}{\boldsymbol {s}}_{k}^{{\mathrm{T}}}}{{\boldsymbol {s}}_{k}^{T}{\boldsymbol {B}}_{k}{\boldsymbol {s}}_{k}},{ {{\boldsymbol{y}}}}_{k}=\nabla f\left({\boldsymbol {a}}_{k+1}\right)-\nabla f\left({\boldsymbol {a}}_{k}\right). | (20) |
Step (7) takes
Finally, the best fit
{\chi }^{2}=\left(n-m\right){\chi }_{{\nu }}^{2},C={\left(\dfrac{\boldsymbol {H}}{2}\right)}^{-1} |
and
{\boldsymbol {H}}_{ij}=\left(n-m\right)\dfrac{\partial {\chi }_{{\nu }}^{2}}{\partial {a}_{i}\partial {a}_{j}}. |
The fitted
{\delta }{\boldsymbol {a}}_{fit}^{i}=\sqrt{{\boldsymbol {C}}_{ii}}. |
Besides using
{\mathrm{ECVI}} =\dfrac{n-m}{n-1}{\chi }_{{\nu }}^{2}+\dfrac{2m}{n} |
(Expected Cross-Validation Index) as a model selection index and a smaller ECVI indicates a better fit model (Liu ZX et al., 2020).
We utilize parametric bootstrap (Efron, 1992; MacKenzie et al., 2017) to characterize the uncertainties of parameter estimation and test the correlations between parameters. Parametric bootstrap is based on the assumption that the initial data set is from a distribution of a specific parametric type. In this paper, the parametric model
In this study, given an initial parameter vector
Figure 2 is the bootstrap simulation results for EPS formula with all eight parameters effective, one of which is closest to the initial parameters, shown in panel (b). The simulated
In addition, Figure 3 plots the scatter diagrams between fitted parameters, also indicating that the averaged parameters (shown as green horizontal and vertical solid lines) agree with the initial parameters (shown as purple stars). We note: (1) the strong positive correlation between
We apply the fitting method to the energy spectra of various representative particle phenomena that achieve the best and most reliable fit, with reasonable parameters, acceptable uncertainty estimations, as well as randomly distributed residuals. As for the fitting uncertainties, since the measurements given by different instruments behave differently, here we use both
Figure 4 and Table 1 show the fitted results of several typical particle phenomena. In Figure 4a, solar energetic electrons (SEEs) (Lin RP, 1985) are fitted to a strict TPL with two sharp transitions, that can be interpreted as a power-law of
{\beta }_{1} | {\beta }_{2} | {\beta }_{3} | {E}_{1} | {E}_{2} | {\alpha }_{1} | {\alpha }_{2} | ||
SEE | 2.8 ± 0.2 | 1.24 ± 0.04 | 2.72 ± 0.08 | 6.1 ± 0.6 keV | 100 ± 8 keV | 30a | 25a | |
SEP O+ | 1.9 ± 0.3 | 0.3 ± 0.3 | 3.4 ± 0.3 | 0.39 ± 0.06 MeV/nuc | 4.3 ± 0.6 MeV/nuc | 6.2 ± 5.3 | 1.2 ± 0.5 | |
Halo & superhalo solar wind | −1a | 6.8 ± 0.4 | 2.74 ± 0.04 | 0.25 ± 0.03 keV | 2.6±0.2 keV | 1a | 10 ± 5.5 | |
SIR ions | 4.2 ± 0.2 | 1.43 ± 0.09 | infinity | 0.56 ± 0.04 MeV/nuc | infinity | 3.8 ± 1.1 | 2.3 ± 0.1 | |
PUI & suprathermal tail | H+ | 0.1 ± 0.1 | 7.7 ± 0.4 | 2.37 ± 0.01 | 2.86 ± 0.04 keV | 4.7 ± 0.1 keV/nuc | 102a | 57a |
He+ | 0.59 ± 0.08 | 6.8 ± 0.4 | 3.27 ± 0.07 | 2.66 ± 0.03 keV | 4.5 ± 0.3 keV/nuc | 56a | 70a | |
He2+ | 4.20 ± 0.07 | 2.24 ± 0.06 | 2.90 ± 0.07 | 4.7 ± 0.2 keV | 12.3 ± 0.7 keV/nuc | 89a | 77a | |
ACR | He | 2.08 ± 0.04 | 0.28 ± 0.05 | 2.8 ± 0.4 | 12.8 ± 0.4 keV/nuc | 53 ± 3 keV/nuc | 37a | 27a |
O | 1.9 ± 0.5 | −0.9 ± 1.3 | 4.1 ± 0.7 | 4.2 ± 0.1 keV/nuc | 11 ± 2 keV/nuc | 20a | 2.0 ± 1.0 | |
Note: a Parameters with no uncertainties indicate the data points are fitted under limiting approximations. |
In this study, we construct an EPS formula (Equation (5)) to self-consistently determine the spectral shape of energetic particles over a wide energy range that often show two spectral transitions/breaks. The EPS formula has eight parameters: the amplitude coefficient A; central energy E1 and E2 of low-energy and high-energy spectral transition; sharpness parameter
This EPS formula can be rewritten as a piecewise form of generalized TPL. When
Considering the uncertainties in both E and J, we utilize the BFGS algorithm (Broyden, 1970; Fletcher, 1987) in order to minimize
This research is supported in part by NSFC under contracts
Bevington, P. R., and Robinson, D. K. (2003). Data Reduction and Error Analysis for the Physical Sciences (3rd ed, pp. 2383−2402). Boston: McGraw-Hill.
|
Broyden, C. G. (1970). The convergence of a class of double-rank minimization algorithms 1. General considerations. IMA J. Appl. Math., 6(1), 76–90. https://doi.org/10.1093/imamat/6.1.76
|
Chen, J. L., Zou, H., and Ye, Y. G. (2024). Explaining the dynamics of the sub-relativistic electron third belt in the Earth’s radiation belts by using medium Earth orbit satellite observations. Earth Planet. Phys., 8(5), 762–775. https://doi.org/10.26464/epp2024054
|
Cummings, A. C., Stone, E. C., Heikkila, B. C., Lal, N., Webber, W. R., Jóhannesson, G., Moskalenko, I. V., Orlando, E., and Porter, T. A. (2016). Galactic cosmic rays in the local interstellar medium: Voyager 1 observations and model results. Astrophys. J., 831(1), 18. https://doi.org/10.3847/0004-637X/831/1/18
|
Efron, B. (1992). Bootstrap methods: another look at the jackknife. In: S. Kotz, et al. (Eds.), Breakthroughs in Statistics: Methodology and Distribution (pp. 569−593). New York: Springer. https://doi.org/10.1007/978-1-4612-4380-9_41
|
Ellison, D. C., and Ramaty, R. (1985). Shock acceleration of electrons and ions in solar flares. Astrophys. J., 298, 400–408. https://doi.org/10.1086/163623
|
Fletcher, R. (1987). Practical Methods of Optimization (2nd ed). Chichester: John Wiley & Sons. https://doi.org/10.1002/9781118723203
|
Gloeckler, G. (2003). Ubiquitous suprathermal tails on the solar wind and pickup ion distributions. AIP Conf. Proc., 679(1), 583–588. https://doi.org/10.1063/1.1618663
|
Guo, X. N., Wang, L. H., Li, W. Y., Ma, Q. Y., Yang, L., Wimmer-Schweingruber, R. F., and Bale, S. D. (2024). Evolution of electron acceleration by corotating interaction region shocks at 1 AU. Astrophys. J., 966(1), L12. https://doi.org/10.3847/2041-8213/ad3d5f
|
Krucker, S., Oakley, P. H., and Lin, R. P. (2009). Spectra of solar impulsive electron events observed near Earth. Astrophys. J., 691(1), 806–810. https://doi.org/10.1088/0004-637X/691/1/806
|
Lin, R. P. (1985). Energetic solar electrons in the interplanetary medium. Sol. Phys., 100(1-2), 537–561. https://doi.org/10.1007/BF00158444
|
Lin, R. P., and Schwartz, R. A. (1987). High spectral resolution measurements of a solar flare hard X-ray burst. Astrophys. J., 312, 462. https://doi.org/10.1086/164891
|
Lin, R. P. (1998). WIND observations of suprathermal electrons in the interplanetary medium. Space Sci. Rev., 82(1-2), 61–78. https://doi.org/10.1023/A:1005048428480
|
Liu, Z. X., Wang, L. H., Wimmer-Schweingruber, R. F., Krucker, S., and Mason, G. M. (2020). Pan-spectrum fitting formula for suprathermal particles. J. Geophys. Res.: Space Phys., 125(12), e2020JA028702. https://doi.org/10.1029/2020JA028702
|
MacKenzie, D. I., Nichols, J. D., Royle, J. A., Pollock, K. H., Bailey, L., and Hines, J. E. (2017). Occupancy Estimation and Modeling: Inferring Patterns and Dynamics of Species Occurrence (pp. 115−215). Amsterdam: Elsevier. https://doi.org/10.1016/C2012-0-01164-7
|
Mason, G. M., Wiedenbeck, M. E., Miller, J. A., Mazur, J. E., Christian, E. R., Cohen, C. M. S., Cummings, A. C., Dwyer, J. R., Gold, R. E., … Von Rosenvinge, T. T. (2002). Spectral properties of He and heavy ions in 3He-rich solar flares. Astrophys. J., 574(2), 1039–1058. https://doi.org/10.1086/341112
|
Mason, G. M., and Gloeckler, G. (2012). Power law distributions of suprathermal ions in the quiet solar wind. Space Sci. Rev., 172(1-4), 241–251. https://doi.org/10.1007/s11214-010-9741-0
|
Mewaldt, R. A., Cohen, C. M. S., Labrador, A. W., Leske, R. A., Mason, G. M., Desai, M. I., Looper, M. D., Mazur, J. E., Selesnick, R. S., and Haggerty, D. K. (2005). Proton, helium, and electron spectra during the large solar particle events of October–November 2003. J. Geophys. Res.: Space Phys., 110(A9), A09S18. https://doi.org/10.1029/2005JA011038
|
Press, W. H., Teukolsky, S. A., Vetterling, W. T., and Flannery, B. P. (2007). Numerical Recipes: The art of Scientific Computing (3rd ed). Cambridge: Cambridge University Press.
|
Senanayake, U. K., Florinski, V., Cummings, A. C., and Stone, E. C. (2015). Spectral evolution of anomalous cosmic rays at voyager 1 beyond the termination shock. Astrophys. J., 804(1), 12. https://doi.org/10.1088/0004-637X/804/1/12
|
Tao, J. W., Wang, L. H., Zong, Q. G., Li, G., Salem, C. S., Wimmer-Schweingruber, R. F., He, J. S., Tu, C. Y., and Bale, S. D. (2016). Quiet-time suprathermal (~0.1−1.5 keV) electrons in the solar wind. Astrophys. J., 820(1), 22. https://doi.org/10.3847/0004-637X/820/1/22
|
Tao, J. W., Wang, L. H., Li, G., Wimmer-Schweingruber, R. F., Salem, C., Jian, L. K., and Bale, S. D. (2021). Solar wind ~0.15−1.5 keV electrons around corotating interaction regions at 1 AU. Astrophys. J., 922(2), 198. https://doi.org/10.3847/1538-4357/ac2505
|
Wang, L. H., Lin, R. P., Krucker, S., and Gosling, J. T. (2006). Evidence for double injections in scatter-free solar impulsive electron events. Geophys. Res. Lett., 33(3), L03106. https://doi.org/10.1029/2005GL024434
|
Wang, L. H., Yang. L., He, J. S., Tu, C. Y., Pei, Z. T., Wimmer-Schweingruber, R. F., and Bale, S. D. (2015). Solar wind ~20−200 keV superhalo electrons at quiet times. Astrophys. J. Lett., 803(1), L2. https://doi.org/10.1088/2041-8205/803/1/L2
|
Wang, L. H. (2022). Interplanetary energetic electrons observed in Earth’s polar cusp/cap/lobes. Rev. Mod. Plasma Phys., 6, 12. https://doi.org/10.1007/s41614-022-00073-5
|
Wang, W., Wang, L. H., Krucker, S., and Wimmer-Schweingruber, R. F. (2023). Energy spectrum of solar energetic electron events over 25 years. Astrophys. J., 948(1), 51. https://doi.org/10.3847/1538-4357/acbea2
|
Wei, W. W., Zhuang, B., Huang, J., Shen, F., Zhao, L. L., Liu, M. Z., Zhang, X. X., and Feng, X. S. (2022). Energy spectra variations of energetic ions associated with a stream interaction region. J. Geophys. Res.: Space Phys., 127(10), e2022JA030652. https://doi.org/10.1029/2022JA030652
|
Wang, X. D., Klecker, B., Nicolaou, G., Barabash, S., Wieser, M., Wurz, P., Galli, A., Cipriani, F., and Futaana, Y. (2022). Neutralized solar energetic particles for SEP forecasting: Feasibility study of an innovative technique for space weather applications. Earth Planet. Phys., 6(1), 42–51. https://doi.org/10.26464/epp2022003
|
Ye, Y. G., Zou, H., Zong, Q.-G., Chen, H. F., Zou, J. Q., Shi, W. H., Yu, X. Q., Zhong, W. Y., Wang, Y. F., .. Hao, X. Y. (2021). Energetic electron detection packages on board Chinese navigation satellites in MEO. Earth Planet. Phys., 5(2), 158–179. https://doi.org/10.26464/epp2021021
|
Yoon, P. H., Ziebell, L. F., Gaelzer, R., Lin, R. P., and Wang, L. (2012). Langmuir turbulence and suprathermal electrons. Space Science Reviews, 173, 459–489. https://doi.org/10.1007/s11214-012-9867-3
|
Zong, Q.-G., Wang, Y. F., Ren, J., Zhou, X. Z., Fu, S. Y., Rankin, R., Zhang, H. (2017). Corotating drift-bounce resonance of plasmaspheric electron with poloidal ULF waves. Earth Planet. Phys.,, 1(1), 2–12. https://doi.org/10.26464/epp2017002
|
GuoHong Shen, DongHui Hou, Yuan Chang, XianGuo Zhang, HuanXin Zhang, Bin Yuan, BinQuan Zhang, Ying Sun. 2025: Neutron observations from the energetic particle detector on China’s Space Station. Earth and Planetary Physics, 9(2): 460-466. DOI: 10.26464/epp2024078 | |
YuJi Zhu, Fang Shen, Xi Luo, Yang Wang, BoFeng Tang. 2024: Solar energetic particles intensity variations associated with a tilted-dipole 3D corotating interaction region. Earth and Planetary Physics, 8(5): 797-810. DOI: 10.26464/epp2024049 | |
YingJie Zhang, TianRan Sun, Jennifer. A. Carter, WenHao Liu, Steve Sembay, ShuiNai Zhang, Li Ji, Chi Wang. 2024: Two methods for separating the magnetospheric solar wind charge exchange soft X-ray emission from the diffuse X-ray background. Earth and Planetary Physics, 8(1): 119-132. DOI: 10.26464/epp2023068 | |
Dimitra Koutroumpa. 2024: Solar wind ion charge state distributions and compound cross sections for solar wind charge exchange X-ray emission. Earth and Planetary Physics, 8(1): 105-118. DOI: 10.26464/epp2023056 | |
TaiFeng Jin, BinBin Ni, LingGao Kong, AiBing Zhang, Lei Li, Song Fu, Xing Cao, WenYa Li, BinBin Tang, LiangHai Xie, YiTeng Zhang, ShuYue Pang, XiaoTong Yun, HengLe Du, FuHao Qiao, LiMin Wang, JiJie Ma. 2023: Proton pitch angle distributions in the Martian induced magnetosphere: A survey of Tianwen-1 Mars Ion and Neutral Particle Analyzer observations. Earth and Planetary Physics, 7(5): 533-539. DOI: 10.26464/epp2023072 | |
Xiao-Dong Wang, B. Klecker, G. Nicolaou, S. Barabash, M. Wieser, P. Wurz, A. Galli, F. Cipriani, Y. Futaana. 2022: Neutralized solar energetic particles for SEP forecasting: Feasibility study of an innovative technique for space weather applications. Earth and Planetary Physics, 6(1): 42-51. DOI: 10.26464/epp2022003 | |
ChuanPeng Hou, JianSen He, Lei Zhang, Ying Wang, Die Duan. 2021: Dynamics of the charged particles released from a Sun-grazing comet in the solar corona. Earth and Planetary Physics, 5(3): 232-238. DOI: 10.26464/epp2021023 | |
ShuWen Tang, Yi Wang, HongYun Zhao, Fang Fang, Yi Qian, YongJie Zhang, HaiBo Yang, CunHui Li, Qiang Fu, Jie Kong, XiangYu Hu, Hong Su, ZhiYu Sun, YuHong Yu, BaoMing Zhang, Yu Sun, ZhiPeng Sun. 2020: Calibration of Mars Energetic Particle Analyzer (MEPA). Earth and Planetary Physics, 4(4): 355-363. DOI: 10.26464/epp2020055 | |
LingGao Kong, AiBing Zhang, Zhen Tian, XiangZhi Zheng, WenJing Wang, Bin Liu, Peter Wurz, Daniele Piazza, Adrian Etter, Bin Su, YaYa An, JianJing Ding, WenYa Li, Yong Liu, Lei Li, YiRen Li, Xu Tan, YueQiang Sun. 2020: Mars Ion and Neutral Particle Analyzer (MINPA) for Chinese Mars Exploration Mission (Tianwen-1): Design and ground calibration. Earth and Planetary Physics, 4(4): 333-344. DOI: 10.26464/epp2020053 | |
Wei Chu, JianPing Huang, XuHui Shen, Ping Wang, XinQiao Li, ZhengHua An, YanBing Xu, XiaoHua Liang. 2018: Preliminary results of the High Energetic Particle Package on-board the China Seismo-Electromagnetic Satellite. Earth and Planetary Physics, 2(6): 489-498. DOI: 10.26464/epp2018047 |
{\beta }_{1} | {\beta }_{2} | {\beta }_{3} | {E}_{1} | {E}_{2} | {\alpha }_{1} | {\alpha }_{2} | ||
SEE | 2.8 ± 0.2 | 1.24 ± 0.04 | 2.72 ± 0.08 | 6.1 ± 0.6 keV | 100 ± 8 keV | 30a | 25a | |
SEP O+ | 1.9 ± 0.3 | 0.3 ± 0.3 | 3.4 ± 0.3 | 0.39 ± 0.06 MeV/nuc | 4.3 ± 0.6 MeV/nuc | 6.2 ± 5.3 | 1.2 ± 0.5 | |
Halo & superhalo solar wind | −1a | 6.8 ± 0.4 | 2.74 ± 0.04 | 0.25 ± 0.03 keV | 2.6±0.2 keV | 1a | 10 ± 5.5 | |
SIR ions | 4.2 ± 0.2 | 1.43 ± 0.09 | infinity | 0.56 ± 0.04 MeV/nuc | infinity | 3.8 ± 1.1 | 2.3 ± 0.1 | |
PUI & suprathermal tail | H+ | 0.1 ± 0.1 | 7.7 ± 0.4 | 2.37 ± 0.01 | 2.86 ± 0.04 keV | 4.7 ± 0.1 keV/nuc | 102a | 57a |
He+ | 0.59 ± 0.08 | 6.8 ± 0.4 | 3.27 ± 0.07 | 2.66 ± 0.03 keV | 4.5 ± 0.3 keV/nuc | 56a | 70a | |
He2+ | 4.20 ± 0.07 | 2.24 ± 0.06 | 2.90 ± 0.07 | 4.7 ± 0.2 keV | 12.3 ± 0.7 keV/nuc | 89a | 77a | |
ACR | He | 2.08 ± 0.04 | 0.28 ± 0.05 | 2.8 ± 0.4 | 12.8 ± 0.4 keV/nuc | 53 ± 3 keV/nuc | 37a | 27a |
O | 1.9 ± 0.5 | −0.9 ± 1.3 | 4.1 ± 0.7 | 4.2 ± 0.1 keV/nuc | 11 ± 2 keV/nuc | 20a | 2.0 ± 1.0 | |
Note: a Parameters with no uncertainties indicate the data points are fitted under limiting approximations. |