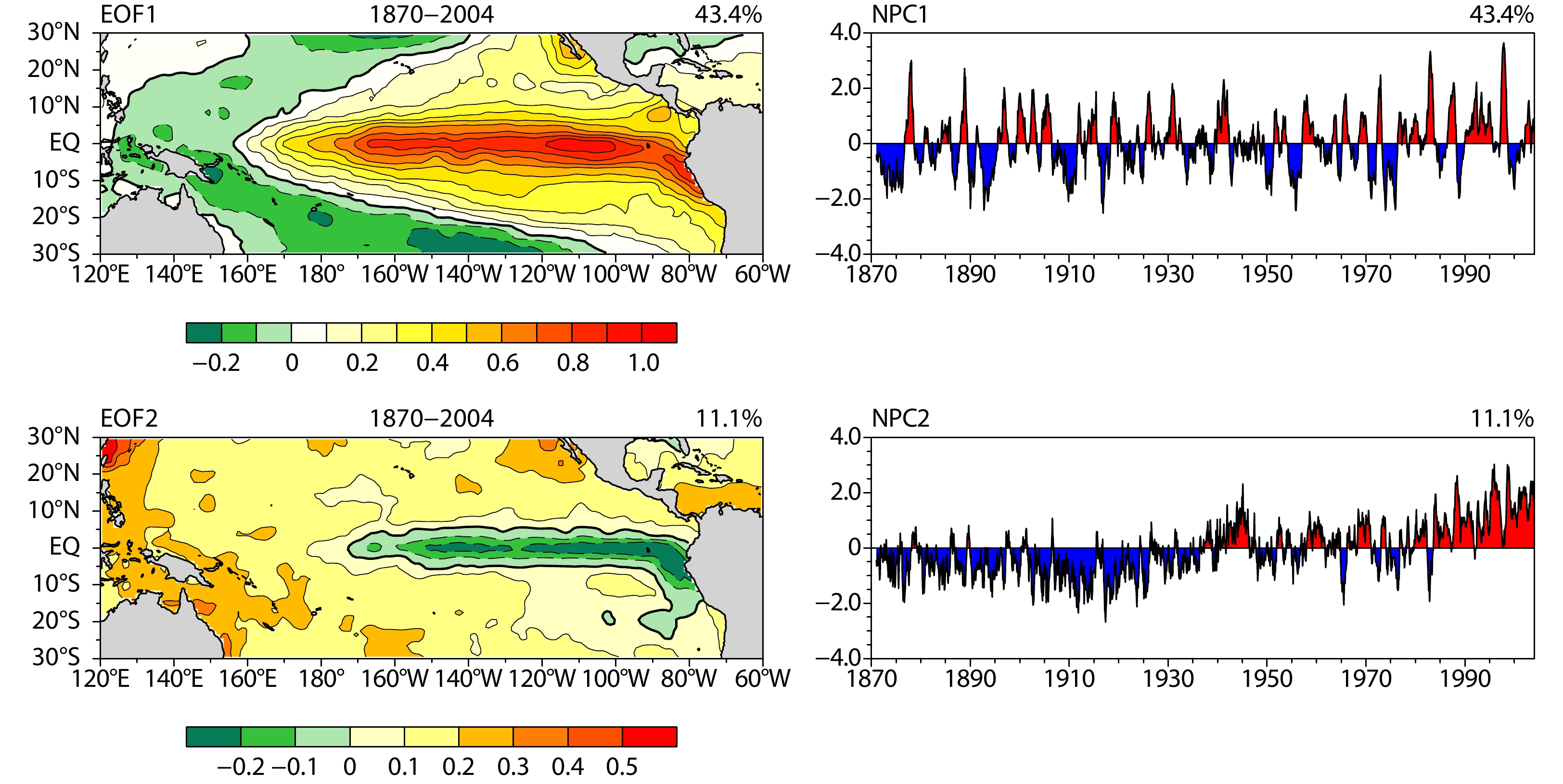
Citation: | Li, Y., Chen, Q. L., Li, J. P., Zhang, W. J., Song, M. H., Hua, W., Cai, H. K., and Wu, X. F. (2019). The tropical Pacific cold tongue mode and its associated main ocean dynamical process in CMIP5 models. Earth Planet. Phys., 3(5), 400–413.. DOI: 10.26464/epp2019041 |
The cold tongue mode (CTM), which represents the out-of-phase relationship in sea surface temperature anomaly (SSTA) variability between the Pacific cold tongue region and elsewhere in the tropical Pacific, shows a long-term cooling trend in the eastern equatorial Pacific. In this study, we investigate how well the CTM is reproduced in historical simulations generated by the 20 models considered in Phase 5 of the Coupled Model Intercomparison Project (CMIP5). Qualitatively, all 20 models roughly capture the cooling SSTA associated with the CTM. However, a quantitative assessment (i.e., Taylor diagrams and the ratio of the trend between the simulations and observations) shows that only five of these 20 models (i.e., CESM1-CAM5, CMCC-CM, FGOALS-g2, IPSL-CM5B-LR, and NorESM1-M) can reproduce with useful accuracy the spatial pattern and long-term trend of the CTM. We find that these five models generally simulate the main ocean dynamical process associated with the CTM. That is, these models adequately capture the long-term cooling trend in the vertical advection of the anomalous temperature by the mean upwelling. We conclude that the performance of these CMIP5 models, with respect to simulations of the long-term cooling trend associated with the vertical advection, and the related long-term decreasing trend of the vertical gradient of the oceanic temperature anomaly, can play an important role in successful reproduction of the CTM.
In recent years, El Niño-Southern Oscillation (ENSO) diversity, representing differences in the sea surface temperature anomalies (SSTA) of ENSO events, has been a topic of great interest (e.g., Karnauskas, 2013; Ren HL and Jin FF, 2013; Ren HL et al., 2013, 2016a; Zhang WJ et al., 2013, 2014; Zheng F et al., 2014, 2016; Capotondi et al., 2015; Zheng F and Yu JY, 2017). Many studies have pointed out that the tropical Pacific background state makes an important contribution to ENSO diversity under global warming (Ashok et al., 2007; Yeh et al., 2009; Collins et al., 2010; Duan WS et al., 2014; Li Y et al., 2017; Lemmon and Karnauskas, 2018). Numerous studies have reported that the tropical Pacific background state is similar to a La Niña-like pattern (i.e., greater warming in the west than the east) and that it plays an important role in ENSO diversity (Duan WS et al., 2014; Li Y et al., 2017; Jiang N and Zhu CW, 2018; Lemmon and Karnauskas, 2018).
Although there is disagreement about the relationship between the long-term trend of tropical Pacific and global warming (Collins et al., 2010), the La Niña-like pattern has been confirmed by many observations. For example, Li Y et al. (2017) reported that the subsurface temperature of the eastern equatorial Pacific is cooler than that of the western equatorial Pacific; Karnauskas et al. (2009) and Coats and Karnauskas (2017) pointed out that many datasets indicate a strengthening of the equatorial Pacific zonal SST gradient. Meanwhile, the equatorial undercurrent and subtropical cells associated with the La Niña-like pattern have been demonstrated to be accelerating (Drenkard and Karnauskas, 2014; Yang CX et al., 2014; Coats and Karnauskas, 2018). Some atmospheric evidence also supports the La Niña-like pattern, such as the strengthening of Walker circulation and trades (L’Heureux et al., 2013; Li Y et al., 2019). These results indicate that the La Niña-like condition plays a dominant role in the tropical Pacific under global warming (Cane et al., 1997).
Many studies have demonstrated that the second EOF mode of the tropical Pacific can present a La Niña-like pattern under global warming (Zhang WJ et al., 2010; Li JP et al., 2013; Duan WS et al., 2014; Li Y et al., 2015, 2017, 2019; Lemmon and Karnauskas, 2019). As shown in Figure 1, the second EOF mode represents an out-of-phase relationship in SSTA variability between the Pacific cold tongue region and the rest of the tropical Pacific. Its spatial pattern is consistent with the La Niña-like pattern (Fig. 5 in Solomon and Newman, 2012), and its normalized principal components (NPC2) show a strong long-term trend. This means that there is a cooling cold tongue region under global warming. In addition, it can be seen from Figure 1 that ENSO is identified by the first EOF mode, with strong interannual variability (Deser et al., 2009; Compo and Sardeshmukh, 2010; Zhang WJ et al., 2010).
Following previous studies (Zhang WJ et al., 2010; Li JP et al., 2013; Li Y et al., 2015, 2017, 2019; Jiang N and Zhu CW, 2018), the second EOF mode is referred to as the cold tongue mode (CTM). Li Y et al. (2015) emphasized that “the long-term trend of the CTM is dominated by the vertical advection of the anomalous temperature by the mean upwelling (
It is noted that the CTM has been identified not only in observations, but also in climate models. Zhang WJ et al. (2010) pointed out that the CTM is accurately simulated in the 20th century run of some Phase 3 models in the Coupled Model Intercomparison Project (CMIP3). The current Phase 5 CMIP (CMIP5) models have higher spatial resolution relative to those in CMIP3 (Taylor et al., 2012). In addition, the CMIP5 models have improved the simulation of climate variability; some are able to capture important climate phenomena in the Pacific, for example ENSO (Kim and Yu JY, 2012; Kug et al., 2012; Zhang WJ and Jin FF, 2012; Bellenger et al., 2014; Ren HL et al., 2016b; Lin RP et al., 2018), Pacific Decadal Oscillation (PDO) (Newman et al., 2016), and the La Niña-like pattern (Funk and Hoell, 2015). However, no research has been devoted to investigating the CTM in the CMIP5 simulations. Consequently, in this study we aim to address two main issues: How well do the CMIP5 models reproduce the CTM pattern and its long-term trend? If some models reproduce the CTM well, how well do these models reproduce the main ocean dynamical process associated with the CTM?
The remainder of this paper is organized as follow. Section 2 introduces the data and methods used in this study. An analysis of the performance of the models in reproducing the historical CTM is presented in Section 3. The performance of the models in reproducing the main ocean dynamical process associated with the CTM is discussed in Section 4. Finally, discussion and conclusions are presented in Section 5.
We used monthly SST from UK Met Office’s Hadley Centre Sea Ice and SST version 1 (HadISST1) dataset (Rayner et al., 2003). We analyzed simulations from the 20 models that participated in CMIP5. The model output and information, including their developing centers and horizontal resolutions, are listed in Table 1. To evaluate the performance of the models in reproducing the CTM, we used monthly output over the period 1870–2004 from historical simulations (r1i1p1), which were forced by observed atmospheric composition changes from natural and anthropogenic sources (Taylor et al., 2012). As the ocean component of most models used a tripolar global grid, the modeled outputs of ocean variables (SST, ocean subsurface temperature, and upwelling) were interpolated onto a common rectangular grid of 1.0° × 1.0° prior to further calculations. Note that the mean seasonal cycle of all datasets from 1961 to 1990 was removed and that our results are not sensitive to the choices of mean seasonal cycle.
Name | Model center/Country | Resolution (Latitude (°)×Longitude (°)) | |
Atmosphere | Ocean | ||
ACCESS1-3 | Commonwealth Scientific and Industrial Research Organization (CSIRO) and Bureau of Meteorology (BOM)/Australia | 145 × 192 | 300 × 360 |
BCC-CSM1-1-M | Beijing Climate Center (BCC), China Meteorological Administration (CMA)/China | 160 × 320 | 232 × 360 |
BNU-ESM | College of Global Change and Earth System Science (GCESS), Beijing Normal University (BNU)/China | 64 × 128 | 200 × 360 |
CanESM2 | Canadian Centre for Climate Modeling and Analysis (CCCMA)/Canada | 64 × 128 | 192 × 256 |
CCSM4 | National Center for Atmospheric Research (NCAR)/United States | 192 × 288 | 384 × 320 |
CESM1-BGC CESM1-CAM5 | National Science Foundation (NSF), Department of Energy (DOE), NCAR/United States | 192 × 288 | 384 × 320 |
CMCC-CM | Centro Euro-Mediterraneo per 1 Cambiamenti Climatici (CMCC)/Italy | 240 × 480 | 149 × 182 |
FGOALS-g2 | State Key Laboratory of Numerical Modeling for Atmospheric Sciences and Geophysical Fluid Dynamics (LASG), Institute of Atmospheric Physics (IAP), Chinese Academy of Sciences (CAS)/China | 60 × 128 | 196 × 360 |
GFDL-ESM2G | National Oceanic and Atmospheric Administration (NOAA) Geophysical Fluid Dynamics Laboratory (GFDL)/United States | 90 × 144 | 210 × 360 |
GISS-E2-H GISS-E2-H-CC | National Aeronautics and Space Administration (NASA) Goddard Institute for Space Studies (GISS)/United States | 90 × 144 | 90 × 144 |
HadGEM2-CC HadGEM2-ES | Met Office Hadley Centre (MOHC; additional HadGEM2-ES realizations contributed by Instituto Nacional de Pesquisas Espaciais)/United Kingdom | 145 × 192 | 216 × 360 |
INM-CM4 | Institute for Numerical Mathematics (INM)/Russia | 120 × 180 | 340 × 360 |
IPSL-CM5A-LR IPSL-CM5B-LR | Institut Pierre-Simon Laplace (IPSL)/France | 96 × 96 | 149 × 182 |
MPI-ESM-P | Max Planck Institute for Meteorology (MPI-M)/Germany | 96 × 192 | 220 × 256 |
NorESM1-M NorESM1-ME | Norwegian Climate Centre (NCC)/Norway | 96 × 144 | 384 × 320 |
To avoid the impact of outliers on trend estimation, trends were calculated as the Theil–Sen median slope (Sen, 1968; Theil, 1992) and tested for statistical significance using the Mann–Kendall test. Note that the Theil–Sen trend estimation method is measured by the median of slopes between all pairs and that it was determined using the following equation:
trend=Median(xi−xji−j),∀j<i,1⩽ |
where trend is the Theil–Sen trend,
In addition, we used the partial correlation coefficient (PR) to detect the relationship between each pair of variables after excluding the effect of the third variable. Moreover, linear regression was used to remove the specific signal. Note that the PR and removal related to linear regression can exclude only linear components but not nonlinear interactions (Zheng F et al., 2015).
As mentioned above, the first EOF mode over the tropical Pacific represents the ENSO mode (Deser et al., 2009; Compo and Sardeshmukh, 2010; Zhang WJ et al., 2010), and the second EOF mode depicts the CTM (Zhang WJ et al., 2010; Li JP et al., 2013; Duan WS et al., 2014; Li Y et al., 2015, 2017). ENSO represents the interannual variability of ocean dynamical processes (Collins et al., 2010), while the CTM is dominated by the long-term change of ocean dynamical processes (Zhang WJ et al., 2010; Li JP et al., 2013; Li Y et al., 2015, 2017). It is suggested that the simulation of ENSO may play an important role in reproducing the CTM (Zhang et al., 2010). Therefore, we first assess the ENSO mode before examining the performance of CMIP5 models in reproducing the CTM. This approach is similar to that of Zhang WJ et al. (2010).
Figure 2 shows the first EOF modes of SSTA over the tropical Pacific and their corresponding NPC1 time series that are simulated by each of the 20 CMIP5 models. Qualitatively, it can be seen from Figure 2 that the spatial pattern of the ENSO mode is not captured by six of the models (i.e., BCC-CSM1-1-M, CanESM2, CCSM4, GISS-E2-H, IPSL-CM5A-LR, and MPI-ESM-P). This is because these six models show a warming SSTA across almost the whole tropical Pacific, and their time series exhibit no significant interannual variability and are dominated by a rising trend in SSTA (Figure 2). In particular, for four of the models (i.e., CanESM2, GISS-E2-H, IPSL-CM5A-LR, and MPI-ESM-P), their time series almost correspond to a strong warming trend (Figure 2). According to previous research (Zhang WJ et al., 2010), the most plausible cause of this result is that the interannual signal of the ENSO mode is hidden by global warming.
To quantitatively examine the accordance between the CMIP5 models and the observations, a pair of Taylor diagrams (Taylor, 2001) is used to compare the performance of the models based on the spatial pattern of the ENSO mode. For the six models named above, the corresponding ratios of the standard deviation of the modeled and observed ENSO patterns are less than 0.75 (Figure 3a). Using root-mean-square (RMS) differences between the simulations and the observations, shown as the distance between the model point and the “REF” point in the Taylor diagram, the distances obtained for these six models are approximately greater than 0.5 (Figure 3a). This supports the conclusion that these six models perform less well in reproducing the observed ENSO pattern.
Aside from the above six models, three models (i.e., BNU-ESM, GFDL-ESM2G, and GISS-E2-H-CC) tend towards strong interannual variability in the western equatorial Pacific (Figure 2). Correspondingly, the RMS differences of these six models are approximately larger than 0.5 (Figure 3a). In particular, BNU-ESM generates an RMS difference of more than 1.0 (Figure 3a). Meanwhile, another two models (i.e., HadGEM2-ES and INMCM4) underestimate the amplitude of the ENSO mode (Figure 2). This can be seen from the ratios of the standard deviation of the modeled and observed ENSO patterns, whose values are just about 0.5 (Figure 3a).
Another important aspect of the behavior of ENSO is its spectral characteristics; i.e., ENSO shows an interannual variability. Here, we use the continuous power spectrum to examine periodicity of ENSO mode in the observation and performance of the CMIP5 models in reproducing the spectral structure of the ENSO mode. The NPC1 of ENSO mode in the observation shows a significant periodicity of 2–4 years. In summary, most of the models capture the observed spectral peak at 2–4 years (Figure 4), except for the six models mentioned above (i.e., BCC-CSM1-1-M, CanESM2, CCSM4, GISS-E2-H, IPSL-CM5A-LR, and MPI-ESM-P), which all have spectral peaks at very low frequency. This corresponds to the strong long-term trend of the time series in these six models. It further suggests that the interannual signal of these six models is masked by global warming.
Overall, 11 models (i.e., BCC-CSM1-1-M, BNU-ESM, CanESM2, CCSM4, GFDL-ESM2G, GISS-E2-H, GISS-E2-H-CC, HadGEM2-ES, INMCM4, IPSL-CM5A-LR, and MPI-ESM-P) are not able accurately to capture the spatial or temporal characteristic of the ENSO mode (Figures 3a and 4). The remaining nine models (i.e., ACCESS1-3, CESM1-BGC, CESM1-CAM5, CMCC-CM, FGOALS-g2, HadGEM2-CC, IPSL-CM5B-LR, NorESM1-M, and NorESM1-ME) perform well in reproducing both the observed spatial pattern and the interannual variations of the ENSO mode. It can be seen from Figure 3a that the distances between the points associated with these nine models and the “REF” point are generally smaller than those of the other 11 models. Also, these nine models exhibit peaks over interannual scales of about 2–4 years.
As discussed above, we first analyze the performance of the CMIP5 models in reproducing the ENSO mode. Next, we consider how well the CMIP5 models reproduce the spatial and temporal characteristics of the CTM. We know that in nature the CTM represents an out-of-phase relationship of SSTA variability between the Pacific cold tongue region and elsewhere in the tropical Pacific, and that the time series of the CTM exhibits a strong long-term trend (Figure 1). Thus, in the following, we use the Taylor diagram to evaluate the spatial pattern of the modeled CTM, and use the ratio of trends to make a comparison between the modeled and observed time series of the CTM.
Figure 5 shows the second EOF modes in SSTA over the tropical Pacific and their NPC2 time series. Most of the models are able to simulate the cooling SSTA in the eastern equatorial Pacific associated with the positive CTM well (Figure 5). However, compared with Taylor diagram analysis of the ENSO mode (Figure 3a), the spatial correlation coefficients in Figure 3b are overall lower (Figure 3b), and the distances between the model points and the “REF” point (Figure 3b) are typically greater. This implies that the CMIP5 models generally provide a better simulation of the ENSO mode than of the CTM. Interestingly, eight of the models (i.e., BCC-CSM1-1-M, BNU-ESM, CCSM4, GFDL-ESM2G, GISS-E2-H, HadGEM2-ES, INMCM4, and MPI-ESM-P) mentioned above, which are not able accurately to simulate the spatial or temporal characteristic of the ENSO mode, are also unable to capture realistically the spatial features of the CTM. We note that these eight models have weaker spatial correlation coefficients, smaller ratios of the standard deviation, or are further from the “REF” point in comparison with the other models (Figure 3b).
Considering the simulation of the temporal features of the CTM, we use the ratio of the simulated and observed trends of NPC2 to assess the performance of the CMIP5 models in reproducing the long-term trends in the CTM. Quantitatively, there are eight models (i.e., ACCESS1-3, CanESM2, GFDL-ESM2G, GISS-E2-H, HadGEM2-CC, IPSL-CM5A-LR, MPI-ESM-P, and NorESM1-ME) whose ratios of trends are relatively weak compared with the other models (Figure 6). For four of these models in particular (i.e., ACCESS1-3, GISS-E2-H, HadGEM2-CC, and IPSL-CM5A-LR), their NPC2 time series are almost dominated by the interannual signal (Figure 5). This suggests that the CTM simulated by these eight models tends to have a stronger interannual signal than that seen in the observations. In addition, of these eight models mentioned above, we also note that five (i.e., CanESM2, GFDL-ESM2G, GISS-E2-H, IPSL-CM5A-LR, and MPI-ESM-P) do not accurately reproduce the spatial or temporal characteristics of the ENSO mode. Except for these eight models, the rest of the models yield ratios of trends between the simulated and observed NPC2 time series that are generally located within a band about 0.2 wide centered on 1.0 (Figure 6). This indicates that the 10 models in the pink rectangle (Figure 6) are able to capture the observed long-term trend of the CTM. In addition, we note that in the CESM1-BGC and GISS-E2-H-CC models the long term trends are relatively strong compared with the observations (Figures 5 and 6).
Overall, 15 models (i.e., ACCESS1-3, BCC-CSM1-1-M, BNU-ESM, CanESM2, CCSM4, CESM1-BGC, GFDL-ESM2G, GISS-E2-H, GISS-E2-H-CC, HadGEM2-CC, HadGEM2-ES, INMCM4, IPSL-CM5A-LR, MPI-ESM-P, and NorESM1-ME) are unable to capture satisfactorily the spatial or temporal characteristic of the CTM (Figures 3b and 6). Compared with the capability, mentioned above, of the CMIP5 models in capturing the ENSO mode, our analysis suggests that if a model is not able to reproduce ENSO, then it is likely also to be unable to simulate the CTM usefully. This finding supports the suggestion of Zhang WJ et al. (2010), who emphasized that the reproduction of the CTM depends on the simulation ability of ENSO in CMIP3 models. Finally, we find that five models (i.e., CESM1-CAM5, CMCC-CM, FGOALS-g2, IPSL-CM5B-LR, and NorESM1-M) yield superior results, reproducing realistic ENSO features and observed spatial characteristic and long-term trends associated with the CTM. Why the CTM is better simulated by these five models will be discussed in the following section.
Li Y et al. (2015, 2017) used the heat budget equation to show that “the cooling associated with the vertical advection of the anomalous temperature by the mean upwelling (
The bottom panels in Figure 7 show a normalized time series of the
Raw | reENSO | ||||
- \bar w{{\partial T'} / {\partial z}} | - {{\partial T'} / {\partial z}} | - \bar w{{\partial T'} / {\partial z}} | - {{\partial T'} / {\partial z}} | ||
CESM1-CAM5 | 0.14 | 0.13 | –0.005 | –0.02 | |
CMCC-CM | –0.40 | –0.52 | –0.46 | –0.55 | |
FGOALS-g2 | –0.25 | –0.30 | –0.27 | –0.43 | |
IPSL-CM5B-LR | –0.20 | –0.16 | –0.23 | –0.36 | |
NorESM1-M | –0.19 | –0.21 | –0.30 | –0.34 | |
aBold values indicate significant at the 95% confidence level (nonparametric Mann–Kendall test). |
Despite there being a statistically insignificant trend of the
The analysis above motivated us to examine further why these five models are able to capture the main ocean dynamical process associated with the CTM. Li Y et al. (2015, 2017) emphasized that “the cooling term
Figure 8 shows, for the five models (i.e., CESM1-CAM5, CMCC-CM, FGOALS-g2, IPSL-CM5B-LR, and NorESM1-M), their normalized time series of the
To further clarify the decreasing
We also analyze the long-term trends of the subsurface temperature of the eastern equatorial Pacific in the five models (Figure 10). The raw trends above thermocline in these five models are generally warming (Figure 10). However, the raw trends of sea temperature in four of the models (i.e., CMCC-CM, FGOALS-g2, IPSL-CM5B-LR, and NorESM1-M in Figure 10) are that warming decreases with increased depth. This pattern induces the decreasing trend of the
This study used historical simulations generated by 20 CMIP5 models to test their ability to simulate the CTM. Using Taylor diagrams, the power spectra, and the ratio of the trend in normalized principal components (NPC2) between the model simulations and the observations, we evaluated the spatial and temporal characteristics of the ENSO mode and CTM in these 20 models. In general, we find that models unable accurately to simulate the ENSO feature in the EOF1 mode of the tropical Pacific are also unable to reproduce usefully the observed spatial pattern and long-term trend associated with the CTM that appears in EOF2. Note that eleven of the models (i.e., BCC-CSM1-1-M, BNU-ESM, CanESM2, CCSM4, GFDL-ESM2G, GISS-E2-H, GISS-E2-H-CC, HadGEM2-ES, INMCM4, IPSL-CM5A-LR, and MPI-ESM-P) could not accurately simulate the spatial characteristic of ENSO and also could not reproduce the CTM well. Of the rest of the models, some are able to reproduce the ENSO features, but could not capture effectively the spatial characteristics or the long-term trend of the CTM. For instance, the models ACCESS1-3, HadGEM2-CC, and NorESM1-ME are unable to reproduce the spatial pattern of the CTM (Figure 3b), and the long-term trend of the CTM simulated by CESM1-BGC is too strong, compared with the observations (Figure 6). Finally, five models (i.e., CESM1-CAM5, CMCC-CM, FGOALS-g2, IPSL-CM5B-LR, and NorESM1-M) are capable of capturing with reasonable accuracy the spatial pattern and long-term trend of the CTM. Note that other models are unable to simulate the CTM, which may be related to tropical biases in these CMIP5 models (Kug et al., 2012; Bellenger et al., 2014). In addition, based on the main ocean dynamical process associated with the CTM, the diversity among models in representing the mean upwelling of the eastern equatorial Pacific (Li G and Xie SP, 2012; Li Y et al., 2015) may induce the inter-model difference in the CTM (Figure 5).
Regarding the performance of CMIP5 models in reproducing the main ocean dynamical process associated with the CTM, we find that five models (i.e., CESM1-CAM5, CMCC-CM, FGOALS-g2, IPSL-CM5B-LR, and NorESM1-M) generally simulate the main ocean dynamical process associated with the CTM. In four of these five models (i.e., not in CESM1-CAM5; Table 2), the time series of the
The cooling trend of the
This study has briefly assessed the representation of the observed CTM in the 20 CMIP5 models, and provides a new insight into the simulation of the dynamics of the tropical Pacific. However, the following questions remain. Why are some CMIP5 models unable to reasonably reproduce the CTM? How well do these models simulate the main ocean dynamical process associated with the CTM? Furthermore, although the CMIP5 models are demonstrably improved compared to the CMIP3 models, has there been corresponding improvement in their simulation of the CTM? If so, what is the cause of the improvement(s)? We will address these important questions in future studies.
This work was supported jointly by the National Key Research and Development Program on Monitoring, Early Warning and Prevention of Major Natural Disaster (2018YFC1506006), the National Natural Science Foundation of China Project (41805054, 41875108, 41775072, 41705065), and the SOA International Cooperation Program on Global Change and Air–Sea Interactions (GASI-IPOVAI-03). We acknowledge the modeling groups listed in Table 1 for providing the model output for analysis, the WCRP’s Working Group on Coupled Modeling (WGCM) for organizing the CMIP, and the Earth System Grid Federation (ESGF) for supporting the model data archive (http://cmip-pcmdi.llnl.gov/cmip5). The HadISST1 dataset was obtained from the UK Met Office Hadley Centre and can be downloaded from http://www.metoffice.gov.uk/hadobs/hadisst/data/download.html.
Ashok, K., Behera, S. K., Rao, S. A., Weng, H. Y., and Yamagata, T. (2007). El Niño Modoki and its possible teleconnection. J. Geophys. Res. Oceans, 112(C11), C11007. https://doi.org/10.1029/2006JC003798
|
Bellenger, H., Guilyardi, E., Leloup, J., Lengaigne, M., and Vialard, J. (2014). ENSO representation in climate models: from CMIP3 to CMIP5. Climate Dyn., 42(7-8), 1999–2018. https://doi.org/10.1007/s00382-013-1783-z
|
Cane, M. A., Clement, A. C., Kaplan, A., Kushnir, Y., Pozdnyakov, D., Seager, R., Zebiak, S. E., and Murtugudde, R. (1997). Twentieth-century sea surface temperature trends. Science, 275(5302), 957–960. https://doi.org/10.1126/science.275.5302.957
|
Capotondi, A., Wittenberg, A. T., Newman, M., Di Lorenzo, E., Yu, J. Y., Braconnot, P., Cole, J., Dewitte, B., Giese, B., … Yeh, S. W. (2015). Understanding ENSO diversity. Bull. Am. Meteor. Soc., 96(6), 921–938. https://doi.org/10.1175/BAMS-D-13-00117.1
|
Coats, S., and Karnauskas, K. B. (2017). Are simulated and observed twentieth century tropical Pacific sea surface temperature trends significant relative to internal variability?. Geophys. Res. Lett., 44(19), 9928–9937. https://doi.org/10.1002/2017GL074622
|
Coats, S., and Karnauskas, K. B. (2018). A role for the Equatorial Undercurrent in the ocean dynamical thermostat. J. Climate, 31(16), 6245–6261. https://doi.org/10.1175/JCLI-D-17-0513.1
|
Collins, M., An, S. I., Cai, W. J., Ganachaud, A., Guilyardi, E., Jin, F. F., Jochum, M., Lengaigne, M., Power, S., … Wittenberg, A. (2010). The impact of global warming on the tropical Pacific ocean and El Niño. Nat. Geosci., 3(6), 391–397. https://doi.org/10.1038/ngeo868
|
Compo, G. P., and Sardeshmukh, P. D. (2010). Removing ENSO-Related Variations from the Climate Record. J. Climate, 23(8), 1957–1978. https://doi.org/10.1175/2009JCLI2735.1
|
Deser, C., Alexander, M. A., Xie, S. P., and Phillips, A. S. (2009). Sea surface temperature variability: Patterns and mechanisms. Annu. Rev. Mar. Sci., 2, 115–143. https://doi.org/10.1146/annurev-marine-120408-151453
|
DiNezio, P. N., Clement, A. C., Vecchi, G. A., Soden, B. J., Kirtman, B. P., and Lee, S. K. (2009). Climate response of the equatorial Pacific to global warming. J. Climate, 22(18), 4873–4892. https://doi.org/10.1175/2009JCLI2982.1
|
Drenkard, E. J., and Karnauskas, K. B. (2014). Strengthening of the Pacific equatorial undercurrent in the SODA reanalysis: mechanisms, ocean dynamics, and implications. J. Climate, 27(6), 2405–2416. https://doi.org/10.1175/jcli-d-13-00359.1
|
Duan, W. S., Tian, B., and Xu, H. (2014). Simulations of two types of El Niño events by an optimal forcing vector approach. Climate Dyn., 43(5-6), 1677–1692. https://doi.org/10.1007/s00382-013-1993-4
|
Funk, C. C., and Hoell, A. (2015). The leading mode of observed and CMIP5 ENSO-residual sea surface temperatures and associated changes in Indo-Pacific climate. J. Climate, 28(11), 4309–4329. https://doi.org/10.1175/JCLI-D-14-00334.1
|
Jiang, N., and Zhu, C. W. (2018). Asymmetric changes of ENSO diversity modulated by the cold tongue mode under recent global warming. Geophys. Res. Lett., 45(22), 12506–512513. https://doi.org/10.1029/2018GL079494
|
Karnauskas, K. B., Seager R., Kaplan A., Kushnir Y., and Cane M. A. (2009). Observed strengthening of the zonal sea surface temperature gradient across the equatorial Pacific Ocean. J. Climate, 22(16), 4316–4321. https://doi.org/10.1175/2009JCLI2936.1
|
Karnauskas, K. B. (2013). Can we distinguish canonical El Niño from Modoki?. Geophys. Res. Lett., 40(19), 5246–5251. https://doi.org/10.1002/grl.51007
|
Kim, S. T., and Yu, J. Y. (2012). The two types of ENSO in CMIP5 models. Geophys. Res. Lett., 39(11), L11704. https://doi.org/10.1029/2012GL052006
|
Kug, J. S., Ham, Y. G., Lee, J. Y., and Jin, F. F. (2012). Improved simulation of two types of El Niño in CMIP5 models. Environ. Res. Lett., 7(3), 034002. https://doi.org/10.1088/1748-9326/7/3/034002
|
Lemmon, D. E., and Karnauskas, K. B. (2019). A metric for quantifying El Niño pattern diversity with implications for ENSO-mean state interaction. Climate Dyn., 52(12), 7511–7523. https://doi.org/10.1007/s00382-018-4194-3
|
L'Heureux, M. L., Lee, S., and Lyon, B. (2013). Recent multidecadal strengthening of the Walker circulation across the tropical Pacific. Nat. Climate Change, 3(6), 571–576. https://doi.org/10.1038/nclimate1840
|
Li, G., and Xie, S. P. (2012). Origins of tropical-wide SST biases in CMIP multi-model ensembles. Geophys. Res. Lett., 39(22), L22703. https://doi.org/10.1029/2012GL053777
|
Li, J. P., Ren, R. C., Qi, Y. Q., Wang, F. M., Lu, R. Y., Zhang, P. Q., Jiang, Z. H., Duan, W. S., Yu, F., and Yang, Y. Z. (2013). Progress in air-land-sea interactions in Asia and their role in global and Asian climate change. Chin. J. Atmos. Sci. (in Chinese)
|
Li, Y., Li, J. P., Zhang, W. J., Zhao, X., Xie, F., and Zheng, F. (2015). Ocean dynamical processes associated with the tropical Pacific cold tongue mode. J. Geophys. Res. Oceans, 120(9), 6419–6435. https://doi.org/10.1002/2015JC010814
|
Li, Y., Li, J. P., Zhang, W. J., Chen, Q. L., Feng, J., Zheng, F., Wang, W., and Zhou, X. (2017). Impacts of the tropical Pacific cold tongue mode on ENSO diversity under global warming. J. Geophys. Res. Oceans, 122(11), 8524–8542. https://doi.org/10.1002/2017JC013052
|
Li, Y., Chen, Q. L., Liu, X. R., Li, J. P., Xing, N., Xie, F., Feng, J., Zhou, X., Cai, H. K., and Wang, Z. L. (2019). Long-term trend of the tropical Pacific trade winds under global warming and its causes. J. Geophys. Res. Oceans, 124(4), 2626–2640. https://doi.org/10.1029/2018JC014603
|
Lin, R. P., Zheng, F., and Dong, X. (2018). ENSO frequency asymmetry and the Pacific Decadal Oscillation in observations and 19 CMIP5 models. Adv. Atmos. Sci., 35(5), 495–506. https://doi.org/10.1007/s00376-017-7133-z
|
Newman, M., Alexander, M. A., Ault, T. R., Cobb, K. M., Deser, C., Di Lorenzo, E., Mantua, N. J., Miller, A. J., Minobe, S., … Smith, C. A. (2016). The Pacific decadal oscillation, revisited. J. Climate, 29(12), 4399–4427. https://doi.org/10.1175/JCLI-D-15-0508.1
|
Rayner, N. A., Parker, D. E., Horton, E. B., Folland, C. K., Alexander, L. V., Rowell, D. P., Kent, E. C., and Kaplan, A. (2003). Global analyses of sea surface temperature, sea ice, and night marine air temperature since the late nineteenth century. J. Geophys. Res. Atmos., 108(D14), 4407. https://doi.org/10.1029/2002JD002670
|
Ren, H. L., and Jin, F. F. (2013). Recharge oscillator mechanisms in two types of ENSO. J. Climate, 26(17), 6506–6523. https://doi.org/10.1175/JCLI-D-12-00601.1
|
Ren, H. L., Jin, F. F., Stuecker, M. F., and Xie, R. H. (2013). ENSO regime change since the late 1970s as manifested by two types of ENSO. J. Meteor. Soc. Japan. Ser. II, 91(6), 835–842. https://doi.org/10.2151/jmsj.2013-608
|
Ren, H. L., Jin, F. F., Tian, B., and Scaife, A. A. (2016a). Distinct persistence barriers in two types of ENSO. Geophys. Res. Lett., 43(20), 10973–10979. https://doi.org/10.1002/2016GL071015
|
Ren, H. L., Zuo, J. Q., Jin, F.-F., and Stuecker, M. F. (2016b). ENSO and annual cycle interaction: the combination mode representation in CMIP5 models. Climate Dyn., 46(11-12), 3753–3765. https://doi.org/10.1007/s00382-015-2802-z
|
Sen, P. K. (1968). Estimates of the regression coefficient based on Kendall's tau. J. Am. Stat. Assoc., 63(324), 1379–1389. https://doi.org/10.1080/01621459.1968.10480934
|
Solomon, A., and Newman, M. (2012). Reconciling disparate twentieth-century Indo-Pacific ocean temperature trends in the instrumental record. Nat. Climate Change, 2(9), 691–699. https://doi.org/10.1038/nclimate1591
|
Taylor, K. E. (2001). Summarizing multiple aspects of model performance in a single diagram. J. Geophys. Res. Atmos., 106(D7), 7183–7192. https://doi.org/10.1029/2000JD900719
|
Taylor, K. E., Stouffer, R. J., and Meehl, G. A. (2012). An overview of CMIP5 and the experiment design. Bull. Am. Meteor. Soc., 93(4), 485–498. https://doi.org/10.1175/bams-d-11-00094.1
|
Theil, H. (1992). A rank-invariant method of linear and polynomial regression analysis. In B. Raj, et al. (Eds.), Henri Theil’s Contributions to Economics and Econometrics: Econometric Theory and Methodology (pp. 345-381). Dordrecht: Springer. https://doi.org/10.1007/978-94-011-2546-8_20
|
Yang, C. X., Giese, B. S., and Wu, L. X. (2014). Ocean dynamics and tropical Pacific climate change in ocean reanalyses and coupled climate models. J. Geophys. Res. Oceans, 119(10), 7066–7077. https://doi.org/10.1002/2014JC009979
|
Yeh, S. W., Kug, J. S., Dewitte, B., Kwon, M. H., Kirtman, B. P., and Jin, F. F. (2009). El Niño in a changing climate. Nature, 461(7263), 511–514. https://doi.org/10.1038/nature08316
|
Zhang, W. J., Li, J. P., and Zhao, X. (2010). Sea surface temperature cooling mode in the Pacific cold tongue. J. Geophys. Res. Oceans, 115(C12), C12042. https://doi.org/10.1029/2010jc006501
|
Zhang, W. J., and Jin, F. F. (2012). Improvements in the CMIP5 simulations of ENSO-SSTA meridional width. Geophys. Res. Lett., 39(23), L23704. https://doi.org/10.1029/2012GL053588
|
Zhang, W. J., Jin, F. F., Zhao, J. X., Qi, L., and Ren, H. L. (2013). The possible influence of a nonconventional El Niño on the severe autumn drought of 2009 in southwest China. J. Climate, 26(21), 8392–8405. https://doi.org/10.1175/JCLI-D-12-00851.1
|
Zhang, W. J., Jin, F. F., and Turner, A. (2014). Increasing autumn drought over southern China associated with ENSO regime shift. Geophys. Res. Lett., 41(11), 4020–4026. https://doi.org/10.1002/2014GL060130
|
Zheng, F., Fang, X. H., Yu, J. Y., and Zhu, J. (2014). Asymmetry of the Bjerknes positive feedback between the two types of El Niño. Geophys. Res. Lett., 41(21), 7651–7657. https://doi.org/10.1002/2014GL062125
|
Zheng, F., Li, J. P., Feng, J., Li, Y. J., and Li, Y. (2015). Relative importance of the austral summer and autumn SAM in modulating Southern Hemisphere extratropical autumn SST. J. Climate, 28(20), 8003–8020. https://doi.org/10.1175/JCLI-D-15-0170.1
|
Zheng, F., Fang, X. H., Zhu, J., Yu, J. Y., and Li, X. C. (2016). Modulation of Bjerknes feedback on the decadal variations in ENSO predictability. Geophys. Res. Lett., 43(24), 12560–12568. https://doi.org/10.1002/2016GL071636
|
Zheng, F., and Yu, J. Y. (2017). Contrasting the skills and biases of deterministic predictions for the two types of El Niño. Adv. Atmos. Sci., 34(12), 1395–1403. https://doi.org/10.1007/s00376-017-6324-y
|
Name | Model center/Country | Resolution (Latitude (°)×Longitude (°)) | |
Atmosphere | Ocean | ||
ACCESS1-3 | Commonwealth Scientific and Industrial Research Organization (CSIRO) and Bureau of Meteorology (BOM)/Australia | 145 × 192 | 300 × 360 |
BCC-CSM1-1-M | Beijing Climate Center (BCC), China Meteorological Administration (CMA)/China | 160 × 320 | 232 × 360 |
BNU-ESM | College of Global Change and Earth System Science (GCESS), Beijing Normal University (BNU)/China | 64 × 128 | 200 × 360 |
CanESM2 | Canadian Centre for Climate Modeling and Analysis (CCCMA)/Canada | 64 × 128 | 192 × 256 |
CCSM4 | National Center for Atmospheric Research (NCAR)/United States | 192 × 288 | 384 × 320 |
CESM1-BGC CESM1-CAM5 | National Science Foundation (NSF), Department of Energy (DOE), NCAR/United States | 192 × 288 | 384 × 320 |
CMCC-CM | Centro Euro-Mediterraneo per 1 Cambiamenti Climatici (CMCC)/Italy | 240 × 480 | 149 × 182 |
FGOALS-g2 | State Key Laboratory of Numerical Modeling for Atmospheric Sciences and Geophysical Fluid Dynamics (LASG), Institute of Atmospheric Physics (IAP), Chinese Academy of Sciences (CAS)/China | 60 × 128 | 196 × 360 |
GFDL-ESM2G | National Oceanic and Atmospheric Administration (NOAA) Geophysical Fluid Dynamics Laboratory (GFDL)/United States | 90 × 144 | 210 × 360 |
GISS-E2-H GISS-E2-H-CC | National Aeronautics and Space Administration (NASA) Goddard Institute for Space Studies (GISS)/United States | 90 × 144 | 90 × 144 |
HadGEM2-CC HadGEM2-ES | Met Office Hadley Centre (MOHC; additional HadGEM2-ES realizations contributed by Instituto Nacional de Pesquisas Espaciais)/United Kingdom | 145 × 192 | 216 × 360 |
INM-CM4 | Institute for Numerical Mathematics (INM)/Russia | 120 × 180 | 340 × 360 |
IPSL-CM5A-LR IPSL-CM5B-LR | Institut Pierre-Simon Laplace (IPSL)/France | 96 × 96 | 149 × 182 |
MPI-ESM-P | Max Planck Institute for Meteorology (MPI-M)/Germany | 96 × 192 | 220 × 256 |
NorESM1-M NorESM1-ME | Norwegian Climate Centre (NCC)/Norway | 96 × 144 | 384 × 320 |
Raw | reENSO | ||||
- \bar w{{\partial T'} / {\partial z}} | - {{\partial T'} / {\partial z}} | - \bar w{{\partial T'} / {\partial z}} | - {{\partial T'} / {\partial z}} | ||
CESM1-CAM5 | 0.14 | 0.13 | –0.005 | –0.02 | |
CMCC-CM | –0.40 | –0.52 | –0.46 | –0.55 | |
FGOALS-g2 | –0.25 | –0.30 | –0.27 | –0.43 | |
IPSL-CM5B-LR | –0.20 | –0.16 | –0.23 | –0.36 | |
NorESM1-M | –0.19 | –0.21 | –0.30 | –0.34 | |
aBold values indicate significant at the 95% confidence level (nonparametric Mann–Kendall test). |