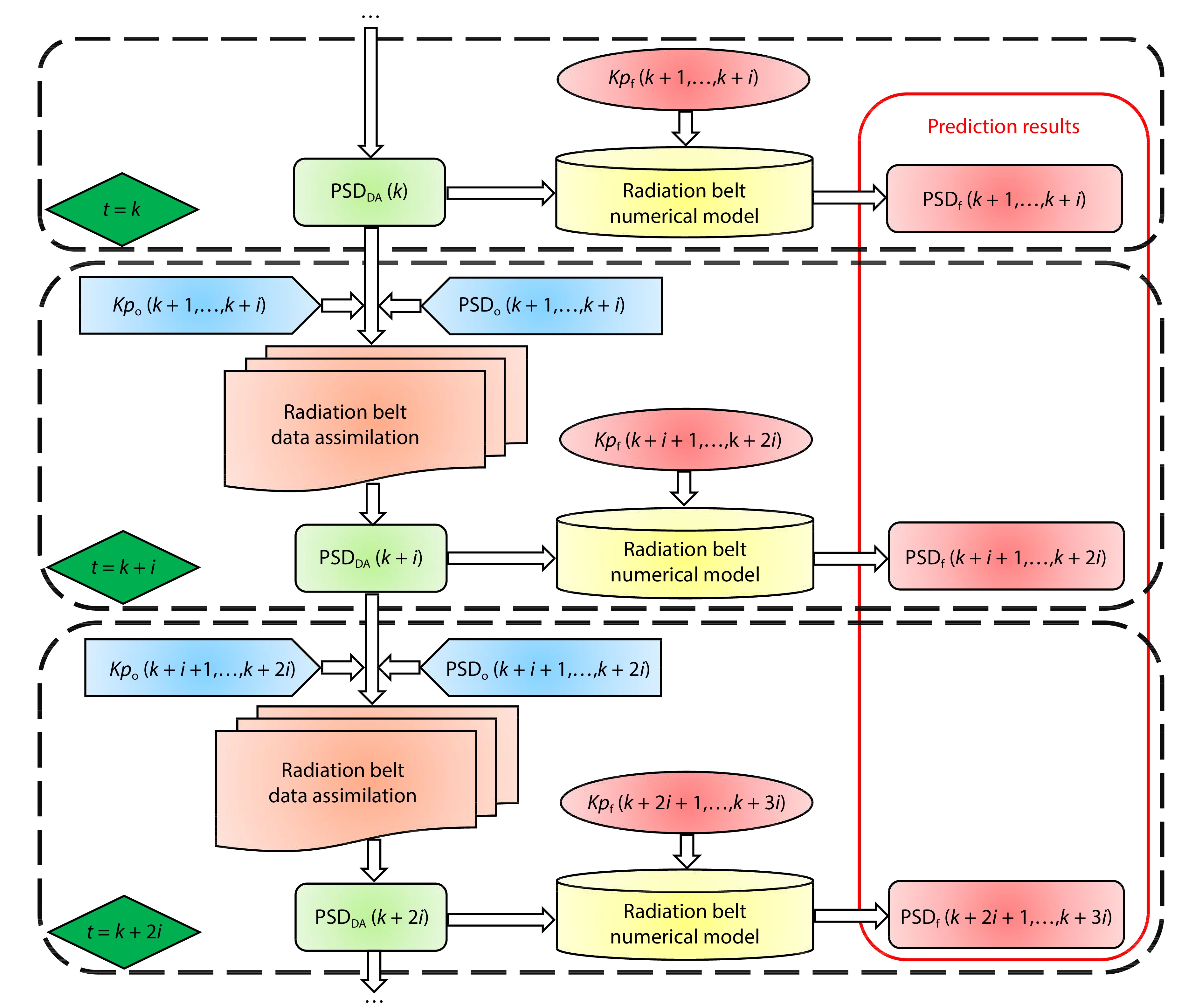
Citation: | Lei, Y., Cao, X., Ni, B. B., Fu, S., Luo, T. R., and Wang X. Y. (2023). A data assimilation-based forecast model of outer radiation belt electron fluxes. Earth Planet. Phys., 7(6), 620–630. DOI: 10.26464/epp2023079 |
Because radiation belt electrons can pose a potential threat to the safety of satellites orbiting in space, it is of great importance to develop a reliable model that can predict the highly dynamic variations in outer radiation belt electron fluxes. In the present study, we develop a forecast model of radiation belt electron fluxes based on the data assimilation method, in terms of Van Allen Probe measurements combined with three-dimensional radiation belt numerical simulations. Our forecast model can cover the entire outer radiation belt with a high temporal resolution (1 hour) and a spatial resolution of 0.25 L over a wide range of both electron energy (0.1–5.0 MeV) and pitch angle (5°–90°). On the basis of this model, we forecast hourly electron fluxes for the next 1, 2, and 3 days during an intense geomagnetic storm and evaluate the corresponding prediction performance. Our model can reasonably predict the storm-time evolution of radiation belt electrons with high prediction efficiency (up to ~0.8–1). The best prediction performance is found for ~0.3–3 MeV electrons at L = ~3.25–4.5, which extends to higher L and lower energies with increasing pitch angle. Our results demonstrate that the forecast model developed can be a powerful tool to predict the spatiotemporal changes in outer radiation belt electron fluxes, and the model has both scientific significance and practical implications.
The Earth’s outer radiation belt consists of high-energy electrons (~100 keV–10 MeV) trapped by the geomagnetic field and is located at L-shell = ~3–7. A majority of medium- and high-orbit satellites pass through the region covered by the outer belt, where the electrons can undermine spacecraft electronics via deep-dielectric charging and discharging phenomena. The outer belt electron fluxes are usually characterized by complex and intense dynamics as a result of the imbalance between acceleration, loss, and transport processes (Ni BB et al., 2013a, 2015, 2022; Baker et al., 2014; Fu S et al., 2020; Mei Y et al., 2021; Wang CQ et al., 2021). Resonant interactions with plasma waves are recognized as playing a crucial role in the dynamic evolution of radiation belt electrons (Thorne, 2010). A series of studies have demonstrated that many plasma waves, such as electromagnetic ion cyclotron (EMIC) waves, magnetosonic waves, whistler-mode chorus waves, and plasmaspheric hiss, can effectively affect the radiation belt electron dynamics via pitch angle and energy diffusion (Summers et al., 2003; Thorne et al., 2007, 2013; Hudson et al., 2008; Fu HS et al., 2011; Reeves et al., 2013; Xiao FL et al., 2014, 2015; Ni BB et al., 2016, 2017, 2019, 2023a, b; Li LY et al., 2016; Cao X et al., 2017a, b, 2020a, 2023; Zong QG et al., 2017; Fu S et al., 2019; Zhang DJ et al., 2020; Capannolo et al., 2021; Zhu, MH et al., 2022; He, F et al., 2023).
Satellite measurements provide an effective means for understanding the radiation belt electron dynamics. However, they are usually limited to a restricted coverage of L-shell, electron energy, and pitch angle. By solving the three-dimensional (3-D) Fokker–Planck diffusion equation, various numerical models have been developed to simulate the dynamics of radiation belt electrons, including the Salammbô code (Beutier and Boscher, 1995), Versatile Electron Radiation Belt (VERB)-3D code (Shprits et al., 2009), Storm-Time Evolution of Electron Radiation Belt (STEERB) code (Su ZP et al., 2010), Dynamic Radiation Environment Assimilation Model (DREAM)-3D code (Tu WC et al., 2013), British Antarctic Survey (BAS) Radiation Belt code (Glauert et al., 2014), and University of California, Los Angeles (UCLA)-3D code (Ma Q et al., 2015). However, radiation belt numerical models still possess some unavoidable shortcomings, such as simplification treatment in solving equations and inaccurate initial and boundary conditions.
Data assimilation (DA) techniques are able to combine numerical models and observation data, taking into account the inherent errors of both separately, to optimally estimate the true state of the system. Therefore, data assimilation has been widely used in the radiation belt community to reconstruct the spatial and temporal distribution of radiation belt electrons. The available data assimilation algorithms that are used in radiation belt research consist of the Kalman filter (KF; e.g., Shprits et al., 2007, 2012, 2013; Ni BB et al., 2009a, b, 2013b; Daae et al., 2011; Schiller et al., 2012; Kellerman et al., 2014; Zhu JN et al., 2021), extended Kalman filter (EKF; e.g., Naehr et al., 2005; Kondrashov et al., 2007, 2011), and ensemble Kalman filter (EnKF; e.g., Koller et al., 2007; Godinez and Koller, 2012; Reeves et al., 2012; Yu Y et al., 2014; Tibocha et al., 2021).
To ensure the safety of spacecraft, various models have been developed in previous studies to forecast the evolution of energetic electrons at Geostationary Earth Orbit (GEO). Through linear prediction filter analysis, Baker et al. (1990) predicted radiation belt electron fluxes using the geomagnetic or solar wind indices as inputs. This method was subsequently implemented to develop the relativistic electron forecast model operating at the Space Weather Prediction Center (SWPC). Kellerman et al. (2013) developed a prediction model of radiation belt electron fluxes based on the measurements of solar wind parameters to forecast relativistic electron fluxes at GEO. In addition, the nonlinear processes of electron flux variations have been considered. Boynton et al. (2016) developed an empirical model based on the Nonlinear Autoregressive Moving Average with Exogenous inputs (NARMAX) algorithm to predict geosynchronous electron fluxes. Recently, the neural network technique has been extensively utilized in predicting electron fluxes in the radiation belts (Ling AG et al., 2010; Shin et al., 2016; Wei LH et al., 2018; Zhang H et al., 2020; Son et al., 2022; Tang RX et al., 2022; Wing et al., 2022; Wang JH et al., 2023).
Prediction models also exist that can predict variations in the outer radiation belt electron fluxes over a wide spatial range. Rigler et al. (2004) used the KF to recursively update the linear prediction filter coefficients for the prediction of relativistic electron fluxes. Coleman et al. (2018) developed a nowcast model of 2.1 MeV electron fluxes at L = 3–6 by using a time-varying linear filter. Chen Y et al. (2019) developed the PREdictive models for MeV Electrons (PreMevE) by using a linear prediction filter, which can predict megaelectron volt electron fluxes at L = 2.8–7 with low-orbit satellite data as inputs. Combining machine learning algorithms, de Lima et al. (2020) subsequently upgraded PreMevE to provide improved predictions of megaelectron-volt electron fluxes. However, the aforementioned models focus only on the prediction of electron fluxes at a specific energy or the integral fluxes.
In this work, we reconstruct the distribution of electron fluxes in the outer radiation belt, using the KF method by combining Van Allen Probe observations and 3-D radiation belt numerical modeling. We further develop a forecast model of electron fluxes covering a wide range of electron energy (0.1–5 MeV) and L* (3–7). We have organized this manuscript as follows: Van Allen Probe instruments and the data set used are briefly described in Section 2. Section 3 briefly introduces our algorithms and models. The forecasted results and performance evaluations of this model are presented in Section 4. Finally, the main conclusions of this study are summarized in Section 5.
In the present study, we adopt high-quality electron data observed by the identical twin Van Allen Probes during the period from April to May 2017. Their orbits are characterized by a low inclination (~10°), a perigee of ~1.1 RE (Earth’s radius) and an apogee of ~5.9 RE, with a period of ~9 h (Mauk et al., 2013). The electron data used in this study are measured by two in situ instrumentations: the Magnetic Electron Ion Spectrometer (MagEIS) instrumentation and the Relativistic Electron Proton Telescope (REPT) instrumentation. The MagEIS instrumentation covers an energy range of ~30 keV–4 MeV in 21 energy channels, and the REPT instrumentation covers an energy range of ~1.8–20 MeV in 12 energy channels. The corresponding pitch angles detected by MagEIS and REPT are ~8° to 172° and ~5° to 175°, respectively.
The spatial and temporal evolution of radiation belt electron phase space density (PSD) can be obtained by solving the 3-D Fokker–Planck equation in the coordinates of three adiabatic invariants (μ, K, L*; Schulz and Lanzerotti, 1974):
$$ \begin{split} \frac{\partial {f}}{\partial {t}}=&{{L}}^{{*}2}{\left.\frac{\partial }{\partial {{L}}^{{*}}}\right|}_{{\mu },{K}}\left(\frac{1}{{{L}}^{{*}2}}{{D}}_{{{L}}^{{*}}{{L}}^{{*}}}{\left.\frac{\partial {f}}{\partial {{L}}^{{*}}}\right|}_{{\mu },{K}}\right)+\frac{1}{{{p}}^{2}}{\left.\frac{\partial {f}}{\partial {p}}\right|}_{{{\alpha }}_{0},{{L}}^{{*}}}{{p}}^{2}\left({{D}}_{{p}{p}}{\left.\frac{\partial {f}}{\partial {p}}\right|}_{{{\alpha }}_{0},{{L}}^{{*}}}\right)\\ &+\frac{1}{{T}\left({{\alpha }}_{0}\right){\rm{sin}}\left(2{{\alpha }}_{0}\right)}{\left.\frac{\partial }{\partial {{\alpha }}_{0}}\right|}_{{p},{{L}}^{{*}}}T\left({{\alpha }}_{0}\right){\rm{sin}}\left(2{{\alpha }}_{0}\right)\left({{D}}_{{{\alpha }}_{0}{{\alpha }}_{0}}{\left.\frac{\partial {f}}{\partial {{\alpha }}_{0}}\right|}_{{p},{{L}}^{{*}}}\right)-\frac{{f}}{{\tau }}\;,\end{split} $$ | (1) |
where
In this study, we obtain
The KF, a sequential data assimilation method, allows us to consider the error level of different measurements and a physics-based model and optimally combine the measurements and the model. In this study, the KF is applied to obtain an optimal estimate of radiation belt electron fluxes.
Assuming the dynamic process of the electron PSD evolution described by the 3-D Fokker–Planck equation can be represented by the numerically discretized form:
$$ {\boldsymbol{x}}_{{k}}^{{f}}={\boldsymbol{M}}_{{k}-1}{\boldsymbol{x}}_{{k}-1}^{{a}} , $$ | (2) |
where
$$ {\boldsymbol{x}}_{{k}}^{{t}}={\boldsymbol{M}}_{{k}-1}{\boldsymbol{x}}_{{k}-1}^{{t}}+{\eta}_{{k}-1}^{{m}} , $$ | (3) |
where superscript
$$ {\boldsymbol{P}}_{{k}}^{{f}}={\boldsymbol{M}}_{{k}-1}{\boldsymbol{P}}_{{k}-1}^{{a}}{\boldsymbol{M}}_{{k}-1}^{\rm{T}}+{\boldsymbol{Q}}_{{k}-1} , $$ | (4) |
where
$$ {\boldsymbol{y}}_{{k}}^{{o}}={\boldsymbol{H}}_{{k}}{\boldsymbol{x}}_{{k}}^{{t}}+{\text{ε}}_{{k}}^{{o}} , $$ | (5) |
where superscript
The vector of observations and the vector of the forecast state are used to obtain the analysis state vector
$$ {\boldsymbol{x}}_{{k}}^{{a}}={\boldsymbol{x}}_{{k}}^{{f}}+{\boldsymbol{K}}_{{k}}\left({\boldsymbol{y}}_{{k}}^{{o}}-{\boldsymbol{H}}_{{k}}{\boldsymbol{x}}_{{k}}^{{f}}\right) , $$ | (6) |
where the Kalman gain
$$ {\boldsymbol{K}}_{{k}}={\boldsymbol{P}}_{{k}}^{{f}}{\boldsymbol{H}}_{{k}}^{\rm{T}}{\left({\boldsymbol{H}}_{{k}}{\boldsymbol{P}}_{{k}}^{{f}}{\boldsymbol{H}}_{{k}}^{\rm{T}}+{\boldsymbol{R}}_{{k}}\right)}^{-1} , $$ | (7) |
$$ {\boldsymbol{P}}_{{k}}^{{a}}=\left(\boldsymbol{I}-{\boldsymbol{K}}_{{k}}{\boldsymbol{H}}_{{k}}\right){\boldsymbol{P}}_{{k}}^{{f}} . $$ | (8) |
In this study, we predict electron fluxes for the next i hours, and we assume that electron flux and Kp observations will be received every ith hour during this period. This process can be divided into (1) the forecast process and (2) the assimilation process. The main steps in forecasting electron fluxes are as follows:
(1) Forecast process. The assimilative results of electron PSD at t = k is used as the initial value for forecast. Then, the forecasted value of Kp for each hour in the future, i hours, is input into the numerical model to obtain the forecasted value of PSD for each hour in the future i hours, respectively.
(2) Assimilation process. After i hours, namely t = k + i, the PSD observations and Kp observations for the past i hours are received. Then, the PSD assimilative value at t = k is used as the initial value for assimilation to obtain the PSD assimilative value at the current moment, which is then used as the initial value for the forecast of the next forecast period.
As time advances, the process of forecasting and assimilation is continuously cycled, and the forecast of electron fluxes is realized for the whole time period. Note that the forecasted value of Kp is replaced by its real value, the results of measurement in this study. Our forecast model is able to predict the radiation belt electron fluxes hourly with a spatial resolution of 0.25 L* at L* = 3–7 in the pitch angle range of 5°–90° and the energy range of 0.1–5 MeV.
Usually, a period of runs is required to achieve the optimal model performance when reconstructing electron fluxes using the data assimilation method. Therefore, we first reconstruct the electron fluxes in April 2017 to stabilize the assimilation model, and then predict the electron fluxes in May 2017 for the next 1, 2, and 3 days, respectively.
Figure 2 shows the observed and forecasted electron fluxes for different electron energies and forecast times at a fixed αeq = 30°. Figure 2a illustrates the Kp and Dst indices. Figures 2b–2e and 2f–2i show the results for Ek = 0.5 and 1 MeV, respectively. The observed electron fluxes are shown in Figures 2b and 2f, and the assimilative electron fluxes in April 2017 and the forecasted electron fluxes in May 2017 for the next 1, 2, and 3 days are shown in Figures 2c–2e and 2g–2i. The data assimilation results not only fill the gaps in the observations, but also accurately reconstruct the radial profiles and the evolution of the electron fluxes. The assimilative results are used as initial conditions for model prediction. It can be seen that the 1-day forecast for Ek = 0.5 MeV in Figure 2c can well predict the process of radiation belt electrons in May 2017, including the prompt enhancement of electron fluxes after the intense geomagnetic storm occurred on May 28, although the electron dropout at high L* during the main phase is not captured well. The forecasted results for the electron fluxes at Ek = 0.5 MeV for the 2- and 3-day forecasts generally agree well with the observations, but the discrepancies become pronounced with the extension of forecast time. Compared with 0.5 MeV electrons, the forecasted results for 1 MeV electrons are closer to the observations. Note that as the prediction time increases, the model prediction performance for 1 MeV electrons is more stable than that for 0.5 MeV electrons.
In Figure 3, we further show the observed and forecasted electron fluxes for Ek = 2 and 5 MeV electrons at αeq = 60°. The figure shows that the assimilative results generally reproduce the distribution of 2 and 5 MeV electrons at L* = 3–7 in April 2017. On the basis of the assimilative results in April, our model subsequently predicts the evolution of electron fluxes for Ek = 2 and 5 MeV electrons. We can see that the prediction for 2 MeV electrons is closer to the observations than is the prediction for 5 MeV electrons. Especially during the strong geomagnetic storm on May 28, the predicted fluxes of 5 MeV electrons are much higher than the observations. This result may be attributed to the absence of the EMIC wave-induced loss of ultrarelativistic electrons (Ni BB et al., 2015; Cao X et al., 2017c, 2020b).
Figure 4 illustrates the comparison between the observed and forecasted electron fluxes for different electron energies and pitch angles in May 2017 at L* = 3.5, 4.5, and 5.5. Figures 4a–4f show the results for 0.5 and 1 MeV electrons at αeq = 30°, and Figure 4g–4l show the results for 2 and 5 MeV electrons at αeq = 60°. The green circles in each panel indicate the observations. The black, blue, and red lines represent forecasted electron fluxes for 1-, 2-, and 3-day forecasts, respectively. We can see that compared with the 1 and 2 MeV electrons, the discrepancies between the observed and forecasted fluxes of 0.5 and 5 MeV electrons are more pronounced. The forecasted results at L* = 4.5 are found to be closer to the observations than are those at L* = 3.5 and 5.5, except for 0.5 MeV electrons at αeq = 30°. In addition, 0.5 and 5 MeV electron fluxes are found to be seriously overestimated after the geomagnetic storm.
To quantitatively evaluate our model performance, the mean error (ME), root mean squared error (RMSE), and prediction efficiency (PE) are calculated, which are given by
$$ {\rm{ME}}=\frac{1}{{N}}{\sum }_{{i}=1}^{{N}}\left({{f}}_{{i}}-{{o}}_{{i}}\right) , $$ | (9) |
$$ {\rm{RMSE}}=\sqrt{\frac{1}{{N}}{\sum }_{{i}=1}^{{N}}{\left({{f}}_{{i}}-{{o}}_{{i}}\right)}^{2}} , $$ | (10) |
$$ {\rm{PE}}=1-\frac{{\displaystyle\sum }_{{i}=1}^{{N}}{\left({{f}}_{{i}}-{{o}}_{{i}}\right)}^{2}}{{\displaystyle\sum }_{{i}=1}^{{N}}{\left({{o}}_{{i}}-\langle{o}\rangle\right)}^{2}}={{1}}-\frac{{\rm{RMSE}}^{2}}{{\rm{Var}}\left({o}\right)} . $$ | (11) |
where
To estimate the performance of our model for predicting electron fluxes, Figure 5 shows the results of ME, RMSE, and PE as a function of electron energy and L* for 1-day forecast corresponding to αeq = 30°, 60°, and 80°. Figures 5a–5c show that <0.3 MeV electron fluxes are underestimated at L* < 4 and overestimated at L* > 4.5. For >3 MeV electrons, their fluxes are overestimated at L* > 4.5. This result is consistent with Figures 5d–5f, which show that the RMSE in these areas are more obvious than others. We suggest that the discrepancies at low L* may be caused by the inaccurate empirical model of plasmaspheric hiss and the inaccurate position of plasmapause in our model. The overestimations at high L* may have originated from the absence of electron loss resulting from EMIC wave scattering and magnetopause shadowing affects (Xiang Z et al., 2017, 2018). Figure 5d clearly illustrates that the RMSE of the model for < ~0.7 MeV electron fluxes at ~L* = 5–5.25 is more pronounced than at other L*. We suggest that this may be attributed to the fact that the electron dropout is poorly predicted by our model during this intense geomagnetic storm event on May 28. The RMSE indicates the absolute difference between predicated and observed values, yet this is often an inaccurate measure of the prediction performance of the model for different datasets because of the large differences in magnitude between the electron fluxes at different energies and L*. It is thus more appropriate to use PE to compare our model performance for the electron flux prediction at different energies and L*. Figures 5g–i show high PE (~0.7–1) for 0.3–3 MeV electrons at L* = ~3.25–4.5, which gradually extends to higher L* and lower energies with increasing pitch angle. Note that PE is significantly decreased for ~2.5 MeV electrons with increasing electron energies. Lower PE for higher energy electrons also may be a result of the missing scattering effects of EMIC waves on electrons in our forecast model. The dependence of our model performance on different prediction times is further shown in Figure 6. The figure shows that as the prediction time increases, the model performance gradually declines, regardless of electron energy and L*.
Son et al. (2022) developed a deep learning model to predict the fluxes of >2 MeV electrons at GEO and PE for 1, 2, and 3 days ahead predictions were 0.78, 0.64, and 0.53, respectively. Although PE at GEO in our model is not available because of the absence of satellite measurements, Figure 5 shows that our model performance for ~0.3–3 MeV electrons at L* = ~3.25–4.5 is comparable or even better than that of Son et al. (2022). We also compare it with the prediction performance of the PreMevE model (Chen Y et al., 2019). We find that the PE of our forecast model for 1 MeV electrons is lower than the PreMevE (with the peak PE higher than 0.8 at L = ~3.5) at αeq = 30° but higher than the PreMevE at αeq ≥ 60°.
In this study, a forecast model of outer radiation belt electron fluxes is developed based on a data assimilation technique through the combination of Van Allen Probes measurements and 3-D radiation belt numerical simulations. Our model is able to predict electron fluxes with high temporal (1 hour) and spatial (0.25 L) resolutions over a wide electron energy range (0.1–5 MeV) and pitch angle range (5°–90°). We forecast radiation belt electron fluxes for the next 1, 2, and 3 days based on this model and evaluate the corresponding prediction performance.
The main conclusions can be summarized as follows:
(1) The data assimilation method can optimally estimate the current state of electron fluxes as the initial values for model prediction. Further, our model can reasonably forecast hourly electron fluxes for the next 1, 2, and 3 days, with high PE above ~0.8.
(2) Compared with previous studies, our model not only has good prediction performance, but also can predict radiation belt electron fluxes (L* = 3–7) in the outer belt over a wider pitch angle (5°–90°) and energy range (0.1–5 MeV).
(3) Our model has the best prediction performance (up to ~0.8–1) for electron fluxes at Ek = ~0.3–3 MeV and L* = ~3.25–4.5, which gradually extends to higher L* and lower energies with increasing pitch angle. In addition, the prediction performance becomes more robust as the prediction time decreases.
In this study, we consider only the resonant scattering of radiation belt electrons by whistler-mode chorus waves and plasmaspheric hiss. It is of future interest to include the contributions of other plasma waves (e.g., magnetosonic waves, EMIC waves) to the acceleration and loss processes of radiation belt electrons in our forecast model. Specifically, because EMIC waves can effectively cause the pitch angle scattering loss of relativistic electrons, the absence of EMIC waves in our model will result in the overestimation of relativistic electron fluxes. In addition, magnetopause shadowing effects, which are a crucial mechanism responsible for the loss of electrons, should be taken into account. For simplicity, the observed Kp is used for forecasting radiation belt electron fluxes. It is thus of future interest to better predict radiation belt fluxes by combining a Kp prediction model. Long-term forecasting is also required to perform a more comprehensive and accurate analysis of our model performance, which will be addressed in future work.
This work was supported by the National Natural Science Foundation of China (Grant Nos. 42025404, 42188101, and 42241143), the National Key R&D Program of China (Grant Nos. 2022YFF0503700 and 2022YFF0503900), the B-type Strategic Priority Program of the Chinese Academy of Sciences (Grant No. XDB41000000), and the Fundamental Research Funds for the Central Universities (Grant No. 2042022kf1012). We are grateful to the VAP-ECT group for providing Van Allen Probe electron flux data. All Van Allen Probe data are available from the website (http://www.RBSP-ect.lanl.gov/). We also thank the OMNIWeb service (https://omniweb.gsfc.nasa.gov/) for providing the geomagnetic indices.
Baker, D. N., McPherron, R. L., Cayton, T. E., and Klebesadel, R. W. (1990). Linear prediction filter analysis of relativistic electron properties at 6.6 RE. J. Geophys. Res.:Space Phys., 95(A9), 15133–15140. https://doi.org/10.1029/ja095ia09p15133
|
Baker, D. N., Jaynes, A. N., Hoxie, V. C., Thorne, R. M., Foster, J. C., Li, X., Fennell, J. F., Wygant, J. R., Kanekal, S. G., .. Lanzerotti, L. J. (2014). An impenetrable barrier to ultrarelativistic electrons in the Van Allen radiation belts. Nature, 515(7528), 531–534. https://doi.org/10.1038/nature13956
|
Beutier, T., and Boscher, D. (1995). A three-dimensional analysis of the electron radiation belt by the Salammbô code. J. Geophys. Res.:Space Phys., 100(A8), 14853–14861. https://doi.org/10.1029/94JA03066
|
Boynton, R. J., Balikhin, M. A., Sibeck, D. G., Walker, S. N., Billings, S. A., and Ganushkina, N. (2016). Electron flux models for different energies at geostationary orbit. Space Weather, 14(10), 846–860. https://doi.org/10.1002/2016SW001506
|
Brautigam, D. H., and Albert, J. M. (2000). Radial diffusion analysis of outer radiation belt electrons during the October 9, 1990, magnetic storm. J. Geophys. Res.:Space Phys., 105(A1), 291–309. https://doi.org/10.1029/1999JA900344
|
Cao, X., Ni, B. B., Liang, J., Xiang, Z., Wang, Q., Shi, R., Gu, X. D., Zhou, C., Zhao, Z. Y., .. Liu, J. (2016). Resonant scattering of central plasma sheet protons by multiband EMIC waves and resultant proton loss timescales. J. Geophys. Res.:Space Phys., 121(2), 1219–1232. https://doi.org/10.1002/2015JA021933
|
Cao, X., Ni, B. B., Summers, D., Zou, Z. Y., Fu, S., and Zhang, W. X. (2017a). Bounce resonance scattering of radiation belt electrons by low-frequency hiss: Comparison with cyclotron and Landau resonances. Geophys. Res. Lett., 44(19), 9547–9554. https://doi.org/10.1002/2017GL075104
|
Cao, X., Ni, B. B., Summers, D., Bortnik, J., Tao, X., Shprits, Y. Y., Lou, Y. Q., Gu, X. D., Fu, S., .. Wang, Q. (2017b). Bounce resonance scattering of radiation belt electrons by H+ band EMIC waves. J. Geophys. Res.:Space Phys., 122(2), 1702–1713. https://doi.org/10.1002/2016JA023607
|
Cao, X., Shprits, Y. Y., Ni, B. B., and Zhelavskaya, I. S. (2017c). Scattering of ultra-relativistic electrons in the Van Allen radiation belts accounting for hot plasma effects. Sci. Rep., 7(1), 17719. https://doi.org/10.1038/s41598-017-17739-7
|
Cao, X., Ni, B. B., Summers, D., Fu, S., Gu, X. D., and Shi, R. (2020a). Hot plasma effects on the pitch-angle scattering rates of radiation belt electrons due to plasmaspheric hiss. Astrophys. J., 896(2), 118. https://doi.org/10.3847/1538-4357/ab9107
|
Cao, X., Ni, B. B., Summers, D., Shprits, Y. Y., and Lou, Y. Q. (2020b). Effects of polarization reversal on the pitch angle scattering of radiation belt electrons and ring current protons by EMIC waves. Geophys. Res. Lett., 47(17), e2020GL089718. https://doi.org/10.1029/2020GL089718
|
Cao, X., Lu, P., Zhu, Q., Ma, X., and Ni, B. B. (2023). Bounce resonance scattering of slot region electrons by plasmaspheric hiss. Chin. J. Geophys. (in Chinese)
|
Capannolo, L., Li, W., Spence, H., Johnson, A. T., Shumko, M., Sample, J., and Klumpar, D. (2021). Energetic electron precipitation observed by FIREBIRD-II potentially driven by EMIC waves: Location, extent, and energy range from a multievent analysis. Geophys. Res. Lett., 48(5), e2020GL091564. https://doi.org/10.1029/2020GL091564
|
Carpenter, D. L., and Anderson, R. R. (1992). An ISEE/whistler model of equatorial electron density in the magnetosphere. J. Geophys. Res.:Space Phys., 97(A2), 1097–1108. https://doi.org/10.1029/91JA01548
|
Chen, Y., Reeves, G. D., Fu, X. R., and Henderson, M. (2019). PreMevE: New predictive model for megaelectron-volt electrons inside Earth’s outer radiation belt. Space Weather, 17(3), 438–454. https://doi.org/10.1029/2018SW002095
|
Coleman, T., McCollough, J. P., Young, S., and Rigler, E. J. (2018). Operational nowcasting of electron flux levels in the outer zone of Earth’s radiation belt. Space Weather, 16(5), 501–518. https://doi.org/10.1029/2017SW001788
|
Daae, M., Shprits, Y. Y., Ni, B., Koller, J., Kondrashov, D., and Chen, Y. (2011). Reanalysis of radiation belt electron phase space density using various boundary conditions and loss models. Adv. Space Res., 48(8), 1327–1334. https://doi.org/10.1016/j.asr.2011.07.001
|
de Lima, R. P., Chen, Y., and Lin, Y. Z. (2020). Forecasting megaelectron-volt electrons inside Earth’s outer radiation belt: PreMevE 2.0 based on supervised machine learning algorithms. Space Weather, 18(2), e2019SW002399. https://doi.org/10.1029/2019SW002399
|
Fu, H. S., Cao, J. B., Yang, B., and Lu, H. Y. (2011). Electron loss and acceleration during storm time: The contribution of wave–particle interaction, radial diffusion, and transport processes. J. Geophys. Res.:Space Phys., 116(A10), A10210. https://doi.org/10.1029/2011JA016672
|
Fu, S., Ni, B. B., Zhou, R. X., Cao, X., and Gu, X. D. (2019). Combined scattering of radiation belt electrons caused by Landau and bounce resonant interactions with magnetosonic waves. Geophys. Res. Lett., 46(17-18), 10313–10321. https://doi.org/10.1029/2019GL084438
|
Fu, S., Yi, J., Ni, B. B., Zhou, R. X., Hu, Z. J., Cao, X., Gu, X. D., and Guo, D. Y. (2020). Combined scattering of radiation belt electrons by low-frequency hiss: Cyclotron, Landau, and bounce resonances. Geophys. Res. Lett., 47(5), e2020GL086963. https://doi.org/10.1029/2020GL086963
|
Glauert, S. A., Horne, R. B., and Meredith, N. P. (2014). Three-dimensional electron radiation belt simulations using the BAS Radiation Belt Model with new diffusion models for chorus, plasmaspheric hiss, and lightning-generated whistlers. J. Geophys. Res.:Space Phys., 119(1), 268–289. https://doi.org/10.1002/2013JA019281
|
Godinez, H. C., and Koller, J. (2012). Localized adaptive inflation in ensemble data assimilation for a radiation belt model. Space Weather, 10(8), S08001. https://doi.org/10.1029/2012SW000767
|
He, F., Yao, Z. H., Ni, B. B., Cao, X., Ye, S. Y., Guo, R. L., Li, J. X., Ren, Z. P., Yue, X. A., Zhang, Y. L., Wei, Y., Zhang, X. X., and Pu, Z. Y. (2023). Sawtooth and dune auroras simultaneously driven by waves around the plasmapause. Earth Planet. Phys., 7(2), 237–246. https://doi.org/10.26464/epp2023023
|
Hudson, M. K., Kress, B. T., Mueller, H. R., Zastrow, J. A., and Blake, J. B. (2008). Relationship of the Van Allen radiation belts to solar wind drivers. J. Atmos. Solar-Terr. Phys., 70(5), 708–729. https://doi.org/10.1016/j.jastp.2007.11.003
|
Kellerman, A. C., Shprits, Y. Y., and Turner, D. L. (2013). A Geosynchronous Radiation-belt Electron Empirical Prediction (GREEP) model. Space Weather, 11(8), 463–475. https://doi.org/10.1002/swe.20074
|
Kellerman, A. C., Shprits, Y. Y., Kondrashov, D., Subbotin, D., Makarevich, R. A., Donovan, E., and Nagai, T. (2014). Three-dimensional data assimilation and reanalysis of radiation belt electrons: Observations of a four-zone structure using five spacecraft and the VERB code. J. Geophys. Res.:Space Phys., 119(11), 8764–8783. https://doi.org/10.1002/2014JA020171
|
Koller, J., Chen, Y., Reeves, G. D., Friedel, R. H. W., Cayton, T. E., and Vrugt, J. A. (2007). Identifying the radiation belt source region by data assimilation. J. Geophys. Res.:Space Phys., 112(A6), A06244. https://doi.org/10.1029/2006JA012196
|
Kondrashov, D., Shprits, Y., Ghil, M., and Thorne, R. (2007). A Kalman filter technique to estimate relativistic electron lifetimes in the outer radiation belt. J. Geophys. Res.:Space Phys., 112(A10), A10227. https://doi.org/10.1029/2007JA012583
|
Kondrashov, D., Ghil, M., and Shprits, Y. (2011). Lognormal Kalman filter for assimilating phase space density data in the radiation belts. Space Weather, 9(11), S11006. https://doi.org/10.1029/2011SW000726
|
Li, L. Y., Yu, J., Cao, J. B., Wang, Z. Q., Yu, Y. Q., Reeves, G. D., and Li, X. (2016). Effects of ULF waves on local and global energetic particles: Particle energy and species dependences. J. Geophys. Res.:Space Phys., 121(11), 11007–11020. https://doi.org/10.1002/2016JA023149
|
Li, W., Shprits, Y. Y., and Thorne, R. M. (2007). Dynamic evolution of energetic outer zone electrons due to wave–particle interactions during storms. J. Geophys. Res.:Space Phys., 112(A10), A10220. https://doi.org/10.1029/2007JA012368
|
Ling, A. G., Ginet, G. P., Hilmer, R. V., and Perry, K. L. (2010). A neural network–based geosynchronous relativistic electron flux forecasting model. Space Weather, 8(9), S09003. https://doi.org/10.1029/2010SW000576
|
Ma, Q., Li, W., Thorne, R. M., Ni, B., Kletzing, C. A., Kurth, W. S., Hospodarsky, G. B., Reeves, G. D., Henderson, M. G., Spence, H. E., .. Angelopoulos, V. (2015). Modeling inward diffusion and slow decay of energetic electrons in the Earth’s outer radiation belt. Geophys. Res. Lett., 42(4), 987–995. https://doi.org/10.1002/2014GL062977
|
Mauk, B. H., Fox, N. J., Kanekal, S. G., Kessel, R. L., Sibeck, D. G., and Ukhorskiy, A. (2013). Science objectives and rationale for the radiation belt storm probes mission. Space Sci. Rev., 179(1), 3–27. https://doi.org/10.1007/s11214-012-9908-y
|
Mei, Y., Ge, Y. S., Du, A. M., Gu, X. D., Summers, D., Li, X. L., Fu, S., and Xiang, Z. (2021). Energy-dependent boundaries of Earth’s radiation belt electron slot region. Astrophys. J., 922(2), 246. https://doi.org/10.3847/1538-4357/ac25ec
|
Naehr, S. M., and Toffoletto, F. R. (2005). Radiation belt data assimilation with an extended Kalman filter. Space Weather, 3(6), S06001. https://doi.org/10.1029/2004SW000121
|
Ni, B. B., Thorne, R. M., Shprits, Y. Y., and Bortnik, J. (2008). Resonant scattering of plasma sheet electrons by whistler-mode chorus: Contribution to diffuse auroral precipitation. Geophys. Res. Lett., 35(11), L11106. https://doi.org/10.1029/2008GLO34032
|
Ni, B. B., Shprits, Y., Thorne, R., Friedel, R., and Nagai, T. (2009a). Reanalysis of relativistic radiation belt electron phase space density using multisatellite observations: Sensitivity to empirical magnetic field models. J. Geophys. Res.:Space Phys., 114(A12), A12208. https://doi.org/10.1029/2009JA014438
|
Ni, B. B., Shprits, Y., Nagai, T., Thorne, R., Chen, Y., Kondrashov, D., and Kim, H. J. (2009b). Reanalyses of the radiation belt electron phase space density using nearly equatorial CRRES and polar-orbiting Akebono satellite observations. J. Geophys. Res.:Space Phys., 114(A5), A05208. https://doi.org/10.1029/2008JA013933
|
Ni, B. B., Bortnik, J., Thorne, R. M., Ma, Q. L., and Chen, L. J. (2013a). Resonant scattering and resultant pitch angle evolution of relativistic electrons by plasmaspheric hiss. J. Geophys. Res.:Space Phys., 118(12), 7740–7751. https://doi.org/10.1002/2013JA019260
|
Ni, B. B., Shprits, Y. Y., Friedel, R. H. W., Thorne, R. M., Daae, M., and Chen, Y. (2013b). Responses of Earth’s radiation belts to solar wind dynamic pressure variations in 2002 analyzed using multisatellite data and Kalman filtering. J. Geophys. Res.:Space Phys., 118(7), 4400–4414. https://doi.org/10.1002/jgra.50437
|
Ni, B. B., Cao, X., Zou, Z. Y., Zhou, C., Gu, X. D., Bortnik, J., Zhang, J. C., Fu, S., Zhao, Z. Y., .. Xie, L. (2015). Resonant scattering of outer zone relativistic electrons by multiband EMIC waves and resultant electron loss time scales. J. Geophys. Res.:Space Phys., 120(9), 7357–7373. https://doi.org/10.1002/2015JA021466
|
Ni, B. B., Thorne, R. M., Zhang, X. J., Bortnik, J., Pu, Z. Y., Xie, L., Hu, Z. J., Han, D. S., Shi, R., Zhou, C., and Gu, X. D. (2016). Origins of the Earth’s diffuse auroral precipitation. Space Sci. Rev., 200(1), 205–259. https://doi.org/10.1007/s11214-016-0234-7
|
Ni, B. B., Hua, M., Zhou, R. X., Yi, J., and Fu, S. (2017). Competition between outer zone electron scattering by plasmaspheric hiss and magnetosonic waves. Geophys. Res. Lett., 44(8), 3465–3474. https://doi.org/10.1002/2017GL072989
|
Ni, B. B., Huang, H., Zhang, W. X., Gu, X. D., Zhao, H., Li, X. L., Baker, D., Fu, S., Xiang, Z., and Cao, X. (2019). Parametric sensitivity of the formation of reversed electron energy spectrum caused by plasmaspheric hiss. Geophys. Res. Lett., 46(8), 4134–4143. https://doi.org/10.1029/2019GL082032
|
Ni, B. B., Hua, M., Gu, X. D., Fu, S., Xiang, Z., Cao, X., and Ma, X. (2022). Artificial modification of Earth’s radiation belts by ground-based very-low-frequency (VLF) transmitters. Sci. China Earth Sci., 65(3), 391–413. https://doi.org/10.1007/s11430-021-9850-7
|
Ni, B. B., Zhang, Y., and Gu, X. D. (2023a). Identification of ring current proton precipitation driven by scattering of electromagnetic ion cyclotron waves. Fundam. Res., 3(2), 257–264. https://doi.org/10.1016/j.fmre.2021.12.018
|
Ni, B. B., Summers, D., Xiang, Z., Dou, X. K., Tsurutani, B. T., Meredith, N. P., Dong, J. H., Chen, L. J., Reeves, G. D., .. Xu, W. (2023b). Unique banded structures of plasmaspheric hiss waves in the Earth’s magnetosphere. J. Geophys. Res.:Space Phys., 128(3), e2023JA031325. https://doi.org/10.1029/2023JA031325
|
Reeves, G. D., Chen, Y., Cunningham, G. S., Friedel, R. W. H., Henderson, M. G., Jordanova, V. K., Koller, J., Morley, S. K., Morley, S. K., Thomsen, M. F., and Zaharia, S. (2012). Dynamic radiation environment assimilation model: DREAM. Space Weather, 10(3), S03006. https://doi.org/10.1029/2011SW000729
|
Reeves, G. D., Spence, H. E., Henderson, M. G., Morley, S. K., Friedel, R. H. W., Funsten, H. O., Baker, D. N., Kanekal, S. G., Blake, J. B., .. Niehof, J. T. (2013). Electron acceleration in the heart of the Van Allen radiation belts. Science, 341(6149), 991–994. https://doi.org/10.1126/science.1237743
|
Rigler, E. J., Baker, D. N., Weigel, R. S., Vassiliadis, D., and Klimas, A. J. (2004). Adaptive linear prediction of radiation belt electrons using the Kalman filter. Space Weather, 2(3), S03003. https://doi.org/10.1029/2003SW000036
|
Schiller, Q., Li, X., Koller, J., Godinez, H., and Turner, D. L. (2012). A parametric study of the source rate for outer radiation belt electrons using a Kalman filter. J. Geophys. Res.:Space Phys., 117(A9), A09211. https://doi.org/10.1029/2012JA017779
|
Schulz, M., and Lanzerotti, L. J. (1974). Particle Diffusion in the Radiation Belts. Berlin: Springer. https://doi.org/10.1007/978-3-642-65675-0
|
Shin, D. K., Lee, D. Y., Kim, K. C., Hwang, J., and Kim, J. (2016). Artificial neural network prediction model for geosynchronous electron fluxes: Dependence on satellite position and particle energy. Space Weather, 14(4), 313–321. https://doi.org/10.1002/2015SW001359
|
Shprits, Y., Kondrashov, D., Chen, Y., Thorne, R., Ghil, M., Friedel, R., and Reeves, G. (2007). Reanalysis of relativistic radiation belt electron fluxes using CRRES satellite data, a radial diffusion model, and a Kalman filter. J. Geophys. Res.:Space Phys., 112(A12), A12216. https://doi.org/10.1029/2007JA012579
|
Shprits, Y., Daae, M., and Ni, B. B. (2012). Statistical analysis of phase space density buildups and dropouts. J. Geophys. Res.:Space Phys., 117(A1), A01219. https://doi.org/10.1029/2011JA016939
|
Shprits, Y., Kellerman, A., Kondrashov, D., and Subbotin, D. (2013). Application of a new data operator-splitting data assimilation technique to the 3-D VERB diffusion code and CRRES measurements. Geophys. Res. Lett., 40(19), 4998–5002. https://doi.org/10.1002/grl.50969
|
Shprits, Y. Y., Subbotin, D., and Ni, B. B. (2009). Evolution of electron fluxes in the outer radiation belt computed with the VERB code. J. Geophys. Res.:Space Phys., 114(A11), A11209. https://doi.org/10.1029/2008JA013784
|
Son, J., Moon, Y. J., and Shin, S. (2022). 72-hour time series forecasting of hourly relativistic electron fluxes at geostationary orbit by deep learning. Space Weather, 20(10), e2022SW003153. https://doi.org/10.1029/2022SW003153
|
Su, Z. P., Xiao, F. L., Zheng, H. N., and Wang, S. (2010). STEERB: A three-dimensional code for storm-time evolution of electron radiation belt. J. Geophys. Res.:Space Phys., 115(A9), A09208. https://doi.org/10.1029/2009JA015210
|
Summers, D., and Thorne, R. M. (2003). Relativistic electron pitch-angle scattering by electromagnetic ion cyclotron waves during geomagnetic storms. J. Geophys. Res.:Space Phys., 108(A4), 1143. https://doi.org/10.1029/2002JA009489
|
Tang, R. X., Tao, Y. H., Li, J. H., Chen, Z., Deng, X. H., and Li, H. M. (2022). The short-time prediction of the energetic electron flux in the planetary radiation belt based on stacking ensemble-learning algorithm. Space Weather, 20(2), e2021SW002969. https://doi.org/10.1029/2021SW002969
|
Thorne, R. M., Shprits, Y. Y., Meredith, N. P., Horne, R. B., Li, W., and Lyons, L. R. (2007). Refilling of the slot region between the inner and outer electron radiation belts during geomagnetic storms. J. Geophys. Res.:Space Phys., 112(A6), A06203. https://doi.org/10.1029/2006JA012176
|
Thorne, R. M. (2010). Radiation belt dynamics: The importance of wave–particle interactions. Geophys. Res. Lett., 37(22), L22107. https://doi.org/10.1029/2010GL044990
|
Thorne, R. M., Li, W., Ni, B., Ma, Q., Bortnik, J., Chen, L., Baker, D. N., Spence, H. E., Reeves, G. D., .. Kanekal, S. G. (2013). Rapid local acceleration of relativistic radiation-belt electrons by magnetospheric chorus. Nature, 504(7480), 411–414. https://doi.org/10.1038/nature12889
|
Tibocha, A. M. C., de Wiljes, J., Shprits, Y. Y., and Aseev, N. A. (2021). Reconstructing the dynamics of the outer electron radiation belt by means of the standard and ensemble Kalman filter with the verb-3D code. Space Weather, 19(10), e2020SW002672. https://doi.org/10.1029/2020SW002672
|
Tu, W. C., Cunningham, G. S., Chen, Y., Henderson, M. G., Camporeale, E., and Reeves, G. D. (2013). Modeling radiation belt electron dynamics during GEM challenge intervals with the DREAM3D diffusion model. J. Geophys. Res.:Space Phys., 118(10), 6197–6211. https://doi.org/10.1002/jgra.50560
|
Wang, C. Q., Zhang, X. G., Shen, G. H., Zhang, S. Y., Zhang, X. X., Huang, C., and Li, X. J. (2021). Dynamic results of electron flux in radiation belt from 2011 to 2015 based on FY-3B satellite observation. Chin. J. Geophys. (in Chinese)
|
Wang, J. H., Guo, D. Y., Xiang, Z., Ni, B. B., Liu, Y. X. Z., and Dong, J. H. (2023). Prediction of geosynchronous electron fluxes using an artificial neural network driven by solar wind parameters. Adv. Space Res., 71(1), 275–285. https://doi.org/10.1016/j.asr.2022.10.013
|
Wei, L. H., Zhong, Q. Z., Lin, R. L., Wang, J. J., Liu, S. Q., and Cao, Y. (2018). Quantitative prediction of high-energy electron integral flux at geostationary orbit based on deep learning. Space Weather, 16(7), 903–916. https://doi.org/10.1029/2018SW001829
|
Wing, S., Turner, D. L., Ukhorskiy, A. Y., Johnson, J. R., Sotirelis, T., Nikoukar, R., and Romeo, G. (2022). Modeling radiation belt electrons with information theory informed neural networks. Space Weather, 20(8), e2022SW003090. https://doi.org/10.1029/2022SW003090
|
Xiang, Z., Tu, W. C., Li, X. L., Ni, B. B., Morley, S. K., and Baker, D. N. (2017). Understanding the mechanisms of radiation belt dropouts observed by Van Allen Probes. J. Geophys. Res.:Space Phys., 122(10), 9858–9879. https://doi.org/10.1002/2017JA024487
|
Xiang, Z., Tu, W. C., Ni, B. B., Henderson, M. G., and Cao, X. (2018). A statistical survey of radiation belt dropouts observed by Van Allen Probes. Geophys. Res. Lett., 45(16), 8035–8043. https://doi.org/10.1029/2018GL078907
|
Xiao, F. L., Yang, C., He, Z. G., Su, Z. P., Zhou, Q. H., He, Y. H., Kletzing, C. A., Kurth, W. S., Hospodarsky, G. B., .. Wygant, J. R. (2014). Chorus acceleration of radiation belt relativistic electrons during March 2013 geomagnetic storm. J. Geophys. Res.:Space Phys., 119(5), 3325–3332. https://doi.org/10.1002/2014JA019822
|
Xiao, F. L., Zhou, Q. H., He, Y. H., Yang, C., Liu, S., Baker, D. N., Spence, H. E., Reeves, G. D., Funsten, H. O., and Blake, J. B. (2015). Penetration of magnetosonic waves into the plasmasphere observed by the Van Allen Probes. Geophys. Res. Lett., 42(18), 7287–7294. https://doi.org/10.1002/2015GL065745
|
Yu, Y., Koller, J., Jordanova, V. K., Zaharia, S. G., and Godinez, H. C. (2014). Radiation belt data assimilation of a moderate storm event using a magnetic field configuration from the physics-based RAM-SCB model. Ann Geophys., 32(5), 473–483. https://doi.org/10.5194/angeo-32-473-2014
|
Zhang, D. J., Liu, W. L., Li, X. L., Sarris, T. E., Wang, Y. F., Xiao, C., Zhang, Z., and Wygant, J. R. (2020). Relation between shock-related impulse and subsequent ULF wave in the Earth’s magnetosphere. Geophys. Res. Lett., 47(23), e2020GL090027. https://doi.org/10.1029/2020GL090027
|
Zhang, H., Fu, S. Y., Xie, L., Zhao, D., Yue, C., Pu, Z. Y., Xiong, Y., Wu, T., Zhao, S. J., .. Luo, Z. K. (2020). Relativistic electron flux prediction at geosynchronous orbit based on the neural network and the quantile regression method. Space Weather, 18(9), e2020SW002445. https://doi.org/10.1029/2020SW002445
|
Zhu, J. N., Guo, J. G., Ni, B. B., Cao, X., Xiang, Z., Fu, S., Gu, X. D., and Ma, X. (2021). Multi-dimensional data assimilation and analyses of Earth’s outer electron radiation belt. Chin. J. Geophys. (in Chinese)
|
Zhu, M. H., Yu, Y. Q., Cao, X., Ni, B. B., Tian, X. B., Cao, J. B., and Jordanova, V. K. (2022). Effects of polarization-reversed electromagnetic ion cyclotron waves on the ring current dynamics. Earth Planet. Phys., 6(4), 329–338. https://doi.org/10.26464/epp2022037
|
Zong, Q. G., Rankin, R., and Zhou, X. Z. (2017). The interaction of ultra-low-frequency Pc3-5 waves with charged particles in Earth’s magnetosphere. Rev. Mod. Plasma Phys., 1, 10. https://doi.org/10.1007/s41614-017-0011-4
|
1. | Zhang, Q., Ma, X., Guo, D. et al. Multi-dimensional modeling of the diffusionrates of radiation belt electrons by whistle-mode chorus waves and simulations of resultant radiation belt electron loss timescales | [哨声模合声波对辐射带电子扩散系数的多维建模及损失时间尺度建模研究]. Acta Geophysica Sinica, 2024, 67(5): 1679-1698. DOI:10.6038/cjg2023R0548 | |
2. | Luo, T., Cao, X., Ma, X. et al. Three-dimensional data assimilative model of the Earth's outer radiation belt electrons based on the Fortran language | [基于Fortran语言的地球外辐射带电子三维数据 同化建模]. Acta Geophysica Sinica, 2024, 67(4): 1285-1298. DOI:10.6038/cjg2023R0259 |