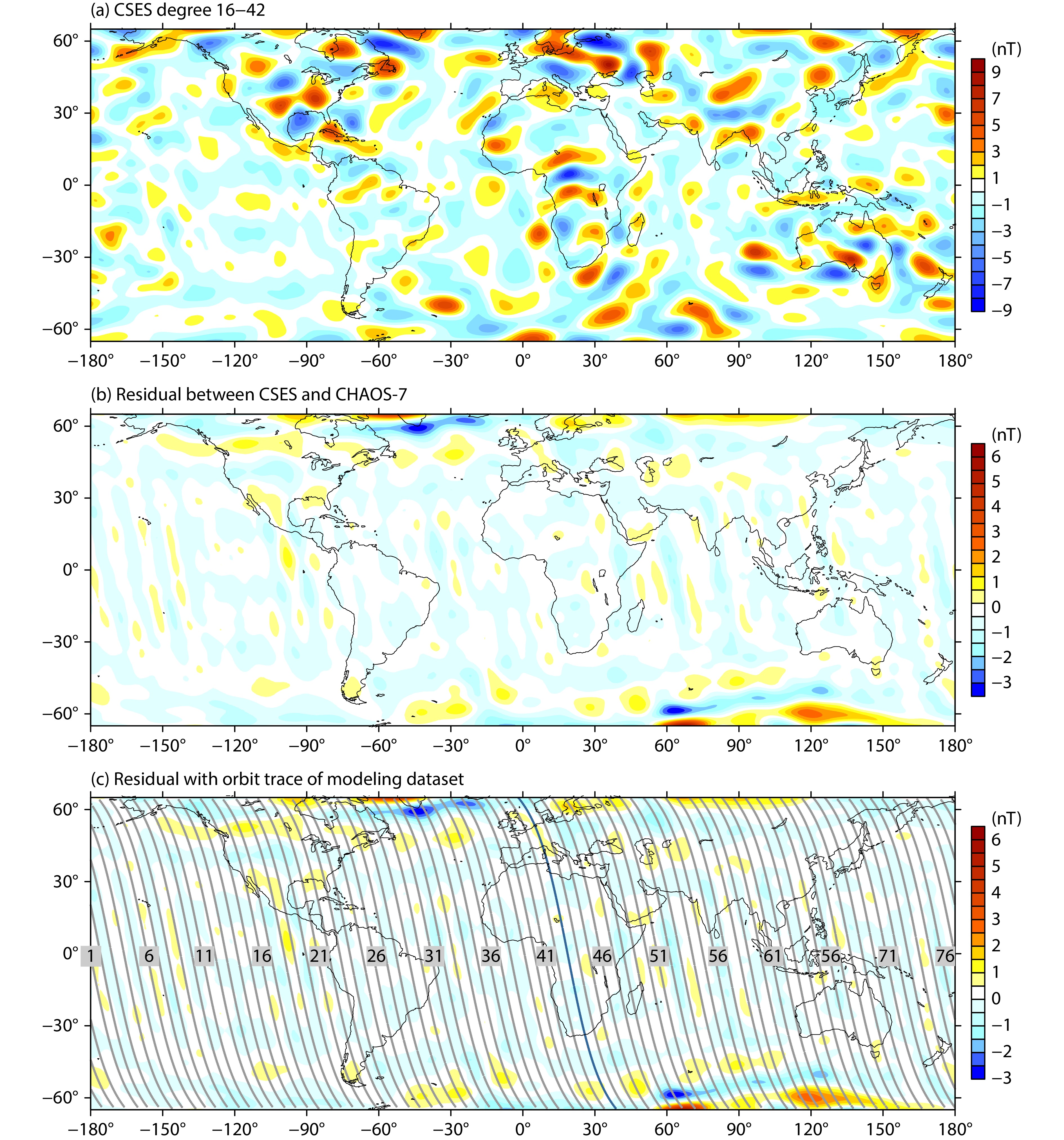
Citation: | Wang, J., Yang, Y. Y., Zeren, Z. M., Huang, J. P., and Lu, H. X. (2025). Evaluation of influence of detrending CSES satellite data on lithospheric magnetic field modeling. Earth Planet. Phys., 9(2), 346–356. DOI: 10.26464/epp2024068 |
The China Seismo-Electromagnetic Satellite (CSES) was successfully launched in February 2018. The high precision magnetometer (HPM) on board the CSES has captured high-quality magnetic data that have been used to derive a global lithospheric magnetic field model. While preparing the datasets for this lithospheric magnetic field model, researchers found that they still contained prominent residual trends within the magnetic anomaly even once signals from other sources had been eliminated. However, no processing was undertaken to deal with the residual trends during modeling to avoid subjective processing and represent the realistic nature of the data. In this work, we analyze the influence of these residual trends on the lithospheric magnetic field modeling. Polynomials of orders 0–3 were used to fit the trend of each track and remove it for detrending. We then derived four models through detrending-based processing, and compared their power spectra and grid maps with those of the CSES original model and CHAOS-7 model. The misfit between the model and the dataset decreased after detrending the data, and the convergence of the inverted spherical harmonic coefficients improved. However, detrending reduced the signal strength and the power spectrum, while detrending based on high-order polynomials introduced prominent distortions in details of the magnetic anomaly. Based on this analysis, we recommend along-track detrending by using a zero-order polynomial (removing a constant value) on the CSES magnetic anomaly data to drag its mean value to zero. This would lead to only a slight reduction in the signal strength while significantly improving the stability of the inverted coefficients and details of the anomaly.
Lithospheric magnetic anomalies quickly decrease with the altitude, and only a few magnetic anomalies with a long wavelength can still be detected onboard low-Earth-orbit satellites. Satellite magnetic anomalies are generated by large-scale magnetized rocks in the crust and locally, the uppermost mantle of the Earth (Ferré et al., 2014). Their geological origins are generally related to plate tectonics, lower crustal processes and impacts, and they reflect important information on the constitution, structure, and evolution of the crust (Purucker and Clark, 2011; Wang J et al., 2024a, b).
The American POGO (1967–1971) and Magsat (1979–1980) satellites confirmed that weak lithospheric magnetic anomalies are detectable on satellite, and generated maps of the global magnetic anomalies (Regan et al., 1975; Langel and Hinze, 1998; Arkani-Hamed et al., 1994). The Danish Ørsted (1999–2013) and German CHAMP (2000–2010) satellites provided particularly valuable data over a long period that helped develop lithospheric magnetic field modeling (Maus et al., 2002, 2007; Purucker et al., 2002; Sabaka et al., 2015). The ESA’s Swarm satellite constellation (2013–) has been designed specifically for geomagnetic field monitoring, and its data have further improved the spatial resolution of satellite-based models of the lithospheric magnetic field (Olsen et al., 2017). China has launched two satellites with the capability of magnetic detection — the China Seismo-Electromagnetic Satellite (CSES; 2018–) and the Macao Science Satellite-1 (MSS-1; 2023–). Data from the CSES have been used to derive a global model of the lithospheric magnetic field (Wang J et al., 2023a).
The lithospheric magnetic field has been modeled based on data from the CSES (Wang J et al., 2023a) by using a serial approach (Thébault et al., 2010), whereby the contributions of all other magnetic fields are sequentially eliminated by using an a priori model. However, in spite of the stringent procedures for data selection (Kauristie et al., 2017) in this sequential approach, residual signals or perturbation fields persist in the data (Finlay et al., 2017) mainly owing to the dynamic system created by the coupling of solar wind, the magnetosphere, ionosphere, and core internal magnetic fields (Thébault et al., 2017). Following the removal of the core and external fields by using the CHAOS-7 model (Finlay et al., 2020), prominent residual trends persisted within the along-track data on magnetic anomalies of the CSES. However, no processing was undertaken to deal with these residual trends to avoid subjective processing and represent the realistic nature of the data. In general, residual signals are filtered by using methods of along-track external field correction, such as Fourier filtering, polynomial filtering, high-pass spherical harmonics, singular spectral analysis, and line levelling (Thébault et al., 2012, 2017; Lesur et al., 2013). These residual signals may be introduced to the model of the lithospheric magnetic field if they are not suitably dealt with. In this work, we analyze the influence of the residual trends on lithospheric magnetic field modeling and provide suggestions on detrending the data.
The CSES satellite was launched in February 2018. It was designed to measure geophysical fields for research on space and solid earth physics (Shen XH et al., 2018; Zeren ZM et al., 2024). The CSES is on a nearly circular, near-polar, sun-synchronous orbit at an inclination angle of 97.4°. It revisits each orbit every five days, and flies at a mean altitude of 507 km above a sphere of radius 6371.2 km. It requires 76 orbits for global coverage, and each can be divided into two half-orbits: night-side (ascending node at local time 02:00) and day-side orbits (descending node at local time 14:00). Traces of the night-side orbit are shown in Figure 1c, and the orbits are numbered after every five orbits.
The high precision magnetometer (HPM) onboard the CSES carries two fluxgate magnetometers (FGM, Zhou B et al., 2019) for vector measurements, as well as a coupled dark-state magnetometer (CDSM, Pollinger et al., 2020) for scalar measurements at a sampling rate of 1 Hz. The CDSM has an accuracy of 0.19 nT (Pollinger et al., 2018). An on-ground prelaunch test showed that remaining magnetic interference from the platform at the center of the CDSM sensor was lower than 0.33 nT (Cheng BJ et al., 2018). The quality of the data captured by the HPM data has been confirmed (Yang YY et al., 2021a; Zeren ZM et al., 2022), and they can be downloaded from https://leos.ac.cn. They have been used for the modeling of the geomagnetic core field (Yang YY et al., 2021b) as well as lithospheric magnetic field (Wang J et al., 2021, 2023a, b).
Scalar magnetic data from the CSES have been used to derive a global model of the lithospheric magnetic field between the geographic latitudes of ±65° (Wang et al., 2023a). The coefficients of the model are available at https://www.leos.ac.cn/#/article/info/250. We chose night-side data from March 2018 to November 2022 under quiet space weather conditions. We then removed the core and external fields by using the CHAOS-7 model (Finlay et al., 2020). After further data quality control, we used them to build a lithospheric magnetic field model through spherical harmonic (SH) analysis. The CSES model thus obtained was compared with other important models in terms of the power spectrum and details of the anomalies. The results confirmed that scalar data from the CSES can provide a reliable model of the lithospheric magnetic field for up to SH degree 42. Figure 1 shows the map of the lithospheric magnetic anomalies, calculated by using the CSES model at 500 km, as well as the residuals compared with the CHAOS-7 model. The residuals were mostly detected along the orbit of the satellite and were caused by the gridding of a discrete dataset with large spaces in the survey line. The orbital interval of the CSES satellite was relatively large, which is 360°/76 (circles for a global coverage) ≈ 4.7°, and the residuals in the orbital gap were larger than those along the orbit. This was especially prominent in low-latitude areas, as shown in Figure 1c.
After removing the core and external fields, the magnetic anomalies exhibited a residual trend that could be represented by the along-track mean value. We calculated the mean values of magnetic anomalies in the datasets for each orbit, and the orbit numbers shown in Figure 2a are identical to those in Figure 1c. The mean values for different orbits varied and most of them were positive. The mean value of the entire dataset was 0.904 nT.
To clearly show the trend of magnetic anomalies in CSES data, we chose data on Orbit 43, which was across the famous Bangui magnetic anomaly — a prominent, low magnetic signal at 5° N latitude. A total of ninety-three revisited files for Orbit 43 have been analyzed, yielding a mean observation value of 1.849 nT, the estimation along Orbits 43, derived from the CSES lithospheric magnetic field model, is calculated to be 0.244 nT. The mean value of estimates of the model was close to zero, while the difference between the observed and estimated values indicates that there was a residual trend in the observations owing to non-lithospheric magnetic sources.
The residual trends in the data on magnetic anomalies from the CSES reflected signals from non-lithospheric magnetic sources. Because the lithospheric magnetic field originates from magnetized rocks in the crust and locally uppermost mantle of the Earth (Ferré et al., 2014), these signals are relatively stable. The residual trends might have had three possible sources: (1) remaining influence of the external field, (2) difference in satellite platforms, and (3) time-variable component of the lithospheric magnetic field. As changes in the lithospheric magnetic field were minimal during this study, they were ignored, and sources (1) and (2) above were identified as the main cause of the residual trend.
Figure 2b shows that the residual trend in each track was different, which means that it was a variable component, and this is consistent with the properties of the external field. The external field was removed before modeling by using the CHAOS-7 model, and the residual trends were mostly caused by the part of the external field that the model failed to fit. This part originated from the dynamic system created by the coupling between solar wind, the magnetosphere, ionosphere, and core internal magnetic fields (Thébault et al., 2017).
Another possible source of the residual trends might have been the difference between the satellite platforms. The data on the core field were also removed by using the CHAOS-7 model. The latter was derived from data from the Ørsted, CHAMP, SAC-C, CryoSat-2 and Swarm satellites, and did not include data from the CSES. The mean values of the CHAOS-7 model for non-polar data from these satellites are listed in Table 1 (Finlay et al., 2020). The amplitudes of misfits of data from the Ørsted and CHAMP satellites both exceeded 0.5 nT, while those of the SAC-C, CryoSat-2, and Swarm were relatively small, and no greater than 0.1 nT. This implies that there were intrinsic differences among the data from different satellites that were possibly caused by the use of inconsistent platforms and instruments.
Satellite | Mean (nT) | Satellite | Mean (nT) |
Ørsted | 0.53 | Swarm-A | −0.07 |
CHAMP | −0.53 | Swarm-B | −0.07 |
SAC-C | 0.10 | Swarm-C | 0.00 |
CryoSat-2 | 0.02 |
The potential
V=a{\sum }_{n=1}^{N}{\sum }_{m=0}^{n}\left(\frac{a}{r}\right)^{n+1}\left({g}_{n}^{m}\mathrm{cos}m\phi +{h}_{n}^{m}\mathrm{sin}m\phi \right){P}_{n}^{m}(\mathrm{cos}\theta ) , | (1) |
where
SH modeling is to estimate the Gaussian coefficients, which is equivalent to obtaining the solution to linear equations. We used the LSQR algorithm (Paige and Saunders, 1982), which is integrated into MATLAB®, to solve the large, overdetermined linear equations. This algorithm uses a damped iterative method to reliably solve ill-conditioned linear equations.
The dataset and method for modeling were the same as for the CSES original model. For detailed information on lithospheric magnetic field modeling, the interested reader can refer to Wang J et al. (2023a).
Because the residual trends vary gently in low-order terms, we used polynomials to fit the trend of each track and remove it to detrend the data. We used 0-order, 1-order, 2-order, and 3-order polynomials for along-track detrending to evaluate its influence on modeling. They can be expressed as follows:
0-order (constant) polynomial:
1-order (linear) polynomial:
2-order (quadratic) polynomial:
3-order (cubic) polynomial:
In the above, y corresponds to the trend of fitting, x represents the latitude of each track, and
The dataset used for modeling was the one used for the CSES original model (Wang J et al., 2023a). After detrending the data by using polynomials, we applied them to derive four models of the lithospheric magnetic field — Detrending-0, Detrending-1, Detrending-2, and Detrending-3 — corresponding to the modeling datasets detrended by using polynomials of orders 0–3, respectively. Table 2 lists the statistics of the CSES original model and the detrending models, including the misfits, range of field values, residuals compared with the CHAOS-7 model, and the residuals between the CSES original model and the four detrending models.
Model | Misfit (nT) | Field value (nT) | Residual with CHAOS-7 (nT) | Residual with CSES original (nT) |
CSES original | 0.0019 | (−8.52, 8.39) | (−2.90, 5.75) | − |
Detrending-0 | 0.0013 | (−8.83, 7.74) | (−3.29, 5.31) | (−1.01, 1.13) |
Detrending-1 | 0.0010 | (−8.91, 7.27) | (−3.04, 5.81) | (−2.12, 1.96) |
Detrending-2 | 0.0007 | (−7.89, 7.57) | (−3.95, 8.43) | (−3.03, 3.15) |
Detrending-3 | 0.0006 | (−7.91, 7.44) | (−4.34, 8.14) | (−3.48, 3.99) |
The misfits between the results of model estimation and the datasets are listed in Table 2. The misfits of all models were minor, with the CSES original model yielding a misfit of 0.0019 nT. As the order of the polynomials increased, the misfit of the detrending models decreased. The misfit of the Detrending-3 model was only 0.006 nT, indicating improved fitting after introducing detrending for the datasets.
Figure 4 illustrates maps of the lithospheric magnetic anomalies and their residuals with the CHAOS-7 model, with a 0.5° × 0.5° grid calculated by the CSES original model and the four detrending models at an altitude of 500 km. The ranges of values of these models and the residuals are listed in Table 2. The maximum values of the detrending models generally decreased with the order of the polynomial used, which means that detrending reduced the amplitude of the datasets. The Detrending-1 model obtained the lowest value, and its maximum value decreased to 7.27 nT, while those of the Detrending-2 (7.57 nT) and Detrending-3 (7.44 nT) models were higher. However, this does not reflect an increase in the power spectra of the Detrending-2 and Detrending-3 models. This is because the amplitude of the residuals also increased with the order of the polynomials. The maximum values of the residuals for the Detrending-2 and Detrening-3 models were 8.43 and 8.14 nT, respectively, which exceeded even their respective maximum values of 7.57 and 7.44 nT. Therefore, the observed increase in value for Detrending-2 and Detrending-3 model may be attributed to detrending processing itself, which altered the data value rather than indicating an actual enhancement of the signal strength.
The detrending models were compared with the CSES original model in terms of the distribution of the residuals and the power spectrum. Figure 5 shows a grid map of the comparison of their residuals. Three profiles of anomalies in the east–west residuals were identified in the four detrending models along latitudes 0°, 30°, and 60°. As the order of the polynomials increased, so did the magnitude of the residuals, indicating a greater change in the dataset. The 0- and 1-order polynomials were both straight lines, and the corresponding changes in the dataset were relatively limited. However, the 2- and 3-order polynomials were characterized by curves, and the corresponding changes in the dataset were large, particularly in the high-latitude region that was more severely influenced by external fields. Moreover, there were two prominent distorted signals nearly along 40° N and 40° S. They were obtained because we conducted the data quality control at low latitudes (|latitude| ≤ 40°) and high latitudes (40° < |latitude| < 65°) separately during modeling. This led to the elimination of data containing severe interference at high latitudes, and the resulting edge effect led to worse fitting at 40° latitude.
Figure 6a shows a comparison of power spectra between the CSES original model and the four detrending models at SH degree 1–42. In the range of SH degree 1–15, the inverted coefficients were not zero and were more sensitive to detrending-related processing than those at larger degrees. We removed the core field to SH degree15, and set the coefficients below degree 16 to zero to avoid uncertainty in the results of fitting of low-degree terms. The power of the detrending models was lower than that of the CSES original model, and continued to decrease as the order of the polynomials increased. Figure 6b shows the differences in the power spectrum obtained by subtracting those of the detrending models from that of the CSES original model. It more clearly shows the reduction in power. This reveals that the strength of the signal decreased when interference by the residual trend was suppressed during the detrending of the data. The reduction in signal strength was more significant for detrending models that used higher-order polynomials.
We estimated the SH coefficients of the CSES dataset by using the LSQR algorithm over 60 iterations. Figures 7a–e illustrate the Lowes–Mauersberger power spectra (Mauersberger, 1956; Lowes, 1966) of the CSES original model and the four detrending models under different times of iterations.
The power spectrum of the CSES models showed that their results were consistent under different times of iterations until SH degree 42. Above degree 42, the differences in the power spectra of the models under different times of iterations increased prominently such that their results became unreliable. We thus truncated the CSES original model at degree 42 (red dashed line) and used the results obtained after 40 iterations.
After detrending the data, the convergence of the power spectrum improved significantly under different times of iterations, and the results remained highly consistent until degree 44. To quantitatively measure the consistency of the results under different times of iterations, Figures 7f–j show the SH correlation analysis between the model run for 40 iterations and those iterated for different times of iterations. The SH correlation between the CSES original model when run for 20 and 60 iterations was 0.93 at degree 42, while it was greater than 0.96 after detrending the data. The convergence of the model did not further improve with an increase in the order of the polynomials.
To further evaluate the detrending models, we compared their regional details with those of the CSES original model and the CHAOS-7 model. Figure 8 shows the magnetic anomalies in the Himalayan–Tibetan Plateau and surrounding regions, obtained at an altitude of 500 km, as calculated by the CSES original model, CHAOS-7 model, and the four detrending models. The southern Himalayan–Tibetan Plateau features an east–west-trending magnetic low anomaly (HMLA), and there is another significant high magnetic anomaly in the Tarim Basin (TMA) to the north. The distributions of these magnetic anomalies obtained by all models were similar, but there were prominent differences in their details. We provide some examples below.
HMLA: The HMLA appeared as three separate low magnetic anomalies lined up in an east–west direction in the CSES original model. However, the HMLA tended to be connected as a single anomaly in the CHAOS-7 model and the detrending models, indicating that the discontinuity in the CSES original model might have been related to the residual trends, and that the detrending had improved the consistency of data from different orbits. But from Detrending-1 model, the spatial area of HMLA decreases.
TMA: The shape of the TMA changed significantly between the Detrending-2 and -3 models, and the latter contained serious distortions. The center of the TMA in it had a prominent northward offset, indicating that detrending had introduced a large deviation in the magnetic anomaly.
In this study, we used polynomial fitting to remove the residual trend of data on magnetic anomalies obtained from the CSES satellite, and used polynomials of orders 0 to 3 to derive four detrending models. The aim was to identify the influence of the residual trends on modeling. We compared the detrending models with the CSES original model in terms of the power spectrum and grid maps. The following conclusions can be drawn:
(1) Positive aspect of detrending: After detrending, the misfit between the model and the dataset decreased, and the convergence of the SH coefficients improved.
(2) Negative aspect of detrending: Detrending led to a reduction in signal strength and a decline in the power spectrum. Detrending based on polynomials of orders 2 and 3 introduced prominent distortions in the details of the anomalies.
(3) Suggestion on detrending: We recommend detrending the data on magnetic anomalies obtained by the CSES before modeling, but by using only 0-order polynomials, i.e., subtracting a constant value to reduce the mean value of the magnetic anomaly to zero. This will lead to only a slight loss of signal strength in return for a significant improvement in the stability of the coefficient and details of the anomalies. Further analysis of the sources of residual signals in different satellites and more advanced methods of detrending are needed to provide a comprehensive strategy for dealing with residual trends.
This work made use of the data from CSES mission (https://leos.ac.cn), a project funded by the China National Space Administration (CNSA) and the Ministry of Emergency Management of China. Thanks for the coefficient and code sharing of CHAOS-7 model (http://www.spacecenter.dk/files/magnetic-models/CHAOS-7 for model coefficients). This work was supported by the Civil Aerospace Technology Pilot Research Project (D040203), the National Natural Science Foundation of China (
Arkani-Hamed, J., Langel, R. A., and Purucker, M. (1994). Scalar magnetic anomaly maps of Earth derived from POGO and Magsat data. J. Geophys. Res.: Solid Earth, 99(B12), 24075–24090. https://doi.org/10.1029/94JB00930
|
Cheng, B. J., Zhou, B., Magnes, W., Lammegger, R., and Pollinger, A. (2018). High precision magnetometer for geomagnetic exploration onboard of the China Seismo-Electromagnetic Satellite. Sci. China Technol. Sci., 61(5), 659–668. https://doi.org/10.1007/s11431-018-9247-6
|
Ferré, E. C., Friedman, S. A., Martín-Hernández, F., Feinberg, J. M., Till, J. L., Ionov, D. A., and Conder, J. A. (2014). Eight good reasons why the uppermost mantle could be magnetic. Tectonophysics, 624–625, 3–14. https://doi.org/10.1016/j.tecto.2014.01.004
|
Finlay, C. C., Lesur, V., Thébault, E., Vervelidou, F., Morschhauser, A., and Shore, R. (2017). Challenges handling magnetospheric and ionospheric signals in internal geomagnetic field modelling. Space Sci. Rev., 206(1), 157–189. https://doi.org/10.1007/s11214-016-0285-9
|
Finlay, C. C., Kloss, C., Olsen, N., Hammer, M. D., Tøffner-Clausen, L., Grayver, A., and Kuvshinov, A. (2020). The CHAOS-7 geomagnetic field model and observed changes in the South Atlantic Anomaly. Earth Planets Space, 72(1), 156. https://doi.org/10.1186/s40623-020-01252-9
|
Kauristie, K., Morschhauser, A., Olsen, N., Finlay, C. C., McPherron, R. L., Gjerloev, J. W., and Opgenoorth H J. (2017). On the usage of geomagnetic indices for data selection in internal field modelling. Space Sci. Rev., 206(1), 61–90. https://doi.org/10.1007/s11214-016-0301-0
|
Langel, R. A., and Hinze, W. J. (1998). The Magnetic Field of the Earth’s Lithosphere: The Satellite Perspective. Oxford: Cambridge University Press. https://doi.org/10.1017/CBO9780511629549
|
Lesur, V., Rother, M., Vervelidou, F., Hamoudi, M., and Thébault, E. (2013). Post-processing scheme for modelling the lithospheric magnetic field. Solid Earth, 4(1), 105–118. https://doi.org/10.5194/se-4-105-2013
|
Lowes, F. J. (1966). Mean-square values on sphere of spherical harmonic vector fields. J. Geophys. Res., 71(8), 2179. https://doi.org/10.1029/JZ071i008p02179
|
Mauersberger, P. (1956). Das mittel der energiedichte des geomagnetischen hauptfeldes an der Erdoberfläche und seine saulare anderung. Gerl. Beitr. Geophys., 65, 207–215.
|
Maus, S., Rother, M., Holme, R., Lühr, H., Olsen, N., and Haak, V. (2002). First scalar magnetic anomaly map from CHAMP satellite data indicates weak lithospheric field. Geophys. Res. Lett., 29(14), 45–1–47–4. https://doi.org/10.1029/2001GL013685
|
Maus, S., Lühr, H., Rother, M., Hemant, K., Balasis, G., Ritter, P., and Stolle, C. (2007). Fifth-generation lithospheric magnetic field model from CHAMP satellite measurements. Geochem. Geophys. Geosyst., 8(5), Q05013. https://doi.org/10.1029/2006GC001521
|
Olsen, N., Ravat, D., Finlay, C. C., and Kother, L. K. (2017). LCS-1: A high-resolution global model of the lithospheric magnetic field derived from CHAMP and Swarm satellite observations. Geophys. J. Int., 211(3), 1461–1477. https://doi.org/10.1093/gji/ggx381
|
Paige, C. C., and Saunders, M. A. (1982). LSQR: An algorithm for sparse linear equations and sparse least squares. ACM Trans. Math. Softw., 8(1), 43–71. https://doi.org/10.1145/355984.355989
|
Pollinger, A., Lammegger, R., Magnes, W., Hagen, C., Ellmeier, M., Jernej, I., Leichtfried, M., Kürbisch, C., Maierhofer, R., … Wallner, R. (2018). Coupled dark state magnetometer for the China Seismo-Electromagnetic Satellite. Meas. Sci. Technol., 29(9), 095103. https://doi.org/10.1088/1361-6501/aacde4
|
Pollinger, A., Amtmann, C., Betzler, A., Cheng, B. J., Ellmeier, M., Hagen, C., Jernej, I., Lammegger, R., Zhou, B., and Magnes, W. (2020). In-orbit results of the coupled dark state magnetometer aboard the China Seismo-Electromagnetic Satellite. Geosci. Instrum. Method. Data Syst., 9(2), 275–291. https://doi.org/10.5194/gi-9-275-2020
|
Purucker, M., Langlais, B., Olsen, N., Hulot, G., and Mandea, M. (2002). The southern edge of cratonic North America: Evidence from new satellite magnetometer observations. Geophys. Res. Lett., 29(15), 56–1–56–4. https://doi.org/10.1029/2001GL013645
|
Purucker, M. E., and Clark, D. A. (2011). Mapping and interpretation of the lithospheric magnetic field. In M. Mandea, et al. (Eds.), Geomagnetic Observations and Models (pp. 311–337). Dordrecht: Springer. https://doi.org/10.1007/978-90-481-9858-0_13
|
Regan, R. D., Cain, J. C., and Davis, W. M. (1975). A global magnetic anomaly map. J. Geophys. Res., 80(5), 794–802. https://doi.org/10.1029/JB080i005p00794
|
Sabaka, T. J., Olsen, N., Tyler, R. H., and Kuvshinov, A. (2015). CM5, a pre-swarm comprehensive geomagnetic field model derived from over 12 yr of CHAMP, Ørsted, SAC-C and observatory data. Geophys. J. Int., 200(3), 1596–1626. https://doi.org/10.1093/gji/ggu493
|
Shen, X. H., Zhang, X. M., Yuan, S. G., Wang, L. W., Cao, J. B., Huang, J. P., Zhu, X. H., Piergiorgio, P., and Dai, J. P. (2018). The state-of-the-art of the China Seismo-Electromagnetic Satellite mission. Sci. China Technol. Sci., 61(5), 634–642. https://doi.org/10.1007/s11431-018-9242-0
|
Thébault, E., Purucker, M., Whaler, K. A., Langlais, B., and Sabaka, T. J. (2010). The magnetic field of the Earth’s lithosphere. Space Sci. Rev., 155(1), 95–127. https://doi.org/10.1007/s11214-010-9667-6
|
Thébault, E., Vervelidou, F., Lesur, V., and Hamoudi, M. (2012). The satellite along-track analysis in planetary magnetism. Geophys. J. Int., 188(3), 891–907. https://doi.org/10.1111/j.1365-246X.2011.05281.x
|
Thébault, E., Lesur, V., Kauristie, K., and Shore, R. (2017). Magnetic field data correction in space for modelling the lithospheric magnetic field. Space Sci. Rev., 206(1), 191–223. https://doi.org/10.1007/s11214-016-0309-5
|
Wang, J., Shen, X. H., Yang, Y. Y., Zeren, Z., Hulot, G., Olsen, N., Zhou, B., Magnes, W., De Santis, A., … Yu, J. B. (2021). Initial scalar lithospheric magnetic anomaly map of China and surrounding regions derived from CSES satellite data. Sci. China Technol. Sci., 64(5), 1118–1126. https://doi.org/10.1007/s11431-020-1727-0
|
Wang, J., Shen, X. H., Yang, Y. Y., Zeren, Z. M., Zhou, B., Werner, M., De Santis, A., Huang, J. P., Yao, C. L., … Lammegger, R. (2023a). A global lithospheric magnetic field model between ± 65° latitude derived from CSES satellite scalar data. Phys. Earth Planet. Inter., 340, 107036. https://doi.org/10.1016/j.pepi.2023.107036
|
Wang, J., Shen, X. H., Yang, Y. Y., Zeren, Z. M., Huang, J. P., Zhao, S. F., Wang, Q., Lu, H. X., and Guo, F. (2023b). The spherical cap harmonic model of lithospheric magnetic anomaly in Chinese region derived from CSES satellite data. Chinese J. Geophys. (in Chinese), 66(7), 2973–2982. https://doi.org/10.6038/cjg2022Q0857
|
Wang, J., Yang, Y. Y., Ji, F., Luo, Y. X., Zeren, Z. M., Huang, J. P., and Shen, X. H. (2024a). Lithospheric long-wavelength magnetic anomalies and their geological origins: A review. Rev. Geophys. Planet. Phys. (in Chinese), 55(6), 668–680. https://doi.org/10.19975/j.dqyxx.2023-056
|
Wang, J., Yang, Y. Y., Zeren, Z. M., Wang, J., Wang, X., Luo, Y. X., and Shen, X. H. (2024b). Preliminary geological interpretation of long-wavelength magnetic anomalies over China and surrounding regions. Earth Planet. Phys., 8(3), 445–458. https://doi.org/10.26464/epp2024025
|
Yang, Y. Y., Zhou, B., Hulot, G., Olsen, N., Wu, Y. Y., Xiong, C., Stolle, C., Zeren, Z. M., Huang, J. P., … Shen, X. H. (2021a). CSES high precision magnetometer data products and example study of an intense geomagnetic storm. J. Geophys. Res.: Space Phys., 126(4), e2020JA028026. https://doi.org/10.1029/2020JA028026
|
Yang, Y. Y., Hulot, G., Vigneron, P., Shen, X. H., Zeren, Z. M., Zhou, B., Magnes, W., Olsen, N., Tøffner-Clausen, L., … Zhu, X. H. (2021b). The CSES Global Geomagnetic Field Model (CGGM): An IGRF-type global geomagnetic field model based on data from the China Seismo-Electromagnetic Satellite. Earth Planets Space, 73, 45. https://doi.org/10.1186/s40623-020-01316-w
|
Zeren, Z. M., Zhou, B., Zhao, S. F., Wang, Q., Huang, J. P., Zeng, L., Lei, J. G., Chen, Y., Li, C., … Shen, X. H. (2022). Cross-calibration on the electromagnetic field detection payloads of the China Seismo-Electromagnetic Satellite. Sci. China Technol. Sci., 65(6), 1415–1426. https://doi.org/10.1007/s11431-021-1965-2
|
Zeren, Z. M., Yang, Y. Y., Yan, R., Zhang, Z. X., Wang, J., He, H., Xu, S., Lu, H. X., Zhou, N., and Huang, J. P. (2024). The representative scientific results of the China Seismo-Electromagnetic Satellite. Earthq. Res. Adv., 100314. https://doi.org/10.1016/j.eqrea.2024.100314
|
Zhou, B., Cheng, B. J., Gou, X. C., Li, L., Zhang, Y. T., Wang, J. D., Magnes, W., Lammegger, R., Pollinger, A., … Shen, X. H. (2019). First in-orbit results of the vector magnetic field measurement of the High Precision Magnetometer onboard the China Seismo-Electromagnetic Satellite. Earth Planets Space, 71, 119. https://doi.org/10.1186/s40623-019-1098-3
|
S. Williams, D. Gubbins, P. W. Livermore, Y. Jiang. 2025: Evaluation of the lithospheric magnetic field mapped by the first year of MSS-1 data. Earth and Planetary Physics, 9(3): 1-8. DOI: 10.26464/epp2025033 | |
JianPing Huang, ZongYu Li, Zhong Li, XingHong Zhu, JunGang Lei, YuanQing Miao, WenJing Li. 2025: Evaluation of the satellite’s wake effect on the electric field detector onboard the CSES-01 satellite. Earth and Planetary Physics, 9(2): 400-409. DOI: 10.26464/epp2024077 | |
Jie Wang, YanYan Yang, ZhiMa Zeren, Jian Wang, Xin Wang, YuXin Luo, XuHui Shen. 2024: Preliminary geological interpretation of long-wavelength magnetic anomalies over China and surrounding regions. Earth and Planetary Physics, 8(3): 445-458. DOI: 10.26464/epp2024025 | |
Yi Jiang, Nils Olsen, JiaMing Ou, Qing Yan. 2023: Simulation for MSS-2 low-perigee elliptical orbit satellites: an example of lithospheric magnetic field modelling. Earth and Planetary Physics, 7(1): 151-160. DOI: 10.26464/epp2023021 | |
PengFei Liu, Yi Jiang, Qing Yan, Ann M. Hirt. 2023: The behavior of a lithospheric magnetization and magnetic field model. Earth and Planetary Physics, 7(1): 66-73. DOI: 10.26464/epp2023025 | |
YuanZheng Wen, Dan Tao, GuangXue Wang, JiaYi Zong, JinBin Cao, Roberto Battiston, ZhiMa ZeRen, XuHui Shen. 2022: Ionospheric TEC and plasma anomalies possibly associated with the 14 July 2019 Mw7.2 Indonesia Laiwui earthquake, from analysis of GPS and CSES data. Earth and Planetary Physics, 6(4): 313-328. DOI: 10.26464/epp2022028 | |
YaLu Wang, XueMin Zhang, XuHui Shen. 2018: A study on the energetic electron precipitation observed by CSES. Earth and Planetary Physics, 2(6): 538-547. DOI: 10.26464/epp2018052 | |
XueMin Zhang, Vladimir Frolov, ShuFan Zhao, Chen Zhou, YaLu Wang, Alexander Ryabov, DuLin Zhai. 2018: The first joint experimental results between SURA and CSES. Earth and Planetary Physics, 2(6): 527-537. DOI: 10.26464/epp2018051 | |
Rui Yan, XuHui Shen, JianPing Huang, Qiao Wang, Wei Chu, DaPeng Liu, YanYan Yang, HengXin Lu, Song Xu. 2018: Examples of unusual ionospheric observations by the CSES prior to earthquakes. Earth and Planetary Physics, 2(6): 515-526. DOI: 10.26464/epp2018050 | |
Bin Zhou, YanYan Yang, YiTeng Zhang, XiaoChen Gou, BingJun Cheng, JinDong Wang, Lei Li. 2018: Magnetic field data processing methods of the China Seismo-Electromagnetic Satellite. Earth and Planetary Physics, 2(6): 455-461. DOI: 10.26464/epp2018043 |
Satellite | Mean (nT) | Satellite | Mean (nT) |
Ørsted | 0.53 | Swarm-A | - 0.07 |
CHAMP | - 0.53 | Swarm-B | - 0.07 |
SAC-C | 0.10 | Swarm-C | 0.00 |
CryoSat-2 | 0.02 |
Model | Misfit (nT) | Field value (nT) | Residual with CHAOS-7 (nT) | Residual with CSES original (nT) |
CSES original | 0.0019 | (−8.52, 8.39) | (−2.90, 5.75) | − |
Detrending-0 | 0.0013 | (−8.83, 7.74) | (−3.29, 5.31) | (−1.01, 1.13) |
Detrending-1 | 0.0010 | (−8.91, 7.27) | (−3.04, 5.81) | (−2.12, 1.96) |
Detrending-2 | 0.0007 | (−7.89, 7.57) | (−3.95, 8.43) | (−3.03, 3.15) |
Detrending-3 | 0.0006 | (−7.91, 7.44) | (−4.34, 8.14) | (−3.48, 3.99) |